Superior prediction accuracy in Engineering: LS Electric collaborates with Neural Concept
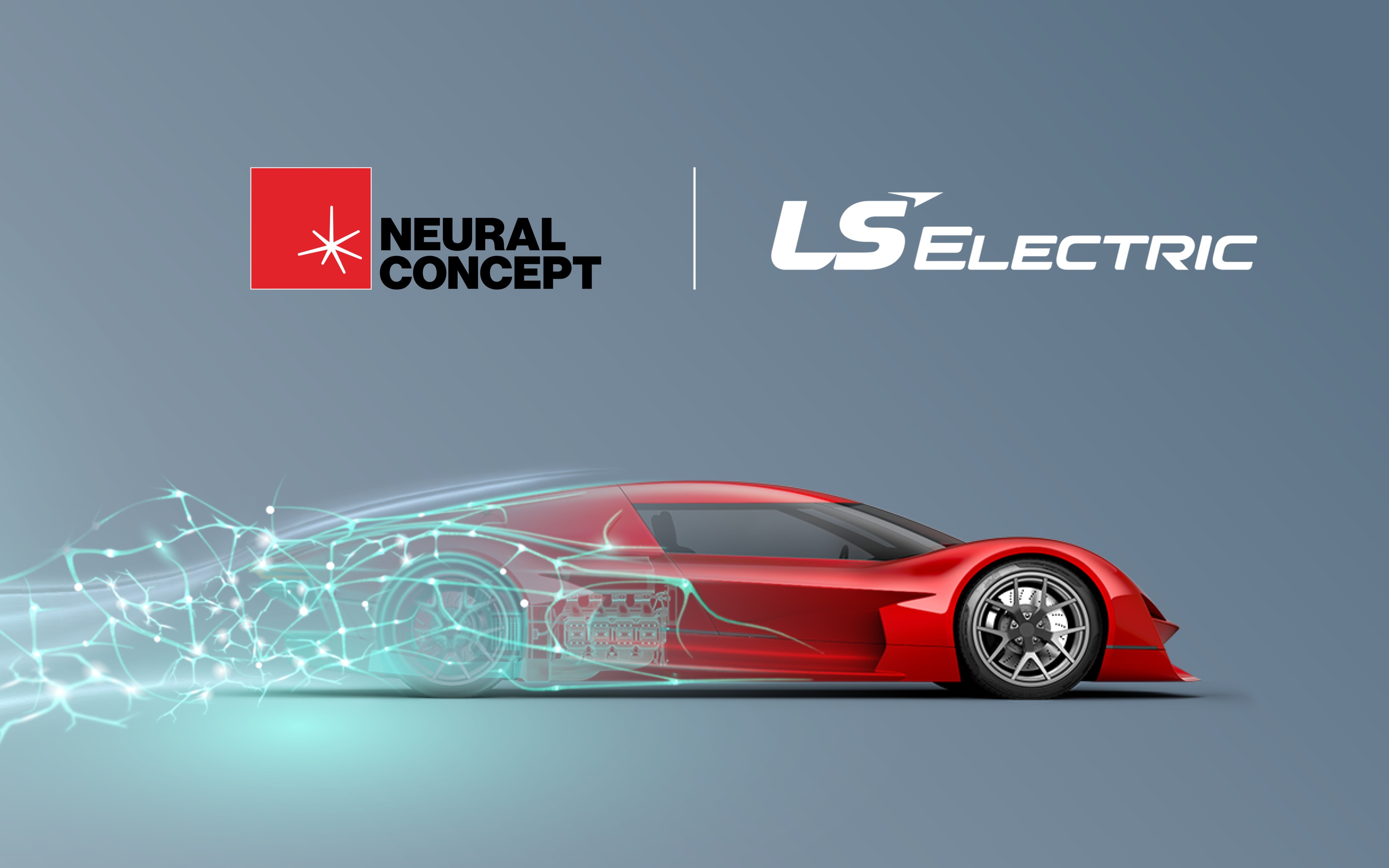
Global smart energy leader LS Electric has published a new technical paper demonstrating how Neural Concept is enhancing engineering efficiency by offering superior prediction accuracy for Mold Transformer Electrical Properties compared with existing design formulas.
The technical paper identifies how Neural Concept can be successfully deployed into automated electrical design environments and underscores the potential of 3D deep learning to accelerate Engineering Intelligence, shortening design lead times, dramatically improving efficiency and reducing the cost of design failure.
Neural Concept’s solution exhibited a high learning reliability, achieving an accuracy rate of over 99% for all three target variables.
Optimizing mold transformer predictions using AI
As engineering teams look to embed AI within production processes, it must be used in a targeted way to deliver tangible results in the areas of greatest need.
For example, mold transformers are widely used in various indoor environments such as buildings, hospitals, and factories due to their excellent insulation performance, high reliability, and stability.
Designing mold transformers with the correct electrical properties to meet the requirements of installation is crucial not only to ensure a stable power supply but also to optimize product costs for manufacturers.
This technical study was conducted to evaluate the feasibility of an AI-based mold transformer electrical characteristic prediction model, developed by learning the design and test data of mold transformers.
Enhancing reliability with neural networks
Prediction accuracy is crucial for engineering teams to identify the most reliable design plan. Data-driven design methods are commonplace, but if prediction deviations can be further reduced, production processes can accelerate reliably, enabling manufacturers to bring better products to market faster.
To test whether the convergence of AI with existing design and production technologies could be made to optimize prediction accuracy, Neural Concept provided an artificial neural network model to predict the main electrical design characteristics of mold transformers, namely, load loss, no-load loss, and % impedance.
Using the Neural Concept platform with Geodesic Convolution Neural Networks (GCNN) deep learning technology, the study shows that AI can be integrated into existing mold transformer electrical design automation and 3D CAD structural design automation environments with great success.
Implementation & results
The development of the neural network was conducted in three stages:
· Pre-processing;
· Training;
· Validation of the prepared dataset, according to a pre-defined neural network training process.
The training dataset of the AI model consisted of 80 main input variables selected from a total of 300 mold transformer design factors, and 3 target variables including load loss, no-load loss, and % impedance.
The AI model exhibited a high learning reliability, obtaining a coefficient of determination of more than 0.99 for all three target variables. This level of accuracy ensures that the AI model can predict these critical parameters with near-perfect accuracy. This is essential in applications like mold transformer design, where small deviations can lead to significant performance issues or increased costs.
Compared with the existing design formula, it was found that the prediction deviation was reduced and the prediction accuracy was improved. This means that, using artificial neural networks, the reliability of predictions can be enhanced, enabling data-driven design and unlocking innovation.
Conclusion
The AI prediction model for mold transformer electrical properties developed in this study can be integrated into existing mold transformer electrical design automation and 3D CAD structural design automation environments.
By applying this real-time and highly accurate electrical property prediction to the design process, manufacturers can shorten design lead times, dramatically improve efficiency, and significantly reduce costs associated with design failures.
This study validates a specific use case for AI to be integrated within production workflows, providing a guide for engineering teams to leverage advanced technology and drive product innovation.
Download the technical paper in Korean here.