Neural Concept presents ‘Enabling Uncertainty Estimation in Iterative Neural Networks’ technical paper at ICML

- Neural Concept has published a new technical paper that explores use of convergence rates as a proxy for uncertainty estimation in deep learning networks.
- The paper demonstrates the potential of iterative neural networks and reveals an approach to uncertainty estimation that provides state-of-the-art estimates at a much lower computational cost than more established techniques.
- The paper will be presented at the International Conference on Machine Learning (ICML) at the Messe Wien Exhibition Congress Center, Vienna, Austria on Thursday, 25 July from 1:30 - 3:00 (CEST).
Challenges paper seeks solution to
As engineers and product teams increasingly look to optimise and accelerate the development process, deep learning models have been leveraged to speed up the prediction and refinement process.
Deep Ensembles have become established means of doing this, and are noted for the potential diversity and accuracy of their predictions. This diversity is a key part of their effectiveness in uncertainty estimation, and the accuracy has contributed to their widespread usage by engineers and product teams across industries.
However, the severe drawback of Ensembles is their high computational cost, and as deep learning becomes increasingly embedded into the engineering workflow, a solution is needed which brings these costs down without compromising on speed or accuracy.
Using convergence rates to deliver high quality estimates at lower costs
In order to find a way around this obstacle, Neural Concept demonstrates a new approach to uncertainty estimation by using the convergence rates of iterative network outputs to deliver state-of-the-art estimates at much lower computational costs.
This is achieved by creating a virtuous circle in which the model receives relevant spatial attention signals that serve as priors and help generate improved predictions at a much faster rate. This can be applied to improve the predictive models used in design and engineering today. With higher accuracy and lower costs, enterprises can bring better products to market faster, and with potentially higher margins.
Method/implementation & key results
In this technical paper, Neural Concept demonstrates the value of this approach by embedding it in two application domains: road detection in aerial images and the estimation of aerodynamic properties of 2D and 3D shapes.
• The report introduces an effective method for estimating uncertainty in iterative models. It relies on consistency across consecutive predictions and does not require modifying the network architectures.
• Through extensive experiments and analyses, this report demonstrates the effectiveness of this new proposed method of uncertainty estimation. In particular, it shows that in going from a toy example to two complex real-world scenarios, there remains a consistent correlation between convergence speed and prediction accuracy.
• The method, once embedded in a Bayesian Optimisation framework, delivers state-of-the-art accuracy and predictive uncertainty quantification in both road detection from aerial images and 2D/3D shape optimisation.
This workflow produced delineation accuracy and delineation uncertainty quality that significantly outperformed other approaches, including Deep Ensembles, U-Net, and MC-Dropout. The accuracy and computational cost optimisation of this method are, therefore, beyond the capabilities of the current established methods of uncertainty estimation.
