Innovations in Turbulence Modeling: Enhancing Simulation

Turbulent flows are complex phenomena encountered in various fields of science and engineering. They exhibit chaotic motion and are characterized by irregular velocity fluctuations and rapid changes in pressure.
Understanding and predicting turbulent flows is important for designing efficiently and optimizing the performance of systems ranging from motorsport competitions to optimizing truck aerodynamics.
Computational Fluid Dynamics (CFD) plays a vital role in simulating and analyzing turbulent flows, enabling engineers and researchers to gain valuable insights into the behaviour of fluids. This article aims to provide a comprehensive overview of turbulent flows, their modelling techniques and advanced turbulence models employed in CFD.
We will discuss the "classic" CFD applications and the innovations introduced by data-driven simulation.
Fundamentals of Turbulence — Defining Turbulent Flows
Turbulence refers to the irregular and chaotic motion exhibited by fluid flows. Turbulent flows are characterized by a high degree of velocity fluctuations and rapid changes in pressure, resulting in enhanced mixing and increased transport of momentum and energy.
In many practical situations, such as the flow of air over an airplane wing or water flowing through a pipe, turbulence is present even under steady flow conditions. In these cases, the flow is turbulent, but it maintains a relatively constant average behavior over time.
However, there are also situations where the flow is both turbulent and unsteady. This can occur when external factors or flow conditions change, leading to fluctuations in the flow properties. Examples include the turbulent wake behind a moving vehicle or the fluctuations in a river flow due to changing water levels.
The Reynolds Decomposition
Reynolds decomposition is a technique used in fluid dynamics to analyze and understand turbulent flows. It involves decomposing the variables describing the flow into a mean component and a fluctuating component. This decomposition allows us to separate the average behavior of the flow from the turbulent fluctuations.
Mathematically, the Reynolds decomposition of a flow variable, such as velocity (u), pressure (p), or any other quantity, can be expressed as:
Variable = Mean + Fluctuation
For example, the decomposition for velocity (u) would be:
u = U + u'
where:
- u is the instantaneous velocity at a given point in the flow.
- U is the mean velocity, which represents the average velocity over time or space.
- u' is the fluctuating velocity component, representing the turbulent fluctuations around the mean velocity.
By decomposing variables in this way, we can analyze the flow as a combination of the mean behavior and the turbulent fluctuations. The mean component represents the large-scale, steady behavior of the flow, while the fluctuating component captures the smaller-scale, unsteady turbulent motions.
The relationship between Reynolds decomposition and the previous discussion is that the decomposition helps us understand and quantify the turbulent nature of flows. By separating the flow into mean and fluctuating components, we can study the statistical properties of the turbulent fluctuations, such as their intensity, spatial distribution, and temporal behavior.
Learn more about Leonardo da Vinci as forerunner of this concept in the Appendix.
Turbulence Model Principles
Fluid Flow and Turbulent Motion
Fluid flow, both internal and external, is influenced by turbulent phenomena. The intricate interplay between turbulent motion and fluid flow phenomena, such as boundary layer and adverse gradients of pressure, significantly impacts the overall performance of systems. Understanding these interactions is essential for accurate modelling and analysis.
Turbulent Kinetic Energy and Reynolds Stress
Turbulent kinetic energy (TKE) represents the energy associated with velocity fluctuations in a turbulent flow. It plays a crucial role in quantifying the intensity of turbulent phenomena and provides insights into the transfer of energy across different scales within turbulent fluctuations. On the other hand, Reynolds stress arises due to the correlation between velocity fluctuations and is a measure of the momentum transfer within turbulent flows.
What Is CFD? Introduction
CFD is a powerful computational tool used to analyze and simulate fluid flow phenomena in various applications. By employing numerical methods and mathematical models, CFD enables engineers and scientists to understand fluid behaviour, investigate complex flow patterns, and optimize designs for improved performance. This introductory chapter provides an overview of CFD, its underlying principles, and its significance in various fields.
