The Role of Structural Dynamic Analysis in Engineering Design

This article introduces the dynamic analysis process and characterizes some engineers' structural dynamics applications.
Even if we are not engineers, we are all intuitively familiar with the basic concepts of static analysis. An example of static analysis is determining the load-bearing capacity of a bridge by calculating the stresses and strains it will experience under various loads.
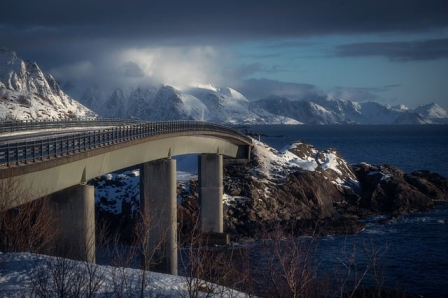
Dynamic simulation modeling is crucial for understanding, before the construction phase, how a structural system responds to time-varying or dynamic loading of external forces.
Therefore, dynamic and static analysis are quite different! Unlike static analysis, which deals with forces in equilibrium, dynamic analysis considers forces and motions that change with time. This type of analysis helps us predict and evaluate a structure's response when subjected to dynamic forces such as vibrations, impacts/shocks, seismic events, floods, or wind gusts.
Dynamic loads are applied as a function of time or frequency. This time-varying or frequency-varying load application induces time-varying or frequency-varying responses. Responses can be displacements, velocities, accelerations, forces, and stresses.
So why aren't we all approaching dynamic analysis? These time-varying or frequency-varying characteristics make dynamic analysis more complicated, although more realistic than static analysis.
We will conclude our exploratory article with the possible role of a 3D deep learning application in structural dynamics analysis.
Various Approaches to Structural Dynamics
This chapter introduces a toolbox of approaches to structural dynamics:
- frequency response analysis
- random vibration analysis
- shock response
- transient analysis.
All these techniques are important for mechanical engineers and provide a way to build high-quality datasets for the implementation of AI in mechanical engineering, including later data science elaborations such as predictive AI models.
Frequency Response Analysis
Frequency response analysis is a forced-response analysis commonly used in engineering to understand how structures behave when subjected to harmonic excitation, i.e., with a single frequency. The structure responds linearly to this input.
Frequency response analysis is indeed a dynamic analysis method. Still, it focuses on a specific aspect of dynamics related to steady-state behavior, meaning that it examines how the system behaves after transient effects have "settled down." Instead of analyzing time-dependent behavior, FRA operates in the frequency domain, providing information about each frequency's amplitude (magnitude) and response phase.
This method helps determine the steady-state response of a structure at any given location when subjected to harmonic excitation. It's particularly useful for assessing how structures respond to various dynamic forces, such as wind-induced vibrations, vortex shedding, or mechanical system excitation, such as vibrations caused by a pump.

For example, in civil engineering, frequency response analysis can help engineers predict how a bridge will respond to wind-induced vibrations. By understanding the structure's response to these dynamic forces, engineers can design safe and comfortable bridges for users.
In mechanical engineering, this analysis provides insight into how mechanical systems, like pumps or rotating machinery, affect the structures they are attached to. By analyzing the frequency response, engineers can identify potential issues, such as resonance or excessive vibrations, that may impact the system's performance or longevity.
In civil engineering, frequency response analysis can illuminate the worst-case scenario of pedestrian-induced vibrations in structures such as bridges or buildings. Engineers can optimize design solutions to minimize discomfort and ensure structural integrity by understanding how these vibrations propagate through the structure.
A Little Bit of Definitions
In structural engineering, natural frequency and mode shape are key concepts when studying the behavior of mechanical systems. The natural frequency of a mechanical system is the frequency at which it naturally vibrates when disturbed. It's an inherent system property and depends on mass, stiffness, and damping factors. A vibrating system's mode shape describes the pattern of motion that the system exhibits at a specific natural frequency. It shows how the system's components move relative to each other during vibration. Each mode shape corresponds to a specific natural frequency, and together, they form the modal analysis of the system.
Mathematically, once you solve for a system's eigenvalues (natural frequencies), you can find the corresponding eigenvectors representing the mode shapes.
