Transforming Engineering Design with AI: A New Era of Possibilities
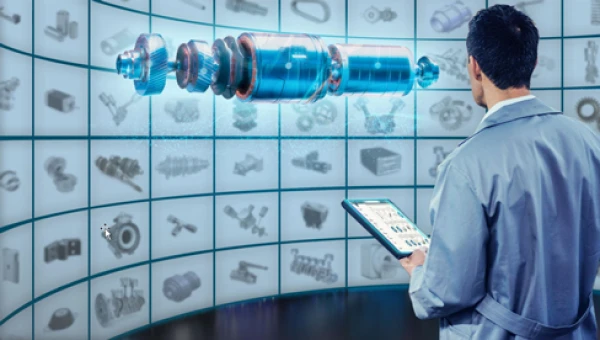
The future of engineering design is here, thanks to Artificial Intelligence (AI). AI has the power to completely transform the field, with advancements for engineers and ultimately for consumers for products and services ranging from autonomous vehicles to eye surgery plans, as we will see!
In this article, we will delve into the exciting and impactful application of AI in engineering design. You will discover how AI can help the way engineers approach design, and learn why and how AI is going to become your indispensable assistant in the next future.
Prediction vs Optimization in Design
We can harness the power of traditional Computer-Aided Engineering (CAE) into AI algorithms that can not only help to identify potential problems before they occur, but also allow real-time design space exploration with a data-driven AI predictive system.
What is moving the CAE simulation game to another level? It's the shift to a real-time prediction, from previous diagnosis or offline prediction.
CAE is already consolidated in several engineering applications, ranging from analyzing the risk of non-compliant products to design space exploration scenarios. Design space exploration in particular, however, requires days or weeks of computations. And this can be only partially alleviated with investments in the hardware infrastructure. AI data-driven simulation, on the other hand, opens up new perspectives for product innovation, by allowing engineers to make informed decisions and optimize their designs in real-time, with reliable predictions based on high-fidelity CAE data (figure).
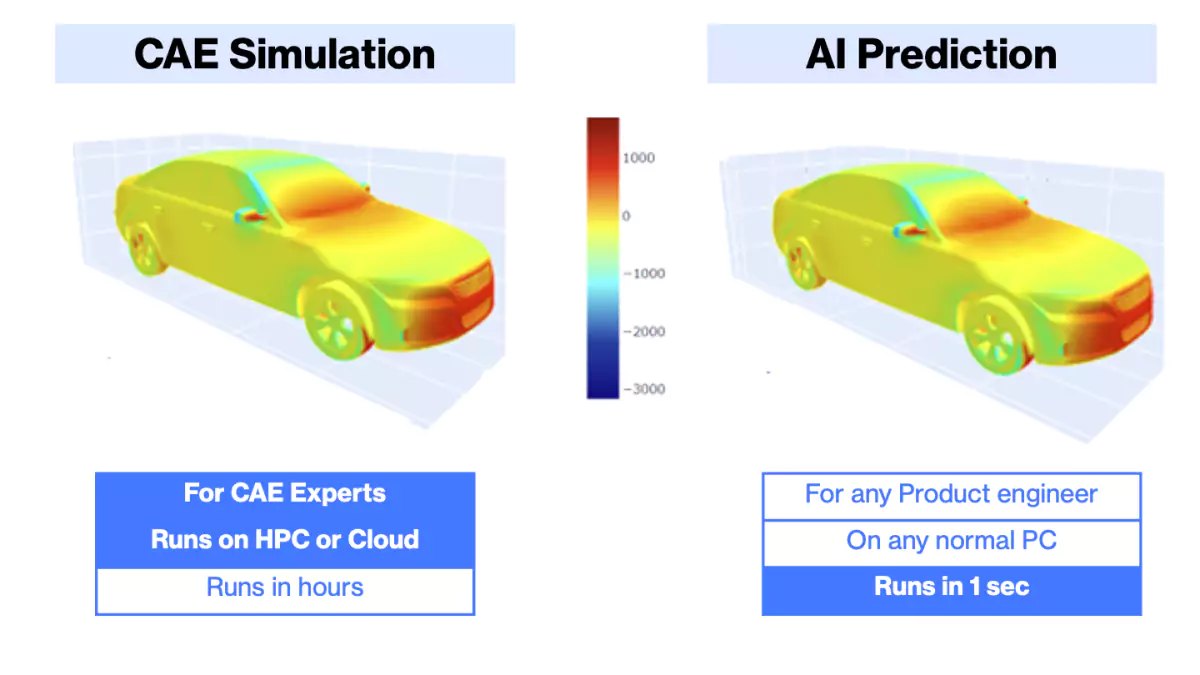
The usual "verification" or single-point simulation involves using predictive models to validate that a design will meet certain criteria and constraints. However, the true value of predictive modelling lies in its ability to be used in a closed loop with engineering targets. This allows designers to not only identify problems but also optimize their designs to reach the desired goals while respecting the imposed constraints.
