The Top Applications of AI in Engineering You Should Know

Artificial Intelligence is often seen as a transformative force shaping our world, yet public perception tends to focus on large language models such as ChatGPT. Can AI systems go beyond conversations and tackle complex technical challenges like those encountered in engineering projects?
This article will show the top applications of Artificial Intelligence in Engineering that revolutionize engineering projects. Traditionally, engineering simulation depended on lengthy classical analyses of structures and fluid dynamics. The rise of AI tools powered by Machine Learning (ML) makes it possible for AI systems to act faster and in a deeper design space than ever.

With advancements in computer science and data analysis, engineers can now harness their datasets to power applications of Artificial Intelligence to their projects.
These predictive systems rely on complex AI algorithms and deep learning models to navigate and make decisions in real-world environments.
How AI Innovations are Transforming Engineering
How can applications of Artificial Intelligence be deployed in practice? Tools supported by an AI engineer capture AI engineering practices. The AI engineer implements ML algorithms in collaboration with applied engineers. Those are civil engineering, aerospace engineering, or mechanical engineering experts who do not need to be experts in AI algorithms. The Neural Concept’s platform bridges the gap between teams of data scientists, simulation experts, and CAD engineers. The link between AI and 3D simulation is the data processing of past simulations.
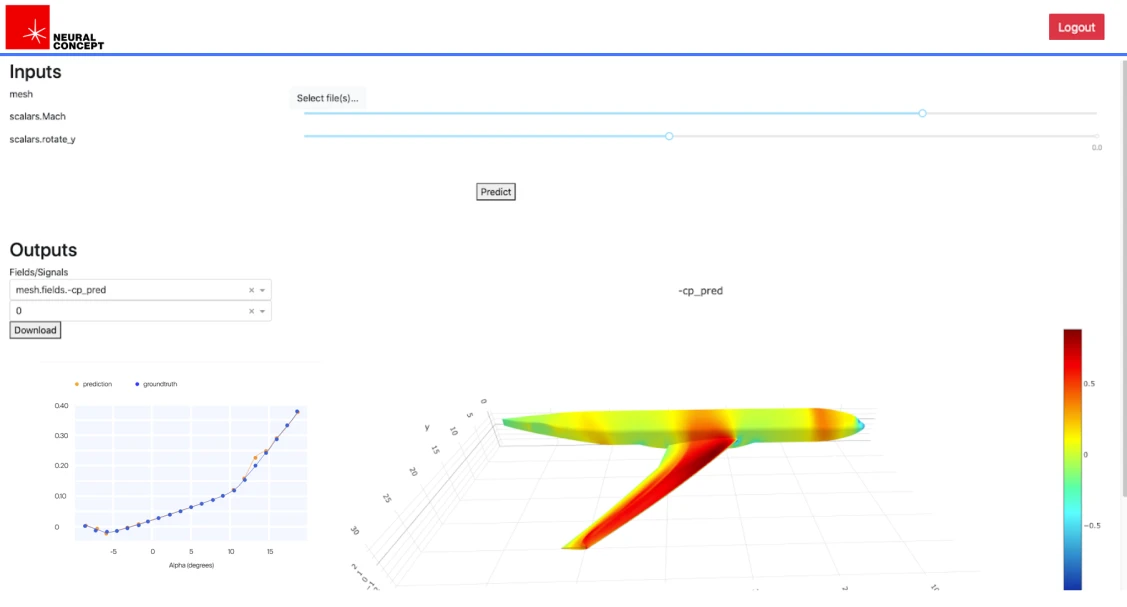
Emerging Applications of AI in Engineering
Additive manufacturing, i.e., 3D printing, enables engineers to create complex, lightweight, and highly customized structures.
Moreover, digital twins help engineers monitor and optimize physical assets in real time using virtual replicas. When augmented with Artificial Intelligence algorithms, twins can predict system failures, optimize maintenance schedules, and improve efficiency by making recommendations in dynamic environments.
Automation and robotics streamline production lines, improve precision, and ensure safety in hazardous environments.
Artificial Intelligence enhances these processes by enabling adaptive control, defect detection, image recognition, and real-time decision-making, minimizing downtime. Thus, AI technologies have contributed to attaining stricter security measures within real-world engineering applications.
Autonomous systems powered by AI are increasingly taking over repetitive and dangerous tasks, reducing the need for human intervention while maintaining high levels of accuracy and consistency. The application of autonomous systems is rapidly expanding to manufacturing, logistics, and agriculture sectors.
The Role of AI Solutions in Engineering: AI Engineering
Thus, AI engineering combines data science and AI technologies with engineering applications disciplines to enhance efficiency and promote innovation with a generative approach.
