PSA Groupe using NCS to Optimize the Performance of Next Generation Vehicles
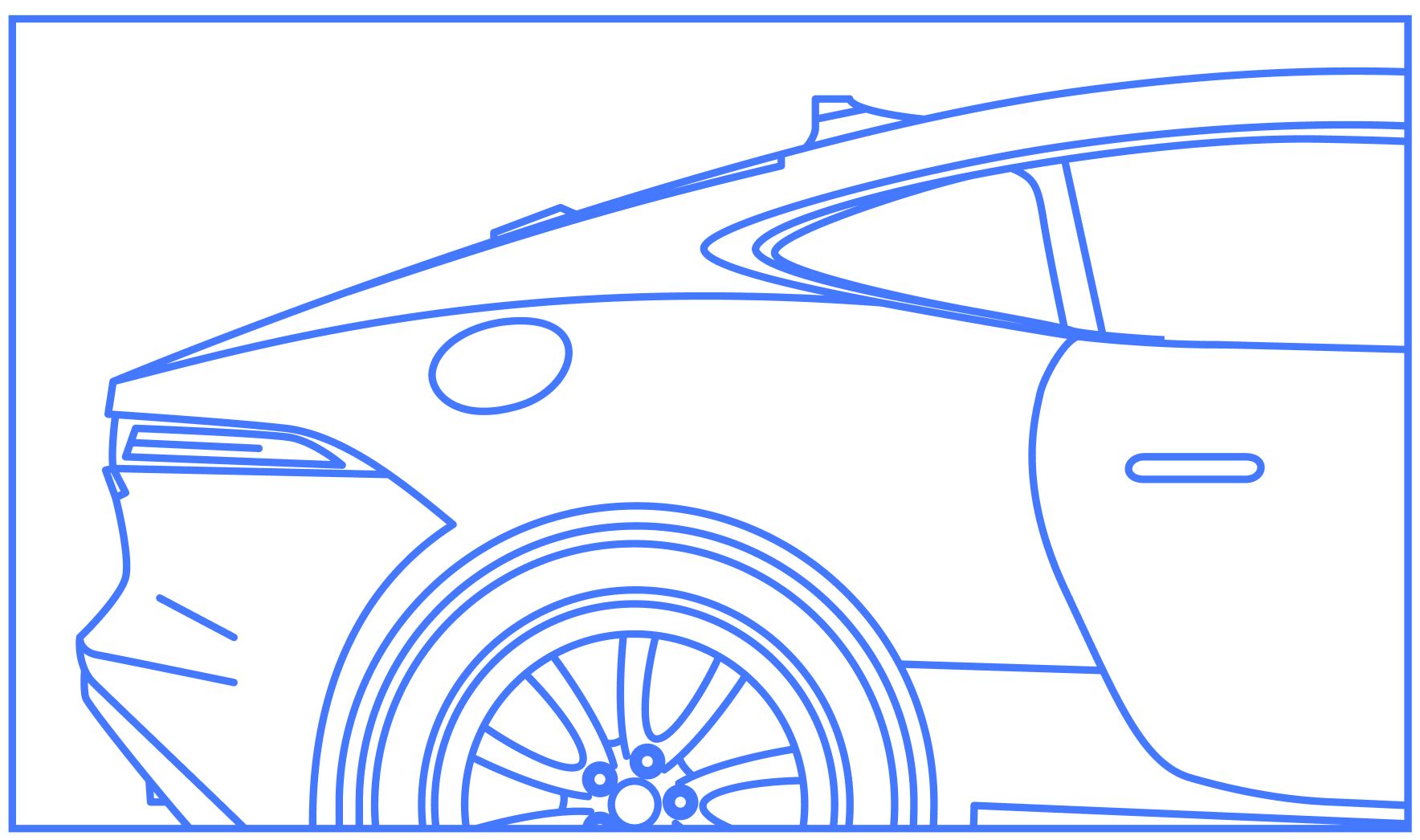

After evaluating the performance of Neural Network models on benchmark test cases (see Fig.1), PSA decided to push the collaboration further towards a real-time predictive model for external aerodynamics, applicable to production-level 3D simulations.
With this new step, PSA will aim at accelerating design cycles, the time between the ideation of a new design and the start of production. Another target is to optimize the performance of the next generations of vehicles, including larger autonomy and greater passenger comfort.
The benchmark study compared Geometric CNNs to Gaussian-Process based regression models, specifically tuned for production-level simulations.
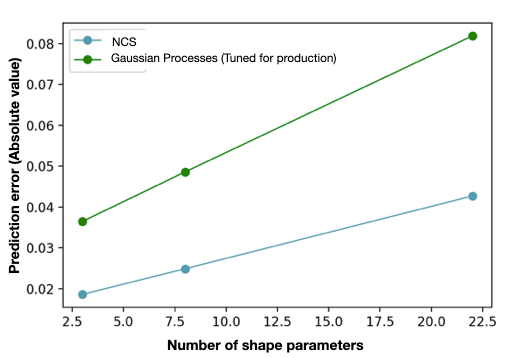
It proved that, even though the Geometric CNN does not have access to the parametric description and is therefore much more broadly applicable than the Gaussian Process, it can also outperform the standard methods by a large margin.
Neural Concept Shape is a high-end deep learning software, which understands 3D shapes (CAD), and learns how they interact with the laws of physics (CAE). It is able to emulate full-fledged simulators, giving predictions in approximately 30 ms versus minutes to hours (or even days) for classic simulators. In other terms, engineers can use Neural Concept Shape to explore, manually or automatically, an infinite amount of designs without calling back the resource-consuming, time-consuming simulator.
NCS is the link between designers and simulation experts in the company, reducing lengthy iterations between teams. This allows to dramatically accelerate R&D cycles, enhance product performances, and solve the most difficult engineering challenges.

