Optimization algorithms, whether traditional or AI-powered, are at the heart of this closed-loop approach to predictive modelling. By continually adjusting and refining a design based on real-time feedback from predictive models, designers can ensure that their products meet their desired goals and perform as expected. This can include factors such as weight, strength, durability, and business factors.
Tackling Risk: From Predictive Modelling to AI
In the 90s, automotive companies were concerned with unreliable products and warranty returns. CAE predictive modelling helped increase their confidence in designs, diffusing from R&D centres to Product Design through consultancy services.
Predictive modelling, or simulating the behaviour of products and systems in different scenarios, provided valuable insights into potential problems before they occurred, improving design reliability and reducing risks associated with warranty claims and market share loss.
Predictive modelling is not limited to the automotive industry, as it can be applied to other industries prioritizing reliability and safety, including aerospace, shipbuilding, civil engineering, and life sciences AI applications (figure below), even targeting final users such as surgeons without any skill at all in simulation.

The next step for design is AI-powered predictive modelling, but before exploring that, it is important to consider the optimization side of the algorithmic approach..
Better Products With AI in Engineering Design: Generative Design Optimization
Product design has come a long way since the days of manual sketching and prototyping and laboratory testing. In a certain sense, this traditional system of trial and error could be reproduced with CAD instead of sketching and CAE instead of prototyping and testing. However, today, engineering departments can get access to powerful technologies that can help them create better products faster and more efficiently. One such technology is generative design optimization, which leverages AI to generate multiple design options based on specified targets and constraints.
The generative design approach involves transforming design targets into mathematical expressions and specified parameters and constraints. These expressions and parameters can then be optimized using algorithms, which propose changes to the product design-based engineering and business objectives.
What are the key benefits?
Design Speed Up
One of the key benefits of generative design is its ability to speed up the design process. Engineers can generate multiple design options in a fraction of the time it would take to manually create and evaluate each option. This not only saves time and resources but also allows for more iteration and exploration of the design space, increasing the chances of finding the optimal solution.
Reduce Total Cost
In addition to its time-saving benefits, generative design also offers the potential for cost savings. By evaluating designs based on cost as well as performance, engineers can make balanced decisions, reducing the risk of over-specifying or over-designing products such as example excessive thickness and therefore weight and total cost of components to prevent failures.
New Knowledge from Non-Human Sources
Another important advantage of generative design is its ability to identify design solutions that may not be immediately apparent to human designers. This can lead to creativity and innovation with unexpected design solutions that would not have been considered otherwise.
In conclusion, with AI algorithms, engineers can generate multiple design options based on specified targets and constraints, saving time and resources while also increasing the chances of finding the optimal design solution.
The Engineering Design Process - Review
Before giving details on AI, let us review the role of the product design department within engineering design projects. This department translates customer requirements into a set of technical specifications that can be used to guide the development of new products or processes. How does Engineering relate to other company teams?
Engineering Design in the Corporate Ecosystem
Engineering Design and Manufacturing teams
Engineering Design relates to Manufacturing since the former provides the technical specifications and designs that the latter use to manufacture products. The two departments work closely together to ensure products are designed with manufacturability in mind.