AI has proven instrumental in engineering applications such as predictive maintenance, process optimization, design optimization, and energy efficiency. It transforms industries from autonomous vehicles to adaptive manufacturing processes to data analytics by enabling smarter, faster, and more sustainable solutions.
Applications of AI in Engineering: A Brief Recap
“AI in engineering” means using artificial intelligence technologies to enhance engineering processes, design, analysis, and decision-making.
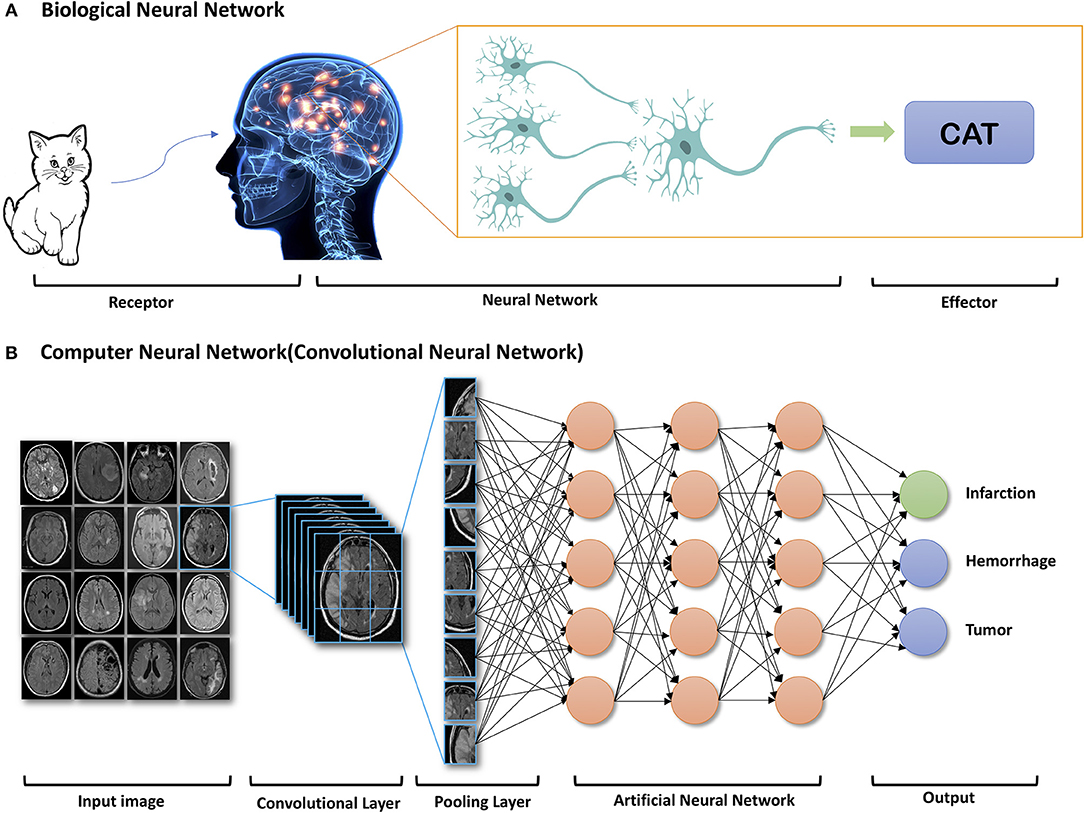
Scope of AI in Engineering
The application scope of AI in engineering spans diverse areas. It is essential in predictive maintenance, design, intelligent automation, real-time data analysis through digital twins, and adaptive control systems.
Artificial Intelligence can be used in electrical, mechanical, or civil engineering to simulate and optimize designs, predict failures, and explore innovative solutions that were previously unattainable using traditional methods. These applications are transformative in the automotive industry, aerospace engineering, and other sectors. Artificial Intelligence is no longer a technology display. It can solve complex engineering problems, such as in automotive manufacturing processes.
Evolution of AI in Engineering
The evolution of AI in engineering has marked milestones that transformed traditional practices. In the early stages, Artificial Intelligence was used for expert systems and rule-based design, aiding decision-making in niche engineering applications.
As computational power grew, adopting machine learning algorithms enabled the analysis of large datasets, paving the way for predictive maintenance and advanced simulation tools. The introduction of generative design was a groundbreaking step. AI algorithms began creating optimized designs by exploring configurations based on predefined constraints, revolutionizing architecture, and product development industries.