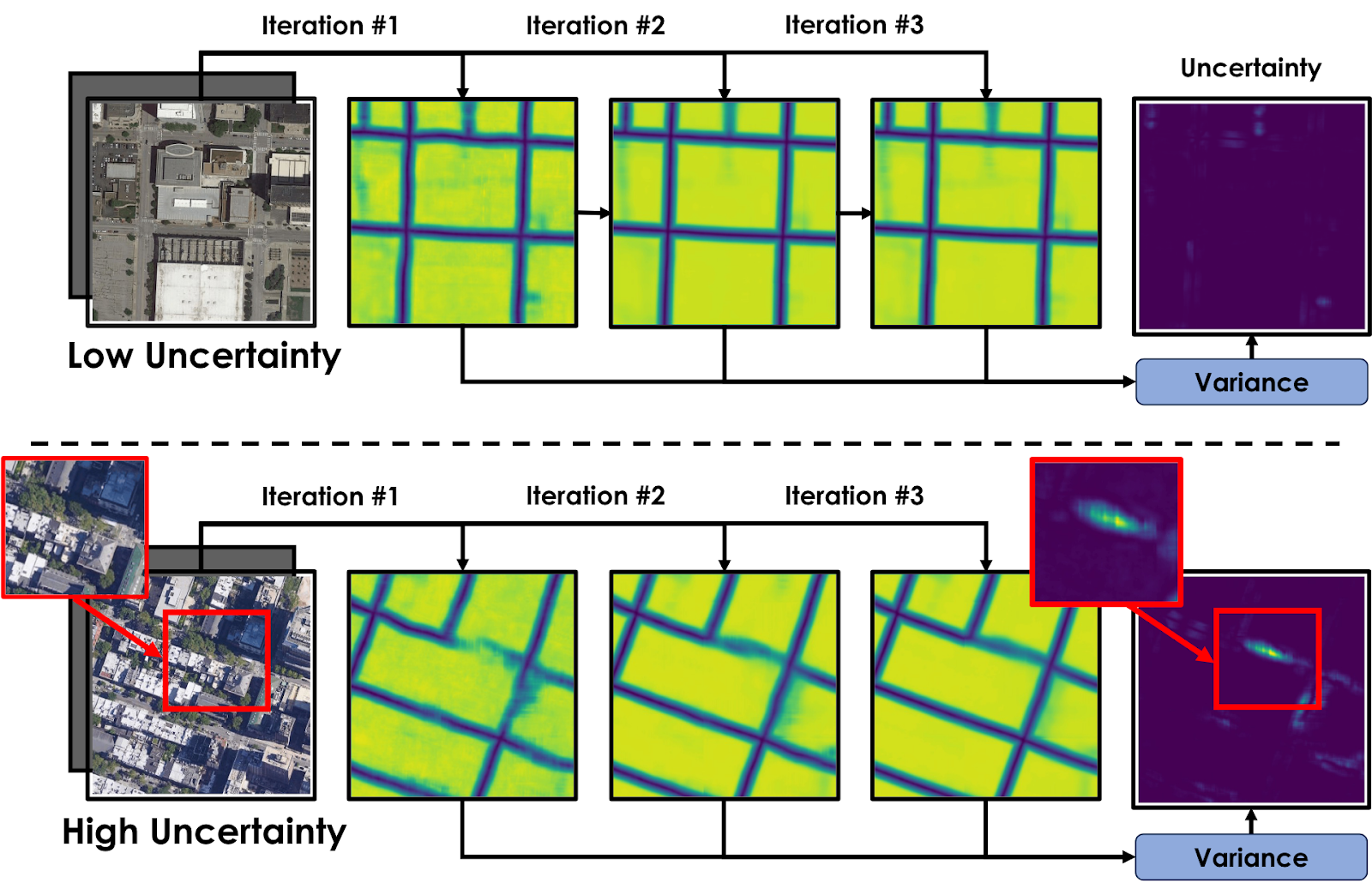
What Does This Mean?
For Engineers and Product Teams: Faster and more accurate predictions without the hefty computational costs, leading to more efficient workflows and quicker iteration cycles.
For OEM’s: Reduced expenses on computational resources, enabling better allocation of budgets and potentially higher profit margins.
For the AI Industry: This breakthrough can drive advancements in various applications, from improving road safety through better detection technologies to enhancing the performance of 2D and 3D shapes in design and engineering.
Conclusion
This novel approach to iterative networks demonstrates a cheaper method of assessing prediction quality than Deep Ensembles. It is also shown to be much more reliable than other state-of-the-art methods.
Given that Deep Ensembles are currently the most reliable approach to such assessments, this presents a big opportunity for cost optimisation within the field of product development for road detection and aerodynamic properties. These are the fields that the report focuses on, but the principles underpinning the experiment apply to many other devices ranging from the cooling plates of an electric vehicle battery to the optics of an image acquisition device.
Neural Concept will explore a broader set of potential applications in future work. However, this work shows how the boundaries of iterative neural networks can be expanded and enterprises can leverage state-of-the-art uncertainty estimation at a much lower computational cost.