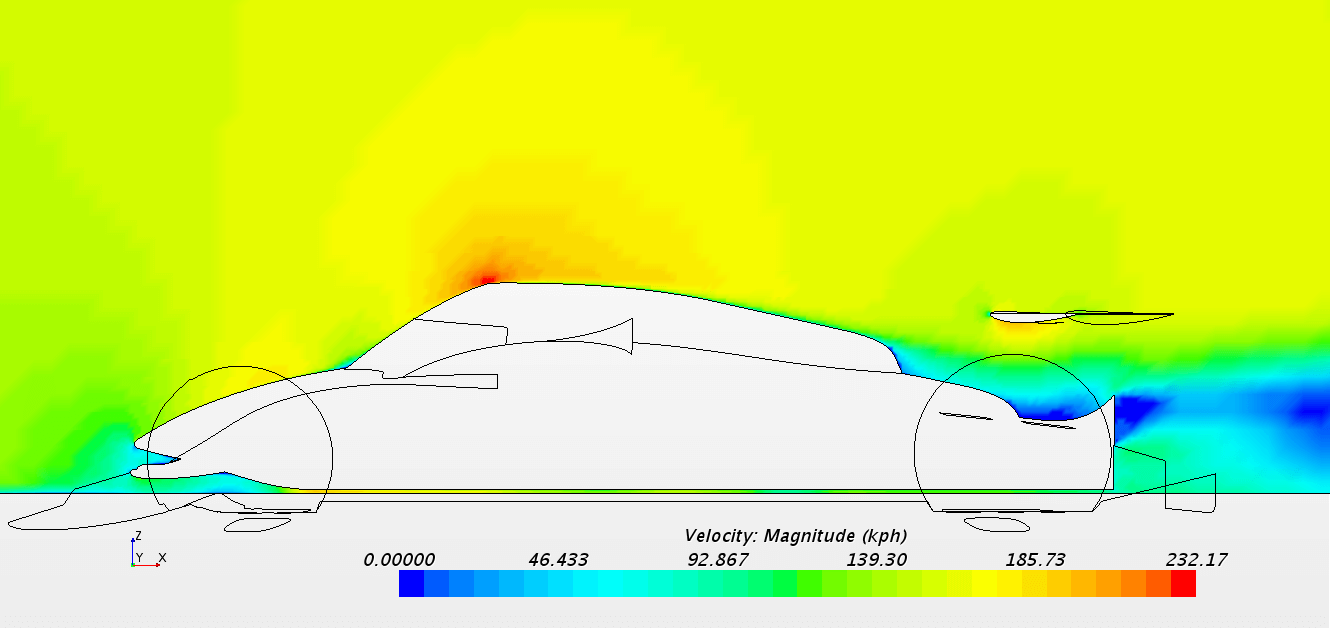
Understanding Fluid Dynamics
Fluid dynamics is the branch of physics that studies the motion and behaviour of fluids, which include liquids and gases. It encompasses a wide range of phenomena, from the flow of air around an aircraft wing to the movement of blood within our bodies. Fluid dynamics principles govern the behaviour of fluid flow, including characteristics such as velocity, pressure, and turbulence.
The Role of CFD in Engineering
CFD has become an indispensable tool in various engineering and scientific disciplines. It enables engineers to optimize designs, assess performance, and predict the behaviour of fluid flow systems. In fields such as aerospace, automotive, energy, and environmental engineering, CFD plays a crucial role in improving efficiency, safety, and sustainability.
CFD Fundamentals
At the core of CFD lies the discretization of fluid flow equations and the numerical solution of these equations on a computational grid. The governing equations, such as the Navier-Stokes equations, are solved iteratively to obtain approximate solutions to momentum equations that describe the flow. CFD incorporates turbulence modelling techniques to accurately simulate real-world scenarios, which capture the effects of turbulent flow and TKE fluctuations.
CFD Workflow and Process
The CFD workflow involves several steps, including problem formulation, geometry creation or import, grid generation, selection of appropriate boundary conditions, solver setup, and post-processing of results. Each step requires careful consideration and expertise to ensure accurate and reliable simulations.
Applications of CFD
CFD finds applications in a wide range of fields. In the aerospace industry, CFD is used to optimize aircraft designs, analyze aerodynamic performance, and assess the impact of airflow on structural components. In automotive engineering, CFD enhances vehicle aerodynamics, reduces drag, and improves fuel efficiency. CFD also plays a crucial role in environmental modelling, HVAC systems design, biomedical engineering, and many other disciplines where fluid flow is a significant factor.
