NCS on Hydrodynamic Applications
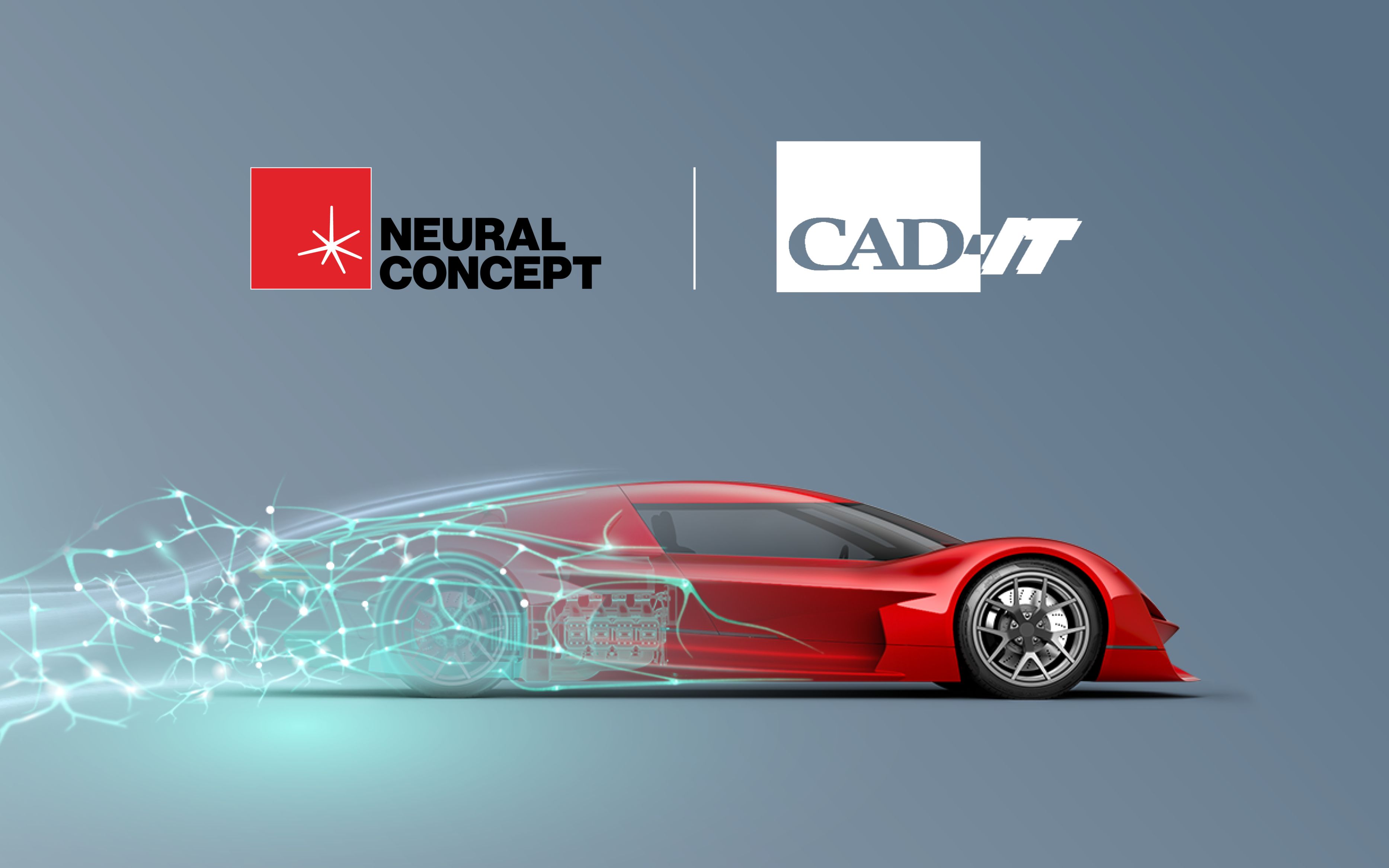
Marine engineering is a complex blend of traditional design best practices with modern knowledge. Naval architects can nowadays carefully design hull shapes based on previous examples and the science of hydrodynamics, leveraging modern simulation software, combined and validated with experimental results. Accuracy is not an option for the naval engineer: slight modifications in the geometry can have a drastic impact on the overall hydrodynamic performance of the vessel!
To evaluate the vessel's hydrodynamic resistance and various performance indices such as sea-keeping and green water, engineers are converging towards a simulation-driven design approach, but some major bottlenecks remain. CFD simulations are time-consuming and expensive, and the design space exploration remains limited to just a few parameters.
Neural Concept Shape (NC Shape), leveraging Geometric Deep Learning models, allows to overcome the above limitations and to radically improve the design process of organizations, also in the marine sector.
In this use case, we show how one of our partners, CAD-IT Korea, within the first month of using Neural Concept Shape, could build a NC Shape model which predicts the hydrodynamic performances of boat hulls, directly from the CAD geometry, within a few ms.
The model was trained using 150 different geometries, directly from the CAD format, together with the corresponding STAR-CCM+ simulations. These hull geometries correspond to typical variations that can be expected for a standard tanker ship. The aim of the training process was to learn how to predict the pressure distribution on the hull, to prevent any undesired flow behavior; also, Deep Learning process involved the prediction of overall performance parameters of the hull: the shear force coefficient, and the pressure force coefficient.
After the training phase, the Deep Learning model was put in production. The results on new, unseen shapes, are given below:


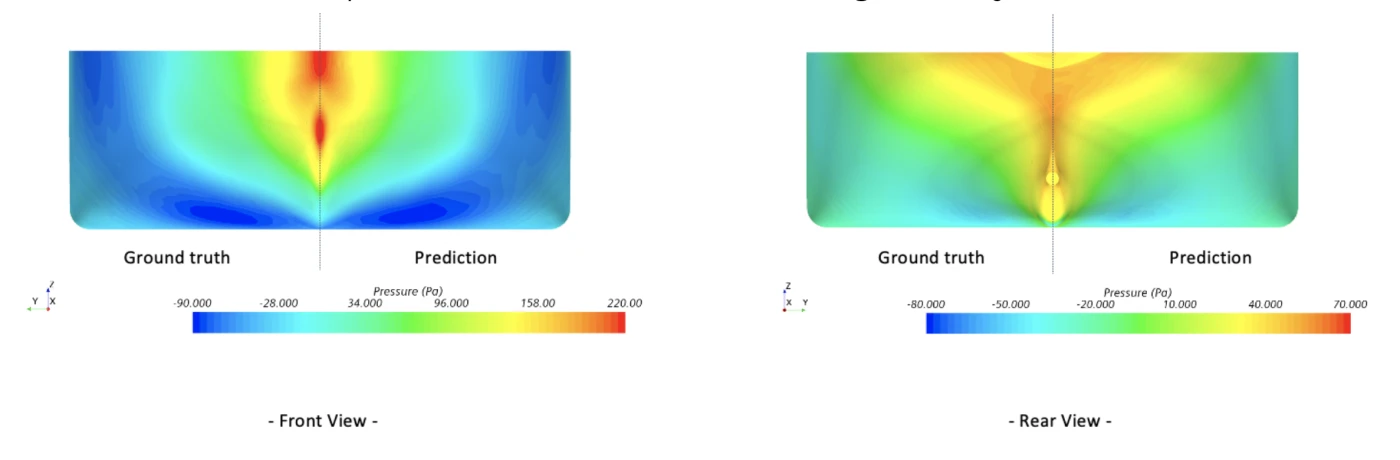