Random Vibration Analysis
Random Vibration Analysis is a method used in engineering to analyze the response of structures to random excitations, such as turbulent air/fluid-induced vibrations or ground-borne vibrations. In this analysis, the excitation is characterized by a power spectrum, representing energy distribution across different frequencies.
The structural response to these random excitations is assumed to be linear, meaning that the relationship between the input (excitation) and the output (structural response) remains consistent across different excitation levels.
This analysis is crucial for understanding how structures, such as buildings, bridges, or aerospace components, respond to unpredictable and varying dynamic loads. For example, civil engineering helps assess the response of buildings and bridges to wind-induced vibrations or ground motions caused by earthquakes.
In aerospace engineering, it evaluates how aircraft structures respond to turbulent airflow during flight. Similarly, it's applied to understand how vehicle components respond to road-induced vibrations in automotive engineering.
The mathematical representation of random vibration analysis involves using power spectral density (PSD) functions.
The PSD describes the power distribution per unit frequency, denoted as Sx(f), where x represents the response variable (displacement, velocity, acceleration), and f is the frequency.
The relationship between the structure's input PSD Sinput (f) and output PSD Soutput (f) is often expressed using the Power Spectral Density Ratio (PSDR) function H(f) as S_output(f) = |H(f)| 2 * S_input(f).
This equation shows how the structure's response modifies the input power spectrum to produce the output power spectrum.
Shock Response Analysis
Shock Response Analysis is a tool for evaluating how structures respond to sudden and intense dynamic loads, such as those caused by earthquakes or explosions.

In this form of forced-response analysis, the excitation is characterized by a shock spectrum, representing the energy distribution over a range of frequencies associated with the shock.
The structural response to these shock inputs is assumed to be linear, meaning that the relationship between the input shock and the resulting structural response remains consistent, regardless of the magnitude of the shock.
This analysis primarily assesses the structural response to seismic events like earthquakes. The American Society of Civil Engineers (ASCE) defines earthquake shock spectra for different regions within the United States, providing engineers with standardized data for analysis.
Seismic design parameters, such as peak ground acceleration (PGA) and spectral acceleration, can be obtained from organizations like the United States Geological Survey (USGS).
Transient Dynamic Analysis
This is the most general but computation-intensive form of forced-response structural dynamics analysis. Transient dynamic analyses consider the entire time history of the response, including transient effects.
While computationally intensive, it is the only analysis capable of capturing nonlinear structural response characteristics (e.g., material yielding and local failure) and discrete fluid viscous dampers (e.g., in tuned-mass dampers).
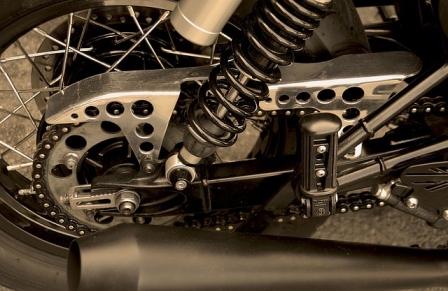
Shock response spectra can be synthesized into equivalent ground motion time series to assess the response of any structure to any earthquake design requirement.
Mathematically, transient dynamic analysis involves solving the equations of motion, typically represented as second-order differential equations:
M d²2u/dt² + C du/dt + K * u = F(t)
where M is mass, C is damping, K is stiffness, u is the displacement, and F(t) is the time-dependent external force.
Analytical solutions are typically difficult for the above equation, especially for complex structures and loading conditions. A few cases exist where simplified analytical solutions exist, such as for simple mass-spring-damper systems subjected to specific loads. However, analytical solutions are often impractical or non-existent for real-world engineering problems involving complex structures and dynamic loading conditions.
This is where Finite Element Analysis (FEA) comes into play.
Starting from a CAD description, FEA discretizes the structure into smaller elements, allowing for the approximate solution of the differential equations governing structural behavior.
Here's why FEA is preferred for transient dynamic analysis.
FEA can handle complex geometries, material properties, and loading conditions that can often not be addressed analytically. Real-world structures are rarely simple, and FEA provides a versatile approach to accurately modeling and analyzing their behavior.