Advancements and Future Perspectives
CFD continues to advance with the development of more accurate turbulence models, improved numerical algorithms, and increased computational power. The emergence of high-performance computing and cloud-based simulations allows for faster and more detailed analysis of complex flow problems. Additionally, integrating CFD with optimization algorithms and machine learning techniques holds the promise of further enhancing design optimization and decision-making processes.
Summary of CFD Simulation
CFD is a branch of fluid dynamics that focuses on the 3D engineering simulation and analysis of fluid flow. It combines the principles of fluid mechanics, applied mathematics, and computer science to solve the governing equations that describe fluid motion. CFD allows for a comprehensive analysis of complex fluid flow phenomena that may be difficult or impossible to study experimentally.
Turbulence Modeling: An Overview
A turbulence model aims to describe and predict the behaviour of turbulent flows. Various turbulence modelling techniques have been developed to simplify the complexities associated with turbulence. These models employ mathematical equations to approximate the turbulent flow field and capture essential characteristics.
Reynolds-Averaged Navier-Stokes (RANS) Equations
The RANS equations form the basis of many turbulence models used in CFD. RANS equations provide a time-averaged representation of turbulent flows by averaging the governing equations of fluid flow over time.
This approach enables computational efficiency, but it also introduces challenges due to the closure problem.
Eddy Viscosity Turbulence Model
These models are a subclass of RANS models and assume an effective molecular viscosity for turbulent flows. These models utilize an eddy viscosity term to account for the impact of turbulence on turbulent viscosity and flow dynamics. The eddy viscosity is related to the local flow properties and is crucial for accurately predicting the turbulence effects.
The k-ε Turbulence Model
The k-ε turbulence model is the most widely employed eddy viscosity model. It represents the TKE (k) and its dissipation rate (ε). By solving additional equations for these quantities, the k-ε model provides insights into the turbulent flow characteristics, particularly in isotropic turbulence and internal flows.
The k-omega Turbulence Model
The k-omega turbulence model is another two-equation turbulence model and the most popular eddy viscosity model that addresses some of the limitations of the k-ε turbulence model. It incorporates an additional transport equation for the specific dissipation rate (ω) to improve the predictions of near-wall flows and adverse pressure gradients. The k-ω model has gained significant attention for simulating complex flows in such cases.
Advanced Turbulence Models
Large Eddy Simulations (LES)
As turbulent flows exhibit a wide range of length scales, resolving flow variables at all scales in a simulation is computationally expensive. Large Eddy Simulation (LES) is an advanced turbulence model that aims to resolve large-scale turbulent structures while modelling smaller scales. The LES turbulence model provides detailed insights into the complex flow features, such as vortex shedding and separation.

Turbulence Transport Equations
Turbulence transport equations govern the evolution of turbulent flow quantities, such as TKE and Reynolds stress. Solving these equations within turbulence models is crucial for accurately capturing the dynamics of turbulent flows. The equations allow for the estimation of turbulent quantities at each point in the computational domain.
Advancements in Turbulence Models
To overcome the limitations of traditional turbulence models, researchers have developed advanced turbulence models that offer improved accuracy and predictive capabilities. These models, such as the Reynolds Stress Model (RSM) and the Scale-Adaptive Simulation (SAS) model, address specific flow scenarios and aim to capture the intricate flow physics more accurately.
Large Eddy Simulation Turbulence Model (LES)
The LES turbulence model incorporates advanced subgrid-scale modelling techniques to capture the effects of unresolved turbulent structures. Large eddy simulation models enable a more detailed representation of turbulence and provide accurate predictions of flow features such as turbulence intensity and spatial distribution of velocity fluctuations.
Direct Numerical Simulation (DNS)
Direct Numerical Simulation (DNS) is a technique that aims to simulate turbulence by explicitly resolving all the scales of motion in a flow. DNS provides highly accurate solutions but is computationally demanding, limiting its application to relatively low Reynolds number flows.
Velocity Fluctuations and Reynolds Stress in DNS
In DNS, the resolved velocity fluctuations provide detailed information about the turbulence intensity and turbulent structures of separated flows. The Reynolds stress, which quantifies the momentum transfer within the flow, can be directly computed from DNS simulations, allowing for precise analysis and validation of turbulence models.