Engineering Design Department and Research & Development teams
The engineering design department works closely with other Research & Development departments and teams, including those focused on material science, manufacturing, and testing, to ensure that new products are designed and developed to meet customer requirements and deliver value to the company. Also, Research & Development is usually supplied with installations of CAE high-fidelity tools both to tackle research topics and to provide internal simulation services to product designers, although with various necessary iterations and unavoidable bottlenecks.
The role of AI (figure) is that of unchaining the power of CAE simulation but making it directly available to a vast majority of engineers and allowing them to explore the design space in real-time.
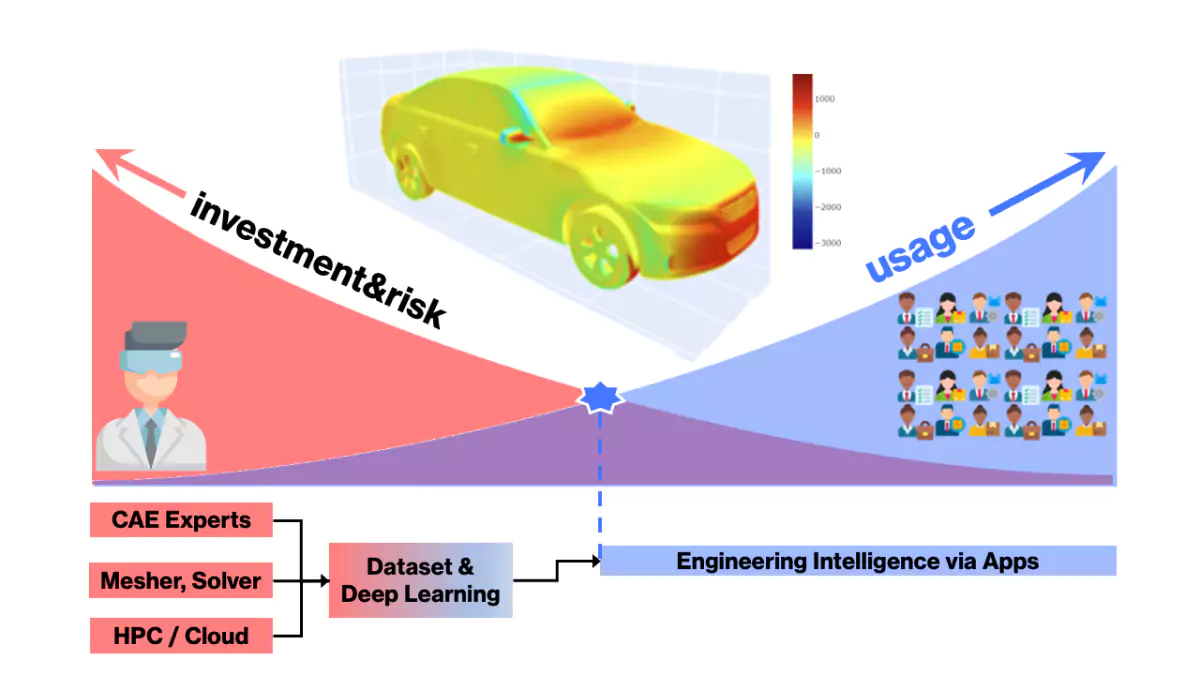
Engineering Design and PMs teams
Project managers ensure that project schedules are completed on time, within budget, and to the desired quality level. The engineering design department provides the technical knowledge and guidance necessary for project managers to make informed decisions and allocate resources effectively.
Engineering Design and Sales teams
Sales engineers work closely with the engineering design department to understand the technical aspects of the products and solutions and communicate this information to customers in a way that is easy to understand and resonates with their needs. The possibility of deploying a predictive solution on their desks, to facilitate for example the answers to RfQs (Request for Quotations) with much deeper information on the technical specifications of their products, thanks to AI-assisted engineering simulations that do not require specific expertise to the sales engineer.
