The Impact of Efficient EV Battery Thermal Management

Electric vehicles rely on complex battery systems to function.
A critical battery component is the Battery Thermal Management System, known as BTMS.
The Battery Thermal Management System technology regulates battery temperature, directly affecting an electric vehicle's performance, safety, and longevity.
A well-designed Battery Thermal Management System keeps a battery within their optimal temperature range, typically between 20°C and 40°C. Outside this range, batteries suffer! Cold temperatures increase internal resistance, reducing power output and range. High temperatures accelerate degradation and, in extreme cases, can lead to dangerous thermal runaway.
An efficient Thermal Management System gives several benefits, such as extended driving range; faster charging capabilities; longer lifespan of the battery pack; and finally, enhanced safety.

Understanding BTMS for EV
Battery thermal management refers to maintaining optimal operating temperatures for the cells within an electric vehicle. This system is crucial because battery performance, lifespan, and safety are highly dependent on temperature.
The primary objectives of battery thermal management include:
- Maintaining the battery temperature within an optimal range
- Ensuring uniform temperature distribution across the battery pack
- Thermal runaway prevention and other temperature-related safety issues for the battery pack
- Optimizing battery performance while extending its lifespan
Temperature Control
Lithium-ion batteries i.e. Li ion battery cells are commonly used in modern electric vehicles. Those EV batteries are sensitive to temperature fluctuations. The optimal temperature range for these EV batteries typically falls between 20°C and 40°C. This hlghlights the importance of the EV battery cooling system for maintaining the battery within its optimal range.
The main reasons are:
1. EV Batteries operate most efficiently within their optimal temperature range, providing maximum power output and range from the battery pack
2. Keeping the battery pack at the right temperature reduces degradation, extending its lifespan.
3. Preventing overheating minimizes the risk of thermal runaway, which can lead to fires or explosion of the battery pack and surroundings
4. Optimal battery temperatures allow for faster charging rates and better energy absorption.
EV batteries generate heat during charging and discharging cycles, which must be effectively managed to prevent overheating and ensure battery pack longevity. The process of dissipating heat to the ambient temperature relies on the principles of convective heat transfer. Active cooling methods such as forced air or liquid cooling are preferred to enhance heat dissipation to the ambient temperature considered as heat sinks.

