What is Product Reliability: Essential Points and Examples

Few concepts are as crucial as product reliability!
This article explores its definition, importance, measurement techniques, and strategies for reliability improvement. It will range from warranty policies to recent AI tools implementations in reliability such as 3D Deep Learning.
What is Product Reliability?
Product reliability means that a product performs its intended function consistently and without failure under specified conditions (known as the operating environment), for a defined period (known as the product lifecycle).
Reliability is a critical aspect of product quality and customer satisfaction, brand reputation. A company is considered reliable when its products have proved reliable; this is an important factor in our daily purchasing decisions.
Nobody would purchase products that perform satisfactorily during the first mile and fail soon after!
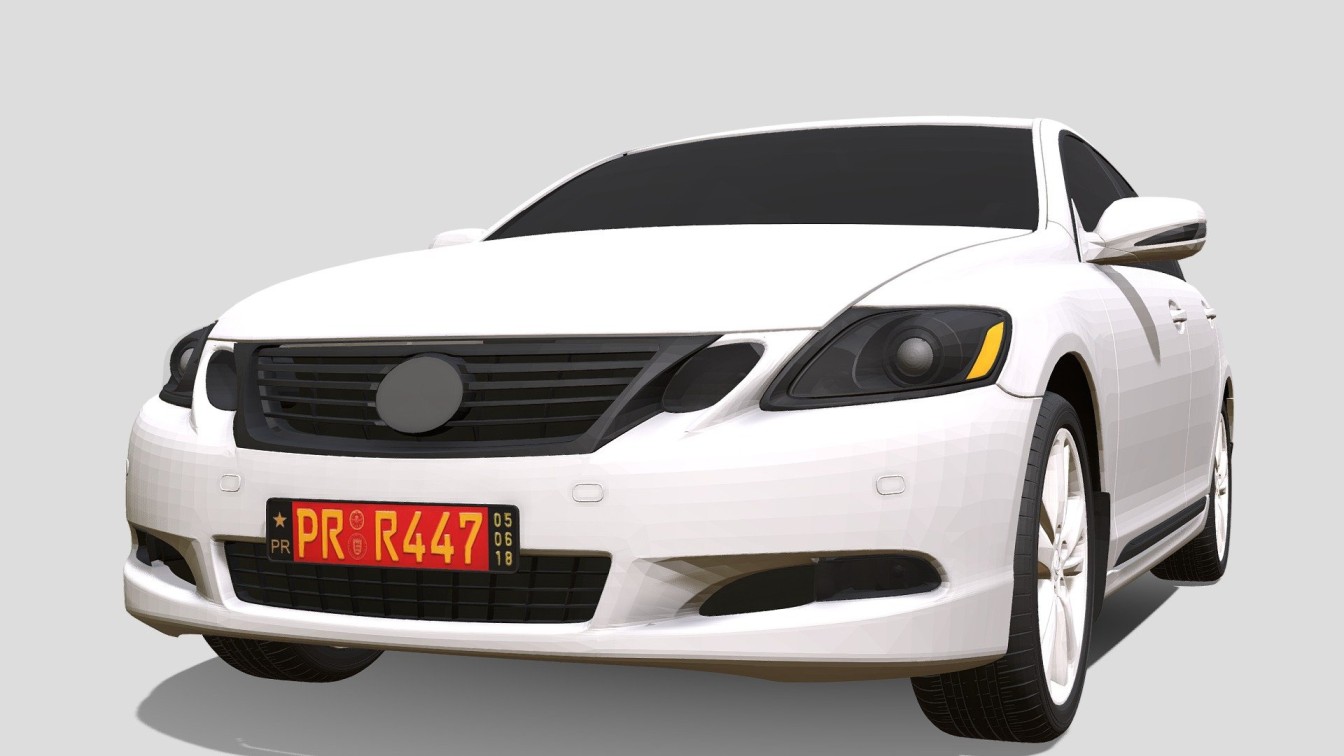
Five Key Components of Product's Reliability
1. Consistency: A reliable product performs its function consistently over time.
2. Durability: A reliable product that withstands wear and tear, maintaining its performance throughout its intended lifespan.
3. Quality: High-quality materials and production processes contribute to overall reliability.
4. Functionality: A reliable product must effectively perform its intended function under various operating conditions.
5. Longevity: A reliable product has a longer useful life, reducing the need for frequent replacements.
Importance of the Reliability of a Product
The significance of a product's reliability and reliability extends far beyond the immediate satisfaction of a customer. It impacts various aspects of a business and its operations.