Software Tools in Engineering Design Projects: Traditional Vision
State of the art in engineering design involves using advanced software for simulation, visualization, and optimization. This allows engineers to iterate and test designs more efficiently, reducing the need for physical prototyping and improving the accuracy of predictions. Some popular applications in this field include:
- CAD (Computer-Aided Design) — AutoCAD, SolidWorks, CATIA, NX, Creo
- PLM (Product Lifecycle Management) software
- CAE
This software is widely used in the automotive sector to design and optimize vehicle components, from engines and transmissions to bodies and interiors. Companies such as Ford, Toyota or BMW use them to improve their products and bring them to market faster and more efficiently.
For example, automotive companies use CFD software to test the aerodynamics of their vehicles virtually.
FEA software is used to optimize the structural integrity of their vehicles and reduce weight without sacrificing safety.

Artificial Intelligence: Basic Applications
AI is a rapidly growing field with tools that can assist humans in various tasks, from automating mundane tasks to making complex decisions.
One of the most popular AI tools is machine learning (ML); its algorithms are designed to find patterns in data and make predictions based on that data.
Another important set of AI tools is Computer Vision (CV), i.e. the ability to interpret and understand visual information from the world around a computer.
AI tools such as ML and CV are rapidly gaining popularity. As AI continues to evolve, we can expect to see even more powerful and versatile tools emerging in the future.
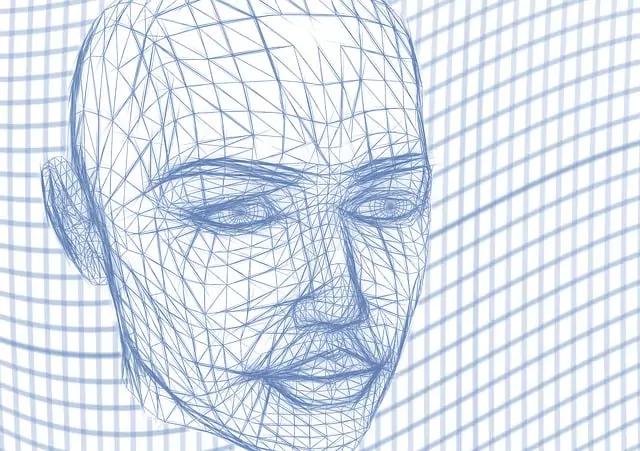
Artificial Intelligence: AI Models
Artificial Intelligence (AI) is a computer science branch with algorithms to perform tasks requiring human intelligence like visual perception or natural language processing; to do so, various AI models are used.
Supervised Learning is widely used for tasks like classification and regression. A machine learning algorithm is trained on a labelled dataset where the desired outcome is known, and this algorithm uses that data to learn to predict outcomes for new, unseen data.
Deep Learning, a type of supervised learning, utilizes artificial neural networks. These algorithms contain multiple interconnected layers that enable them to learn increasingly complex features and data representations, for visual recognition.
Artificial Intelligence in Engineering Design
Learning to Solve Engineering Design Problems
Solving problems for a designer, within today's global economy, requires a mix of innovativeness, timeliness and budget-effectiveness, which benefits from a combination of human skills and AI technology assisting them.
In this AI-assisted scenario, the role of designers is to deeply understand the problem and the customer's needs and, ultimately, to keep control of the whole process.
At the same time, the role of technology such as AI provides valuable insights and assistance in evaluating solutions and identifying potential design iterations.
Thus, by leveraging the strengths of both humans and technology, companies can develop more effective and efficient new designs.
CAE Surrogates
One example of how technology and AI experts can assist humans in solving engineering design problems is by using, or smartly recycling, the knowledge accumulated in previous designs to provide real-time predictive analytics (CAE surrogate) instruments.
Product designers can use previous designs as a starting point and AI algorithms to identify patterns and trends in those designs. This can provide valuable information on what design solutions have been successful in the past and what areas may need improvement. We described how AI in design engineering systems can effectively provide a simulation solution with AI.
Another example is the use of AI-based techniques for generative design.
These techniques can help engineers evaluate design alternatives and find the optimal solution. Experts in AI can use machine learning algorithms to analyze data on design alternatives and predict which new designs will be the most successful based on specific constraints and requirements (functional targets).