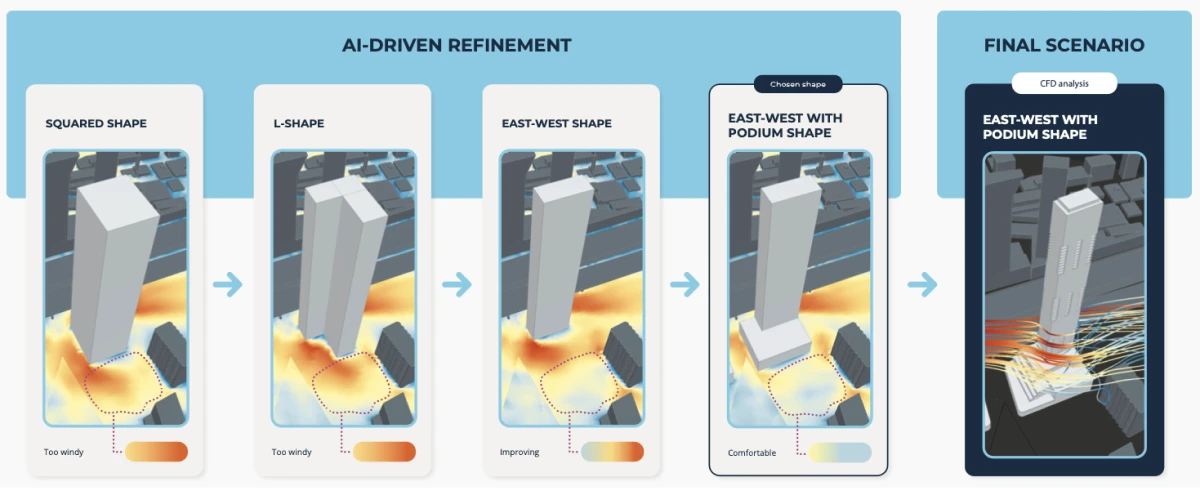
In recent years, real-time engineering applications tackled in real-time by AI solutions have redefined how to approach engineering systems and create value for wider audiences.
Artificial Intelligence-enhanced modeling of engineering applications ranging from aerodynamics to antenna design has enabled highly accurate virtual prototypes, drastically reducing development costs and timelines. Furthermore, advancements in natural language processing have facilitated automated design documentation, intelligent material selection, and seamless integration of engineering processes. These milestones show the applications of Artificial Intelligence’s crucial role in making engineering applications more innovative.
Core Applications of Artificial Intelligence in Engineering
AI influences decisions by providing quicker access to reliable information, thus accelerating decision-making in engineering workflows.
How can AI be quicker than traditional tools? AI can identify patterns and trends by analyzing vast environmental data sets. This capability allows engineers to enhance processes, foresee outcomes, and develop innovative solutions that would take significantly longer with conventional techniques.
Imagine you have 8 hours to simulate one or two engineering solutions with traditional engineering applications in a virtual space. AI tools let you review and rank 1,000 design changes simultaneously. This broader approach helps identify patterns and correlations that a limited scope might miss.
Design Optimization
AI enables engineers to generate and refine designs using generative AI and optimization algorithms. 3D Deep Learning allows engineers to input raw non-parametric geometries and parameters such as material constraints, load conditions, and manufacturing methods into novel solutions. With a 3D industrial “Image recognition” approach, deep learning technologies generate multiple viable designs that suggest novel solutions to designers.

Predictive Analytics with AI
ML algorithms integrated into traditional simulation workflows help engineers conduct faster and more precise simulations, enabling rapid iteration and innovation of new technologies.
In the application of Artificial Intelligence to the design of different types of heat exchangers, neural networks can predict results similar to CFD thousands of times faster. Designers can simultaneously simulate thousands of engineering configurations: “better, faster”!
The applications of Artificial Intelligence simulation range from heat transfer and CFD to structural analysis, electromagnetics, and molding.
.webp)
Quality Control and Environmental Impact with AI
Quality control processes in manufacturing are significantly improved via AI technologies.
AI-driven predictive maintenance reduces downtime and enhances asset utilization by combining machine learning with sensor data like temperature or vibration.
Machine learning algorithms analyze this data alongside historical records to identify failure-associated patterns. Algorithms recognize early warning signs and offer recommendations to prevent maintenance costs due to breakdowns.
Along the same lines, AI plays a crucial role in environmental impact assessment.
Applications of Artificial Intelligence to Digital Twins
Virtual replicas (digital twins) allow real-time monitoring, performance analysis, and predictive maintenance.
Real-world examples of AI-powered digital twins in manufacturing show significant efficiency and waste reduction improvements.
Konecranes, a Finnish company leader in material handling, utilizes digital twin technology in its smart factory to optimize maintenance schedules and prevent downtime.
Emerging and Future Applications of AI in Engineering
AI in smart infrastructures and self-driving cars enhances predictive maintenance and urban operations.
IoT devices monitor factory energy. AI analyzes data to identify inefficiencies, adjusts schedules for off-peak times, automates shutdowns, and optimizes HVAC for occupancy.