Customer Satisfaction
The reliability of a product plays a role in customer satisfaction. When customers purchase a product, they expect it to function as intended for a reasonable period. Products that are reliable meet or exceed these expectations.

Customers who experience consistent, reliable performance from a product are more likely to develop a positive association with the brand, becoming loyal customers and potentially brand advocates.
Brand Reputation
In today's interconnected world, brand reputation is more important than ever. Product reliability significantly influences how a brand is perceived in the market. A reputation for reliability can be a powerful marketing tool, attracting new customers and retaining existing ones. Conversely, a reputation for unreliable products can be devastating, leading to lost sales, negative reviews, and long-term damage to the brand.
Consider the following impacts:
- Reliable products enhance brand image and perceived value.
- Positive reputation leads to increased market share and customer base.
- A strong brand reputation can command premium pricing.
- Reliability helps differentiate products in competitive markets.
Cost Efficiency with a Strategic Warranty Policy
A well-crafted warranty policy, underpinned by product reliability, can significantly impact a company's long-term cost efficiency throughout the product life cycle. By focusing on reliability during the product development process and implementing a strategic warranty, companies can avoid costly issues afterwards. This approach protects against unforeseen expenses and builds a reputation for quality, ultimately improving the company's bottom line.
While investing in reliability and comprehensive warranty coverage might seem costly upfront, it often leads to substantial savings and benefits, as detailed below.
- Optimized warranty costs: A strategic warranty, backed by reliable products, can reduce the frequency and severity of claims, leading to lower overall warranty expenses.
- Reduced product returns: products covered by a clear policy tend to result in fewer returns, saving on logistics and replacement costs.
- Lower customer support and service expenses: Products under a well-designed policy naturally require less customer support, reducing associated costs.
- Decreased likelihood of product recalls: Investing in reliability minimizes the risk of widespread issues that could necessitate costly recalls or mass replacements.
- Improved production efficiency: The focus on reliability often leads to better manufacturing processes, resulting in fewer defects and improved overall efficiency.
- Potential for higher profit margins: A strong warranty, supported by product reliability, can justify premium pricing, potentially increasing profit margins.
- Enhanced customer trust: A comprehensive warranty demonstrates confidence in product quality, which can boost customer loyalty and reduce churn-related costs because of better customer experiences.
Regulatory Compliance
Strict regulations and standards in many industries govern product reliability and safety. Ensuring product reliability is not just a quality assurance measure; it is a critical step in meeting or exceeding these industry requirements. When they prioritize reliability, companies can better tackle complex regulatory compliance systems.
This strategy helps avoid potential legal issues and fines arising from substandard products, thereby protecting the company's reputation and its financial stability.
Beyond the immediate benefits, a strong track record in reliability and compliance builds trust with regulatory bodies and the so-called "industry watchdogs". This trust can prove invaluable, potentially easing future interactions with these entities and reinforcing the company's standing in the industry.
Adherence to reliability standards transcends mere legal obligation; it demonstrates a company's commitment to quality and customer safety, inspiring long-term customer loyalty and industry respect.
How is Product Reliability Measured?
Measuring product reliability is a critical aspect of the product development and product management process. It involves various metrics, testing methods, and analytical techniques.
Practical Formulas for Product Managers
• Mean Time Between Failures (MTBF): The average time between system failures during normal operation.
MTBF = Total Operational Time / Number of Failures
• Failure Rate: The frequency with which a product or a component fails, often expressed as failures per unit of time.
Fail Rate = 1 / MTBF
• Reliability Function R(t): The probability that a product will perform its intended function for a specified period under stated conditions.
R(t) = exp(-Fail Rate * t)
The figure shows a simple comparison between two different products all the rest being equal.

• Availability: The proportion of time a product is in a functioning condition = MTBF / (MTBF + Mean Time to Repair)
• Mean Time To Failure (MTTF): Used for non-repairable systems, it’s the mean time expected until the a piece of equipment first fails = Total Operational Time / Number of Units
Data Analysis for Product Management - FMEA and Weibull
The information collected through various methods is crucial for assessing current reliability levels and identifying areas for improvement. This process begins with collecting real-world performance information from products in use, known as Field Data.
Failure Mode and Effects Analysis (FMEA) is employed to identify potential failure and its impacts. Monitoring and controlling production processes to ensure consistency is achieved through Statistical Process Control (SPC). Additionally, Reliability Block Diagrams provide graphical representations of system reliability relationships, while Fault Tree Analysis offers a top-down approach to identifying potential causes of system failures.