Types of Battery Thermal Management Systems
The main types of battery thermal management systems include air cooling systems, liquid cooling systems, and phase change materials. We will review several types of heat exchanger design in the following paragraphs,
Air Cooling Systems
Air cooling is one of the simplest and most cost-effective methods of battery thermal management. Ambient or forced air transfers heat away from the battery cells. Passive air cooling relies on natural convection to dissipate heat, while active air cooling uses fans or blowers to force air across the battery pack, enhancing heat transfer.
The rate of transfer of heat Q (W) in an air cooling system can be described by Newton's Law of Cooling:
Q = h A (T_s - T_∞)
Where: h = convective heat transfer coefficient (W/m²·K), A = surface area of the battery (m²), T_s = surface temperature of the battery and T_∞ = ambient air temperature (K).
The coefficient (h) depends on factors, including air velocity and surface geometry and typically ranges from 10 to 100 W/m²·K for forced flow.
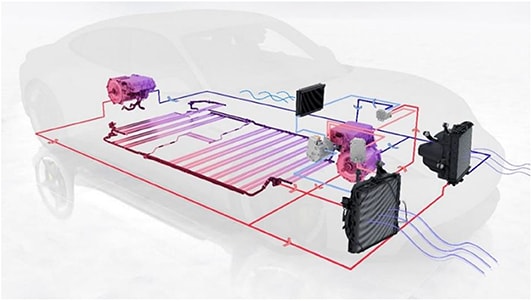
Cold Plate Vs. Air Cooled Systems
Cold plates are an active liquid cooling approach used in electric vehicle batteries as a part of the battery thermal management system to control battery temperatures within the prescribed limits for the battery pack. The cold plate design is engineered to transfer heat away from the battery cells to external heat exchangers, tomaintain an optimal operating temperature. It typically has channels through which a coolant (usually a mixture of water and glycol) flows.
Battery Cooling System - Liquid Cooled
A liquid cooling system is more efficient at transferring heat than air. In active cooling systems, a liquid coolant circulates through cooling plates or channels in direct contact with cells. The heated liquid coolant then passes through a radiator or heat exchanger to dissipate the heat.
The energy balance equation can describe the rate of transfer of heat (W) in a liquid cooling system:
Q = ṁ · c_p · (T_out - T_in)
Where ṁ = mass flow rate of cooling liquid, c_p = specific heat capacity of coolant, T_out = coolant temperature at outlet and T_in = coolant temperature at inlet.
The overall coefficient (U) for the transfer of heat in a liquid-cooled battery system, considering the thermal resistance of the coolant, cooling plate, and battery cell, is expressed as:
1/U = 1/h_c + t_w/k_w + 1/h_b
Where h_c = convective transfer coefficient of coolant, h_b = effective transfer coefficient at the battery-plate interface (W/m²·K); t_w = thickness of cooling plate wall and k_w = thermal conductivity of cooling plate material.
Phase Change Materials (PCMs)
Phase-change materials absorb or release heat as they change from solid to liquid , or vice versa. When integrated into a BTMS, PCMs can help regulate temperature by absorbing excess heat during high-load conditions and releasing it when the battery cools.
The equation can describe the heat storage capacity (J) of a PCM of mass m (kg):
Q = m · [c_s · (T_m - T_i) + L + c_l · (T_f - T_m)]
Where: c_s = specific heat capacity of solid PCM and c_l = specific heat capacity of liquid PCM, L = latent heat of fusion (J/kg), T_i = initial, T_m = melting and T_f = final temperatures.
PCM Practical Example
A PCM with a mass of 2 kg has specific heat capacities: c_s = 2 and c_l = 3 J/kg·K (liquid) with Latent heat of fusion: L = 200 J/kg. Using for temperatures Initial T_i = 300, Melting T_m = 310 and Final temperature: T_f = 320 K and plugging these values into the equation, Q = 2 [2(310 - 300) + 200 + 3(320 - 310)] = 500 J.
This shows the PCM can store 500 Joules of heat energy through its phase change and temperature rise, illustrating its capacity to manage thermal loads effectively.
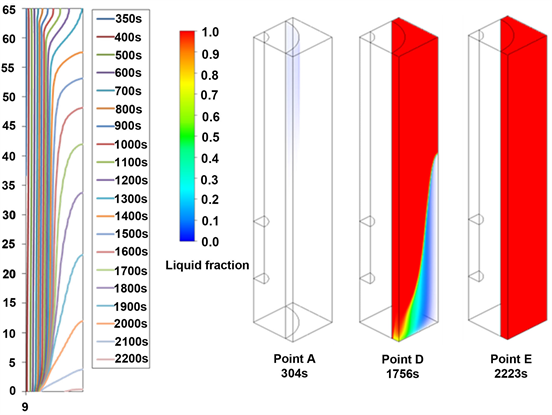
Passive Cooling Methods and Sizing of Cooling Time
Natural convection and conduction are at the basis of passive cooling systems that can dissipate heat generated during driving and light charging scenarios.
However, while passive cooling can be effective in normal situations, it may not be sufficient for high-performance or fast-charging conditions with more heat generation. Therefore passive cooling is supplemented with active cooling methods for thermal management to prevent overheating.
To compare phenomena, consider that:
- Typical values for natural convection h in air are around 2 to 25 W/m²·K (say 10 W/m²·K)
- Typical values for forced convection h in air are around 10 to 100 W/m²·K (say 50 W/m²·K)
- Typical values for forced convection h in water are around from 500 to 10,000 W/m²·K (say conservatively 500 W/m²·K)
Given the same surface area A and temperature difference, we compare the Q rates:
Qforced,air flow = 5 · Qnatural,air; Qforced, air flow = 10 · Qforced,air flow
In short, the cooling time t is inversely proportional to h, i.e. t ∝ 1/h and assuming for natural convection a characteristic cooling time t_natural ∝ 1 (say any standard time, 1 hour or day), we would have, to reach a certain temperature T(t) all the rest being equal, for active cooling: t_air ∝ 0.2 Vs. for active liquid cooling: t_liquid ∝ 0.02
This quantitative example highlighted that air cooling significantly reduces the cooling time compared to natural convection, and active liquid cooling provides an even greater reduction in cooling time. This explains the efficiency of active cooling methods, particularly liquid cooling, in managing thermal loads in battery packs.
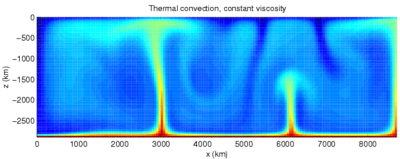
Impact of Thermal Management and Optimal Performance
Efficient battery thermal management profoundly impacts various aspects of electric vehicle performance, including range and efficiency, charging speed and efficiency, battery longevity, and safety enhancement. Fast charging is a key selling point for many electric vehicles, and efficient thermal management is crucial. A well-managed battery temperature allows for higher charging currents without damaging the cells. Maintaining optimal temperatures during charging enables the BTMS to transfer faster and more efficient energy. Effective cooling during fast charging helps prevent accelerated battery degradation caused by heat generation.
One of the most significant impacts of efficient thermal management is battery lifespan. By preventing exposure to extreme temperatures, an effective BTMS slows down the natural degradation process of battery cells. Maintaining consistent temperatures across the battery pack ensures that all cells age at similar rates, preventing premature failure of individual cells. Optimal range of temperature management can increase the number of charge-discharge cycles for battery pack before reaching its end-of-life capacity.
Safety is paramount in electric vehicles, and thermal management is critical. BTMS reduce the risk of thermal runaways, potentially catastrophic event swhere battery cells rapidly release energy in the form of heat. An efficient BTMS allows EVs to operate safely in a wide range of ambient temperatures, from sub-zero cold to scorching heat. The battery thermal management system helps maintain the structural integrity of the battery pack over time by preventing extreme temperature fluctuations.

Future Developments in BTMS
Research and development in BTMS are focused on several promising areas:
1. Developing new types of thermal interface material and composites that conduct heat could enhance heat transfer efficiency within battery packs.
2. Integrating artificial intelligence and machine learning algorithms could lead to more adaptive and predictive management strategies, optimizing performance based on driving conditions, weather forecasts, and individual usage patterns.
3. Direct immersion of cells in dielectric fluids is being explored as a potentially more efficient cooling method for high-performance applications.
4. Holistic approaches integrating BMTSs with overall vehicle thermal systems (including cabin climate control) could lead to significant efficiency gains.
5. The development of solid-state batteries may revolutionize thermal management, as these batteries are expected to have different thermal characteristics and potentially lower cooling requirements.

Conclusion
BTMS are essential in electric vehicles for regulating the battery's temperature, which impacts performance, safety, and lifespan.
Effective BTMS ensures batteries operate within a temperature range, maximizing power output, enabling faster and safer charging, and significantly extending battery lifespan.
By maintaining stable temperatures, BTMS also enhances safety by reducing the risk of runaway.
Despite progress, ongoing challenges include developing cost-efficient systems and adapting to new battery technologies and higher energy densities.