AI algorithms and Natural Language Processing (NLP) are advancing automation, decision-making, and communication. They also transform engineering practices by extracting insights from unstructured data and enabling dynamic models in robotics and predictive engineering. The rapid distribution of results from research conducted in engineering applications that employ AI helps engineers and researchers stay updated with the latest advancements.
Artificial Intelligence in Smart Infrastructures and Self-Driving Cars
AI-powered predictive maintenance systems monitor the health of mechanical structures in real-time, using sensor data and analytics to identify potential failures in bridges, tunnels, and roads before they occur, reducing costs and preventing disasters. By integrating AI in smart cities, we can optimize urban operations such as traffic flow, energy distribution, and waste management, adapting dynamically to changing conditions thanks to self-driving cars.
Artificial Intelligence and IoT Integration
AI and IoT streamline industrial systems with real-time data, facilitating condition-based maintenance that cuts downtime, increases efficiency, and optimizes resources as in the case of GE Vernova. AI algorithms analyze this data in real-time to detect anomalies, identify degradation patterns, and predict failures before they occur, making predictive analytics at GE Vernova a cornerstone of a strategy to enhance reliability and reduce maintenance costsò
Advancements in Algorithms
Advancements in AI algorithms are enabling systems to optimize decision-making in complex scenarios without explicit programming and human intervention. Adaptive simulations use AI to adjust models dynamically based on real-time data, improving accuracy in fields like autonomous systems and robotics. These breakthroughs promise smarter, more efficient solutions across engineering and technology domains.
For example, in autonomous driving, AI algorithms dynamically adjust vehicle control models using real-time sensor data, enabling safer navigation in unpredictable weather changes or traffic patterns without requiring pre-programmed responses.
The Role of Natural Language Processing
NLP-powered tools enable engineers to extract valuable insights from sources like research papers, patents, and manuals.
How does it work? NLP tools automate semantic search and keyword extraction. Summarization allows engineers to focus on solving complex challenges rather than manually sifting through information. Thus, AI plays a “mentoring” role, promoting cross-pollination between disciplines.
For example, imagine an engineer involved in fluid mechanics software development who needs a summary of an electrical engineering publication she would otherwise not have consulted, or vice versa, a summary of F1 aerodynamics for an engineer working in electronics control applications or data science.
Also, NLP is revolutionizing collaboration and communication among engineers through chatbots and virtual assistants, for instance, between a software user and her vendor’s technical support.

Conclusion and Looking Forward
AI has become a significant force worldwide, sparking transformations and revolutionary developments across many industries, including engineering. AI engineering helps designers and policymakers make informed decisions on their products.
AI applications in engineering, such as new technologies like Neural Concept’s AI engineering platform, allow engineers to optimize designs faster. Generative algorithms and simulations enable engineers to explore thousands of configurations, accelerating development cycles and reducing prototyping costs.
Any designer from any industry sector, from the automotive industry to aerospace and energy, can tackle engineering design challenges with deep learning AI systems without needing to be AI engineers.
The predictive tool based on Neural Concept technology is an input-output system that learns to identify patterns in the user’s historical data without forcing designers and engineers to become experts in specific software. This differs from classic software that forces a process on the user: here, AI learns to adapt to humans. Thus, designers can focus on their added-value engineering tasks and process optimization without dealing with tedious data preprocessing, algorithm tuning, or other specialist tasks.

FAQ
How can I use Artificial Intelligence as an engineer?
For example, you can leverage AI techniques to create generatively optimized designs based on specific parameters. AI-powered simulations with various machine learning models can predict performance under different conditions. AI can also identify patterns and anomalies in manufacturing. Additionally, Artificial Intelligence can automate repetitive tasks, freeing you to focus on innovation.
What are the most promising applications of AI in the future?
The future of AI in engineering lies in autonomous systems, sustainable design, and adaptive manufacturing. AI techniques will be deployed in self-optimizing autonomous systems, such as autonomous vehicles or smart grids, where real-time data is used to make adaptive decisions.
Can AI help small engineering teams compete with larger organizations?
Absolutely! Artificial Intelligence democratizes access to powerful tools that were once exclusive to large organizations with substantial resources. Provided they can access datasets or “pre-trained” models, even small teams can use AI for simulation and predictive maintenance, reducing costs and development times. The availability of public datasets is essential for AI-driven engineering projects and research.
What role does AI play in enhancing collaboration within engineering teams?
AI enhances engineering teamwork by streamlining data analysis and improving communication, coordination, and knowledge sharing. Digital twins and real-time simulations allow teams to collaborate on virtual models from any location. Natural language processing automates meeting notes and design reviews, while machine learning recommends solutions based on project history.
Does size matter in datasets?
AI thrives on high-quality data. Smaller, curated datasets outperform large, noisy ones, helping AI learn meaningful patterns instead of irrelevant information.