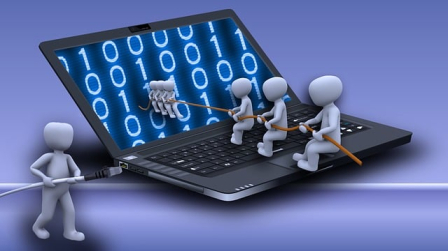
FEA can capture nonlinear behavior such as material yielding, large deformations, and contact interactions. Analytical solutions often rely on linear assumptions, which may not apply to many engineering problems.
Transient dynamic analysis deals with time-varying loads, making it challenging to derive analytical solutions. FEA allows for the simulation of these loads and the resulting structural response over time.
FEA allows engineers to apply complex boundary conditions and constraints, which are often difficult to handle analytically.
While analytical solutions may provide insights into simplified models, FEA can provide more accurate results by considering the full complexity of the structure and loading conditions. Advanced FEA software packages offer robust capabilities for transient dynamic analysis, making it accessible to engineers and efficiently automating complex problems.
Further Info - Implicit & Explicit FEA
Implicit Dynamic Analysis (e.g., to name a commercial software, with ABAQUS/Standard) solves nonlinear equilibrium equations at each time increment, which is suitable for nonlinear dynamic response studies.
Explicit Dynamic Analysis (e.g., ABAQUS/Explicit) calculates displacements and velocities based on known quantities. In such a case, there is no need to form and invert global mass and stiffness matrices. Conditional stability limits the range of applications; small time increments are required.
The Role of Structural Dynamics in Engineering Design
We will briefly summarize why understanding dynamic behavior is crucial in engineering design, emphasizing its role in ensuring the structures' safety, reliability, and optimal performance in various fields.

Risk Mitigation
Structural dynamic analysis aids in risk mitigation by identifying weak points. Dynamic simulations reveal vulnerable areas prone to failure during earthquakes, windstorms, or other dynamic events. Engineers can reinforce these regions or modify the design to enhance safety.
Engineers assess their impact on structural components by analyzing variable loads (e.g., wind gusts, traffic-induced vibrations). This knowledge informs design modifications and load-bearing capacity adjustments.
Dynamic models predict how structures respond to sudden loads, ground motion, or impact. This information helps engineers make informed decisions to prevent catastrophic failures.
Design Optimization
Structural dynamics aids in optimizing designs for efficiency and cost-effectiveness.
Dynamic analysis helps optimize structural shapes to minimize aerodynamic drag, reduce vibrations, and enhance stability. Finding the right form improves the overall performance of bridges, towers, and other structures.
Dynamic simulations consider material properties (e.g., stiffness, damping) under varying loads. Engineers choose materials that balance strength, weight, and cost while meeting performance requirements.
Dynamic analysis provides insights into load distribution and stress concentrations. By avoiding overdesign, engineers create efficient structures without compromising safety.
Cost and Time Savings in Design Iterations
Early analysis and iterations based on structural dynamics can save time and resources in the design phase. Early dynamic analysis leads to significant savings. Identifying issues during the design phase prevents costly redesigns later. Dynamic simulations catch problems early, allowing engineers to adjust before construction begins.
Engineers optimize material quantities by understanding dynamic behavior. This reduces waste and lowers material costs.
Applications of Structural Dynamics
Dynamic structural analysis is helpful in aerospace, civil, and automotive engineering. It enables the design of safe, reliable, and efficient structures that withstand loads and environmental conditions. We will explore the critical role of Dynamic Analysis in modern engineering practices.
Aerospace Engineering Applications of Structural Dynamics
Dynamic structural analysis (vibrational or modal analysis and response to dynamic loading) is indispensable in aerospace engineering for designing and ensuring the safety of aircraft and spacecraft components under various operational conditions.
- Vibration Analysis: Engine Mounts in an Aircraft Engine. Vibration analysis ensures that engine mounts can withstand the dynamic forces produced by the engine's operation, preventing structural fatigue and ensuring passenger comfort.