Applications and Limitations of DNS
DNS has found applications in fundamental research, serving as a benchmark for validating turbulence models and providing insights into the underlying flow physics. However, the computational cost associated with DNS restricts its usage to relatively small domains and low Reynolds number flows.
Applications of Turbulence Modeling
Turbulence Modeling in Internal Flows
Turbulence modelling is crucial in analyzing and optimizing internal flows, such as those occurring in pipes, ducts, and channels. Understanding the characteristics of boundary layers, adverse pressure gradients, and turbulence intensity is vital for designing efficient systems in various industries.
Turbulence Modeling in a Boundary Layer
The Boundary Layer, as a region near solid surfaces, is greatly influenced by turbulence. Accurate turbulence modelling in a boundary layer is essential for predicting skin friction, heat transfer, and separation, all of which significantly impact the performance of aircraft, vehicles, and industrial equipment.
Turbulence Modeling in Complex Flows
Complex flows, including those with swirling motion, vortices, and recirculation zones, require advanced turbulence models to capture their intricate behaviour. Turbulence modelling in these scenarios helps optimize the design and operation of systems such as turbomachinery, combustion chambers, and environmental flows.
Turbulence Modeling with Adverse Pressure Gradient
Turbulence models are essential in analyzing adverse pressure gradient situations where the flow undergoes significant changes in pressure along its path. Accurate predictions of turbulence effects in adverse pressure gradients are crucial for optimizing the performance of aircraft wings, turbine blades, and other flow control devices.
Use-Case: Aerodynamics Analysis
The analysis of aerodynamics heavily relies on turbulent kinetic energy, computational fluid dynamics, and turbulence transport equations. These techniques allow engineers to gain an understanding of intricate flow behavior, making precise predictions regarding aerodynamic performance, and optimizing design for improved efficiency and safety. Thanks to these advancements, the field of aerodynamics is constantly evolving and driving innovation.

Introduction to Aerodynamics Analysis
Aerodynamics is pivotal in designing efficient aircraft, vehicles, and various engineering structures. Understanding the complex flow behaviour, including turbulence, is crucial for optimizing performance and ensuring safety. In this chapter, we explore the application of turbulent kinetic energy, CFD, and turbulence equations in aerodynamics analysis.
Turbulent Kinetic Energy
Turbulent kinetic energy (TKE) is a key parameter in aerodynamics analysis, as it quantifies the intensity of turbulence in a flow field. By considering the TKE distribution, engineers can evaluate the turbulence levels and their impact on aerodynamic performance. Utilizing CFD, the TKE values can be accurately predicted and analyzed.
Turbulence Transport Equations
Turbulence equations are essential in aerodynamics analysis as they govern the evolution of turbulent flow quantities. The equations for TKE, Reynolds stress, and dissipation rate play a crucial role in modelling and predicting turbulent flow effects. By solving these equations within CFD, engineers can gain insights into the behaviour of turbulence and its impact on aerodynamic performance.
Eddy Viscosity Models
Eddy viscosity models are widely used in aerodynamics analysis to approximate the effects of turbulence. These models, including the popular k-ε and k-ω models, incorporate an effective viscosity term to account for the turbulent flow characteristics. Engineers can accurately capture turbulent flow effects by incorporating those models into CFD simulations.
Large Eddy Simulations
Large Eddy Simulation (LES) is an advanced turbulence modelling technique used in aerodynamics analysis. By resolving the large-scale turbulent structures and modelling the smaller scales, LES provides detailed insights into complex flow features such as separation, vortex shedding, and wake dynamics. LES simulations combined with CFD allow for a comprehensive analysis of aerodynamic performance.
Averaged Navier Stokes (RANS)
Averaged Navier Stokes (RANS) equations, a class of turbulence models, are commonly employed in aerodynamics analysis. RANS-based models provide a time-averaged representation of mean flow characteristics of turbulent flows, allowing for efficient computations. By solving the RANS equations and turbulence closure models, engineers can accurately predict aerodynamic forces, lift, drag, and other flow characteristics.