The idealized XY plot in the figure illustrates an FMEA risk priority matrix.
X-axis: Represents the Severity of the failure mode (FM) (scale of 1-10)
Y-axis: Represents the Occurrence or likelihood of the FM (scale of 1-10)
Bubble size: Represents the Risk Priority Number (RPN), which is typically calculated as Severity Occurrence Detection, and each bubble represents a specific FM
The general equation for Risk Priority Number (RPN) in FMEA is:
RPN = S O D
Where S = Severity O = Occurrence and D = Detection. The RPN equation in FMEA (FM and Effects Analysis) has significant limitations and has been the subject of much debate and criticism in the quality management field because it's an "equation" based on qualitative considerations.
This visualization allows us quickly identify high-risk failure modes:
- Bubbles in the upper-right quadrant represent high-severity, high-occurrence failures
- Larger bubbles indicate higher RPN values, suggesting priority for corrective actions
Finally, Weibull Analysis is a statistical method used for modeling and analyzing life data, completing the comprehensive approach to information collection and elaboration in product management with a more versatile approach than RPN calculations.
Examples of Product Reliability
Products that are highly reliable can be found across various industries.
The examples below demonstrate how reliability can become a key differentiator and selling point across different industries.
1. Automotive Industry
Toyota vehicles are renowned for being reliable. Many models run for hundreds of thousands of miles with minimal issues.
Tesla's electric powertrains have shown to be exceptionally reliable due to their simplicity compared to traditional combustion engines.
2. Electronics
Apple's iPhone series has consistently scored high in ratings, with low failure rates and long-term performance.
IBM's mainframe computers are designed to be exceptionally reliable, sometimes achieving 99.999% uptime.
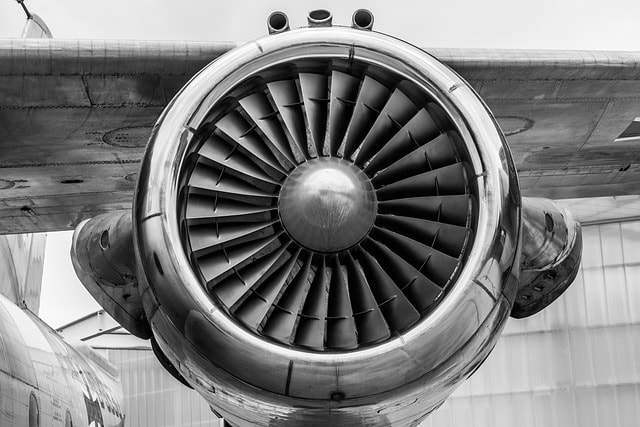
3. Aerospace
Jet engines from manufacturers like Rolls-Royce are engineered for ultra-high reliability, with rigorous testing and maintenance schedules.
Spacecraft equipment undergoes extensive reliability testing to ensure they can withstand the harsh conditions of space.
4. Medical Devices
Pacemakers and implantable cardioverter-defibrillators (ICDs) are designed with extreme reliability in mind, often lasting over a decade without issues. MRI machines are built to operate continuously in hospital settings, with high reliability to minimize downtime and maintenance.
5. Consumer Appliances
Miele high-end washing machines and dishwashers are known for their longevity and reliable performance, often lasting 20 years or more.
Vitamix blenders have lengthy warranties and are built to withstand daily use for years without significant performance degradation.
How to Ensure Product Reliability?
Ensuring product reliability is a multifaceted process that should be integrated throughout the product lifecycle.
Here are key strategies!
Design for Reliability
Incorporating reliability considerations from the earliest stages of product development is crucial. This approach, known as Design for Reliability (DFR), starts with identifying potential failure modes early in the design process. So the idea is that engineers can prevent many problems before they occur, reducing the need for costly redesigns or field fixes by focusing on reliability during the design phase,
With robust design principles, engineers can minimize sensitivity to variations and employ redundancy in critical systems to prevent single points of failure. Selecting materials and components with proven reliability records further ensures product dependability. Additionally, utilizing computer-aided engineering tools for simulation allows for thorough evaluation and optimization. Considering the entire product lifecycle, including manufacturing, transportation, and end-use conditions, helps anticipate and mitigate potential issues.
Quality Control
Maintaining strict quality control throughout manufacturing is essential for ensuring product reliability. Key aspects include:
- Implementing statistical process control to monitor and improve manufacturing consistency.
- Conducting incoming quality checks on raw materials and components.
- Performing in-process inspections at critical stages of production.
- Utilizing automated inspection systems for consistent and objective quality assessments.