- Modal Analysis: Wings of an Aircraft. Modal analysis helps determine the wing structure's natural frequency and mode shapes to prevent resonance and fatigue failure during flight maneuvers or turbulent conditions.
- Response to Variable Loads: Fuselage Structure of an Aircraft. Time history analysis of the fuselage's response to loads, such as turbulence during flight, ensures its structural integrity and safety for passengers and cargo and provides much better confidence than the response to a static load.
Civil Engineering Applications of Structural Dynamics
We will review seismic analysis applications, wind load, and bridge analysis.
- Seismic Analysis of Skyscrapers: Seismic analysis ensures that tall buildings can withstand the dynamic forces generated by earthquakes without collapsing by analyzing the behavior of building components like columns and beams under seismic loads.
- Wind Loads and Structural Dynamics of Suspension Bridges: Dynamic analysis of suspension bridges helps engineers understand how they respond to wind loads, ensuring their stability and safety for vehicles and pedestrians during windy conditions.
- Bridge Deck Dynamic Analysis. Dynamic structural analysis of bridge decks helps engineers evaluate their response to variable traffic loads, preventing excessive vibrations and ensuring long-term durability and safety.
Automotive Engineering Applications of Structural Dynamics
We will finally review three Dynamic Analysis applications in the Automotive industry.
- Crashworthiness Analysis of Frontal Crash Structure. Engineers use dynamic structural analysis to design and test the front-end structure of vehicles, which absorbs and dissipates energy during a crash, protecting occupants in the passenger compartment.
- Vehicle Dynamics - Suspension System. Dynamic analysis of suspension components helps engineers optimize vehicle handling and ride comfort by analyzing factors such as stiffness, damping, and tire interaction during cornering, braking, and acceleration.
- NVH (Noise, Vibration, and Harshness) Analysis of Car Body Structure. NVH analysis involves studying the dynamic response of the car body to reduce noise and vibrations experienced by passengers. Dynamic structural analysis helps identify and address noise and vibration sources, such as engine and road noise, by optimizing component stiffness and damping properties.
Possible Role of Deep Learning
Deep learning is a branch of artificial intelligence in which algorithms are trained to learn from data and make predictions or decisions. It is now in a phase that is mature enough to provide exciting use cases in modal analysis and structural dynamics with such important quotes straight from exerts in structural dynamics analysis:
"For the considered application, NC performs clearly better than currently used surrogate models and therefore we see the potential of NC for more use-cases."
Deep learning algorithms are designed to mimic the structure and function of the human brain's neural networks, enabling them to learn directly from raw data or features.
Artificial neural networks (ANNs) with multiple layers (hence the term "deep") are the building blocks of Deep Learning.
These layers consist of interconnected nodes or neurons, each performing simple computations. Deep learning algorithms use these neural networks to automatically learn hierarchical representations of data, with each layer extracting increasingly abstract features from the input data.

Applications of Deep Learning to Engineering
With experimental testing and CAE simulation data, deep learning can learn to predict and mitigate the effects of loads, thus enhancing the reliability and longevity of mechanical systems.
Deep Learning, feeding on the data generated by finite element analysis, can aid project teams with new insights that are within the reach of any engineer, thanks to the almost real-time speed or response. Even more appealing to designers, this allows them to explore a wider design space in the same amount of time, say 100 to 1'000 or more virtual design changes. This evolution is similar to the one witnessed in the 80s and 90s when organizations started to adopt simulation to complement experimental laboratory testing.
The ideal application of Deep Learning prediction is to be deployed very early in the design process, thus bringing benefits to program managers, cost estimators, technical sales engineers, and CAD design engineers.
While Deep Learning offers great potential, it’s essential to validate its predictive models rigorously and continuously update them as new data becomes available. Platforms such as Neural Concept (NC) offer skilled experts the opportunity to take care of the neural network's initial training phase while deploying intuitive apps that deliver turnkey solutions in the hands of most engineers who do not need to be experts in Deep Learning, Data Science, or Simulation.
As new data become available from numerical analysis or experimental laboratory testing, they can be integrated into NC to enhance the realism and accuracy of Deep Learning predictions and their impact on product design.