Aerodynamics Analysis: Case Studies
To illustrate the practical application of turbulent kinetic energy, computational fluid dynamics, and turbulence equations in aerodynamics analysis, we present two case studies:
- Case Study 1: Aircraft Wing Design Optimization Using CFD. With CFD and turbulence models, engineers analyze the airflow over an aircraft wing to optimize lift, minimize drag, and enhance fuel efficiency. The turbulent kinetic energy is evaluated to assess the intensity of turbulence, and the computed flow patterns provide insights into pressure distribution and airflow separation.
- Case Study 2: Automotive Aerodynamics Analysis. The automotive industry uses CFD coupled with turbulence models to improve vehicle aerodynamics. By analyzing the turbulent kinetic energy distribution and turbulence equations, engineers can refine the vehicle shape, reduce drag forces, and enhance vehicle stability and fuel economy.
Case Study 1: Aircraft Wing Design Optimization
Aircraft wing design plays a critical role in achieving optimal aerodynamic performance. Engineers utilize CFD and turbulence models to analyze the airflow over an aircraft wing, aiming to optimize lift, minimize drag, and enhance fuel efficiency. This case study demonstrates how turbulent kinetic energy, CFD, and turbulence transport equations contribute to the design optimization process.
Problem Formulation
The first step in aircraft wing design optimization is to define the problem and the desired objectives. The engineers set specific goals, such as maximizing lift while minimizing drag and reducing fuel consumption. These objectives guide the subsequent analysis and inform the selection of appropriate turbulence models and computational techniques.
CFD Application
CFD is employed to model the airflow around the aircraft wing numerically. The three-dimensional geometry of the wing is discretized into a computational grid, and the governing equations of fluid flow, such as the Navier-Stokes equations, are solved iteratively. To accurately capture the effects of turbulence, turbulence models, such as the k-ε or k-omega models, are incorporated into the simulations.
Turbulent Kinetic Energy Evaluation
Turbulent kinetic energy (TKE) is a key parameter in assessing the intensity of turbulence in the flow field. Engineers evaluate the TKE distribution across the wing surface and in the surrounding flow to identify regions of high turbulence intensity. This information helps in understanding the impact of turbulence on the overall aerodynamic performance and provides insights into flow separation and pressure distribution.
Pressure Distribution Analysis
The computed flow patterns obtained from the CFD simulations provide valuable information about the pressure distribution over the wing surface. Engineers analyze the pressure contours to identify areas of high and low pressure, which directly influence lift and drag forces. By optimizing the pressure distribution, engineers can enhance lift generation and reduce drag, thereby improving the overall efficiency of the wing.
Flow Separation Investigation
Separation occurs when the airflow fails to follow the contour of the wing surface, leading to adverse effects on lift and increased drag. CFD coupled with turbulence models allows engineers to identify regions prone to separation. By studying the flow separation behaviour and its correlation with turbulent kinetic energy, engineers can design wing geometries and employ flow control devices to mitigate separation and improve overall aerodynamic performance.
Case Study 2: Automotive Aerodynamics Analysis
The automotive industry heavily relies on aerodynamic design to enhance vehicle performance, stability, and fuel efficiency. In this case study, computational fluid dynamics simulations, coupled with turbulence models, are utilized to improve automotive aerodynamics. The analysis focuses on reducing drag forces, refining the vehicle shape, and enhancing stability and fuel economy.
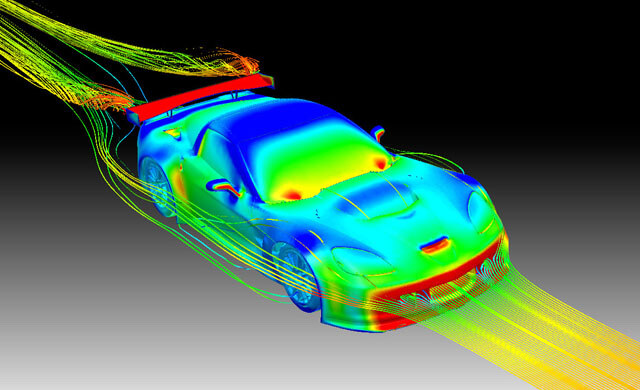
Problem Formulation
The case study begins by defining the problem and objectives that are specific to automotive aerodynamics.