- Implementing traceability systems to track components and products through the supply chain.
- Continuously training staff on quality control procedures and the importance of reliability.
Quality control not only catches defects before products reach customers but also helps identify and address systemic issues in the production process.
Maintenance and Support
Even the most reliable product requires proper maintenance and support. Strategies in this area include:
- Developing clear, comprehensive user manuals
- Offering preventive maintenance programs to customers
- Analyzing information collected from the field to identify common issues and develop solutions
Effective maintenance and support extend the life of products, enhance customer satisfaction, and provide valuable feedback for product improvements.

AI and Product Reliability
Artificial Intelligence (AI) is a game changer in product reliability, with tools and methodologies to enhance product performance and longevity.
AI in Design and Testing
AI is making significant inroads in product design and testing phases, starting with generative design, where AI algorithms generate multiple design options optimized for reliability and performance.
AI for product design leverages machine learning to predict potential failure modes based on historical collections of simulations.
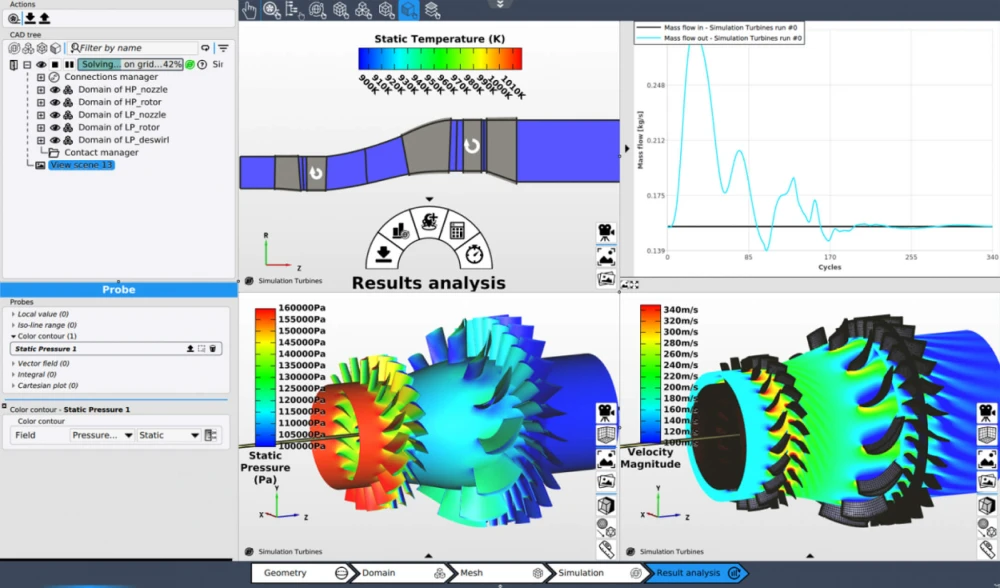
Automated testing with AI-powered systems conducts and analyzes complex tests more efficiently than traditional methods. AI also assists in choosing the optimal materials for specific applications. Additionally, ML algorithms aid in design optimization, fine-tuning designs to improve reliability while balancing cost and manufacturability.
These AI-driven approaches allow engineers to explore a wider range of design possibilities and identify potential reliability issues earlier in the development process.
AI in Predictive Maintenance
AI is transforming how companies approach product maintenance. AI in predictive maintenance can reduce downtime, extend the product life cycle, and improve overall reliability.
Here is a quick list of applications.
- In Predictive Analytics, AI algorithms can analyze information collected from sensors and IoT devices to predict when maintenance is needed, preventing unexpected failures.
- In Anomaly Detection, ML can identify unusual patterns in product performance that may indicate impending product failures.
- In Optimization of Maintenance Schedules, AI can help determine optimal maintenance intervals based on usage and environmental conditions.
- In Root Cause Analysis, AI-powered systems can identify the underlying causes of failures more quickly and accurately.
- With Digital Twins, AI-driven digital representations of products can simulate performance and predict maintenance needs.
Conclusions
Product reliability encompasses the technical aspects of design and manufacturing and impacts customer satisfaction, brand reputation, and profitability.
As engineers, our role in ensuring product reliability is more critical than ever, especially as products become increasingly complex and interconnected.
By focusing on the concept of reliable products throughout the product lifecycle—from initial design to ongoing maintenance and support in the warranty period—we can create products that not only meet but even exceed customer requirements.
The integration of advanced technologies like AI / ML offers exciting new possibilities for enhancing a product's reliability, allowing engineers to predict and prevent failures with unprecedented accuracy.