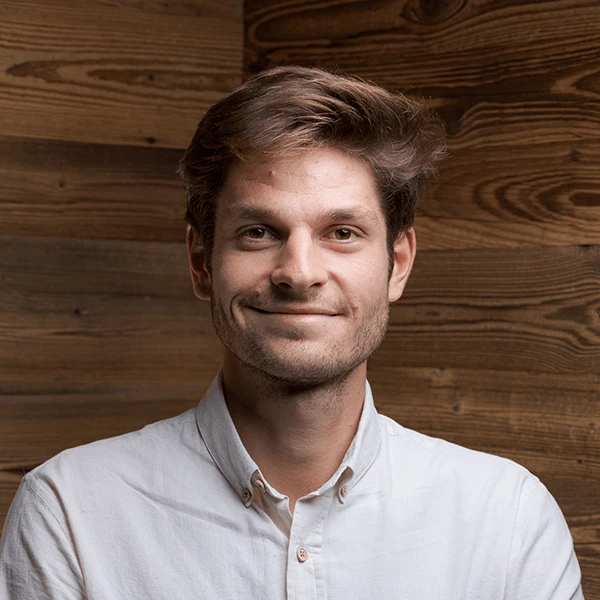

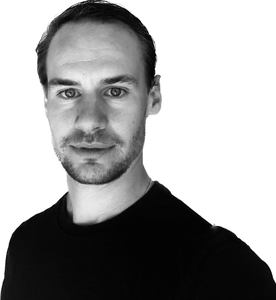
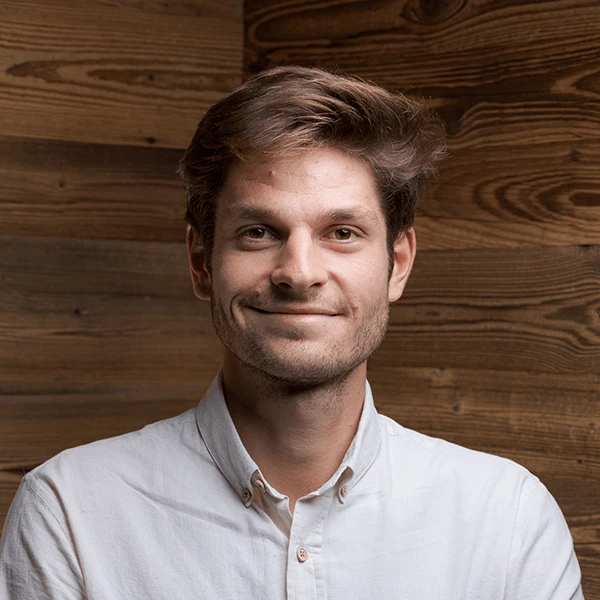










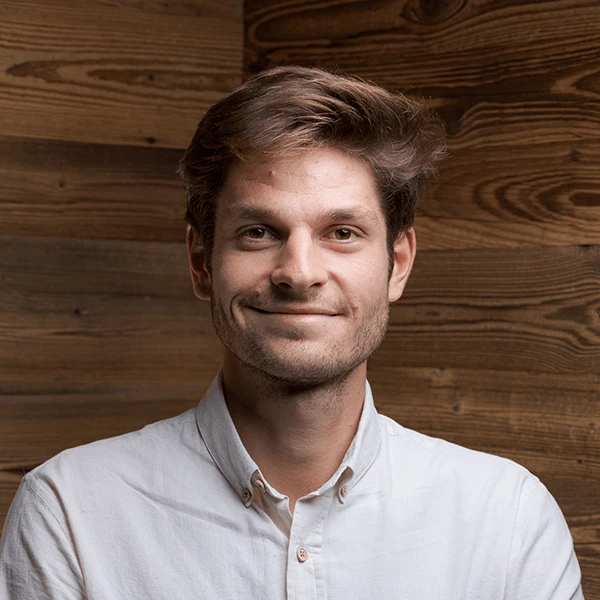














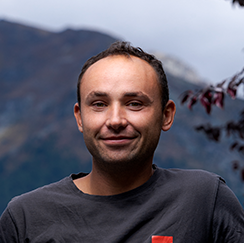