AI Applied to the Design Process: Aerodynamics Use-Case
Companies in the automotive and aerospace sectors spend thousands of hours of CAE and CFD on HPC clusters, to simulate different design configurations with several operating conditions for each design, to get a better insight into the aerodynamic behaviour of aircraft or ground vehicles.
Most of these cumulated CAE data are only used and never used again. Since there is no reason to make the same mistake twice, the AI solution from Neural Concept (NCS) learns from your heterogenous data with an overnight AI training process.
AI experts deliver overnight a predictive App, but the most exciting part of this process is that end-users can specify what type of output they need for the design process, in other words, the construction of the AI app is driven by design knowledge.
For instance, most aero engineers usually analyze only a few operating conditions, but this is due to time limitations. Since NCS allows the prediction of hundreds of results in a few seconds, spanning a range of flow velocities or angles, informed designers will choose the option of a predictive app that can predict a whole "flight envelope" (figure).
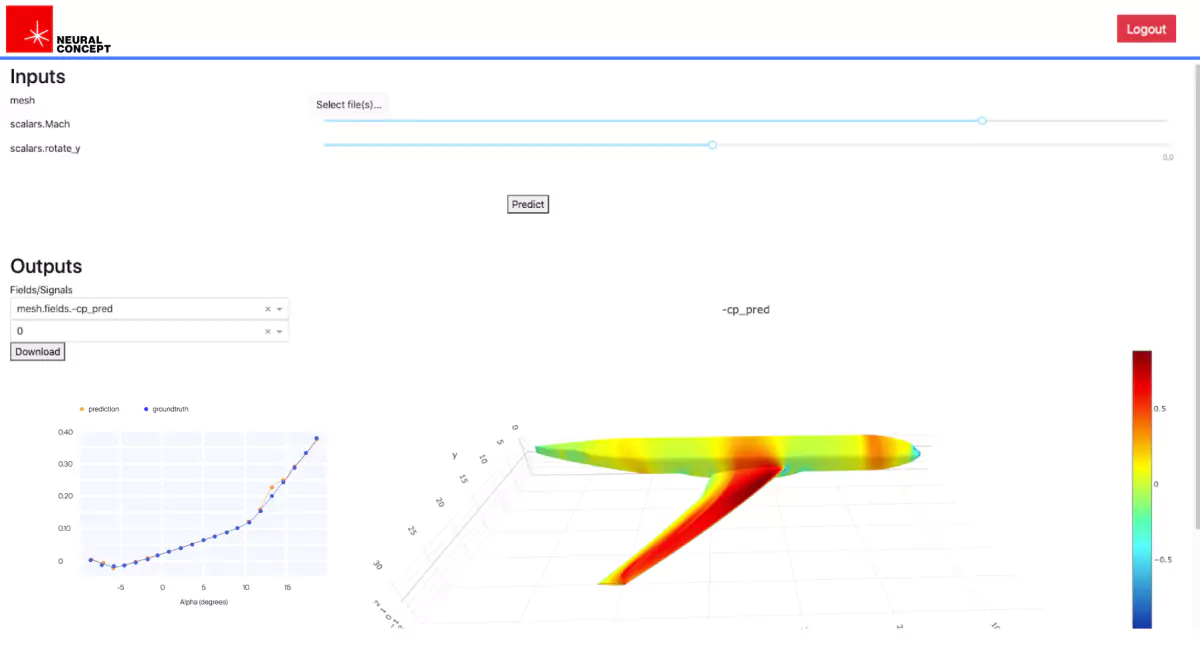
Conclusions
We have seen how the Product Design department is connected to many company functions ranging from R&D to Manufacturing. At the same time, high-fidelity CAE can provide accurate answers to support diagnostics on faulty products, compare solutions or explore design spaces up to generative design capabilities. However the speed of response represents a bottleneck, and the second bottleneck is the amount of skills required.
By re-using CAE data, AI with a Deep Learning predictive model can become an assistant on the desk of any designer, thanks to its capabilities to use data to support design evaluations. Thus, it is truly possible to increase the speed and the efficiency of the product design development.
Cover picture: engineering.com