Engineers aim to reduce aerodynamic drag, significantly reducing fuel consumption and limiting vehicle speed. Additionally, they seek to improve stability and minimize unwanted aerodynamic effects, such as lift and side forces. These objectives guide the subsequent analysis and inform the choice of turbulence models and simulation methodologies.
Computational Fluid Dynamics Simulations
CFD is employed to model turbulence quantities in the airflow around the vehicle geometry. The three-dimensional vehicle shape is discretized into a computational grid, and the governing fluid flow equations, including the Navier-Stokes equations, are solved numerically. To accurately capture turbulence effects, appropriate turbulence models, such as the Reynolds-Averaged Navier-Stokes
AI Case Study: Addressing the Challenges in Turbulence (HiFi-TURB Project)
In applied fluid mechanics, a major hurdle faced by engineers and scientists is the limited understanding and predictive capabilities of turbulence-dependent features. This poses a significant challenge, leading to a lack of confidence in using Computational Fluid Dynamics (CFD) for various aeronautical applications, such as airflow detachment over aircraft wings or interactions between shock waves and boundary layers. To tackle these issues, the HiFi-TURB project, spearheaded by NUMECA International, aims to develop innovative solutions and address the deficiencies in the modelling of turbulent flows.
The advent of High-Performance Computing (HPC) has opened up new possibilities for advancing turbulence model development. The HiFi-TURB project put together Artificial Intelligence and Machine Learning (ML) techniques.
These techniques are applied to a comprehensive database comprising high-fidelity, scale-resolving simulations of test cases that encompass the complex features of separated flow regions and intricate 3D flows.
As shown the image below, these simulations provide the foundation for improving turbulence models.

Managing and extracting valuable insights from the enormous volume of data generated by these simulations necessitates a new approach to data mining. Neural Concept, a partner in the project, brings its expertise in Deep Learning to the table, offering a toolchain specifically designed for the analysis of vast amounts of data derived from 3D scale-resolving simulations.
Using Neural Concept's Geometry-based Variational Auto-Encoders (VAE), NUMECA has gained insights into the correlations between numerous statistically averaged variables. The VAE technique first compresses the data into physically meaningful representations, referred to as "embeddings," and then accurately reconstructs the original input from the compressed data.
This process allows for using the ML model as a surrogate for the original data, simplifying data handling while enabling the application of data mining and analysis techniques to gain a deeper understanding of the underlying physics.

Figure above illustrates one example of the potential analysis made possible through this approach. These plots offer a fresh perspective on the behaviour of flows through the lens of the machine learning model.
Through the HiFi-TURB project, the fusion of AI, ML, and high-fidelity simulations paves the way for more accurate and reliable predictions in aeronautical applications, ultimately enhancing the efficiency and safety of aircraft design and operation.
Conclusions — Summary
Together, we explored the innovations in turbulence modeling and their impact on simulation, specifically in the context of CFD. Turbulent flows, characterized by irregular velocity fluctuations and rapid pressure changes, are complex phenomena found in various fields, including motorsport and aerodynamics. CFD enables engineers and researchers to simulate and analyze turbulent flows, providing valuable insights into fluid behavior.
We covered the fundamentals of turbulence modeling principles such as turbulent kinetic energy and Reynolds stress, and the interaction between fluid flow and turbulent motion. It provides an introduction to CFD and its significance in engineering and science, explaining the governing equations and the role of turbulence modeling techniques in capturing turbulent effects accurately.
Various models were discussed, including the Reynolds-Averaged Navier-Stokes (RANS) equations, the k-ε and k-ω models, and advanced models such as Large Eddy Simulation (LES) and DNS. The application of turbulence modeling in various types of flows was also explored.
We concluded with case studies illustrating the practical application of CFD models in aerodynamics analysis, focusing on aircraft wing design optimization and automotive aerodynamics analysis.
Also, we have seen with the HiFi-TURB project example the fusion of AI, ML, and high-fidelity simulations operning up new possibilities for improving the modelling physics for engineers.
Appendix: Leonardo Da Vinci and Reynolds Decomposition
Leonardo da Vinci is known for his iconic artwork, but many people are unaware he was also a prolific inventor and engineer. In his notebooks, Leonardo documented his observations and theories about various topics, including fluid dynamics and the nature of turbulence.
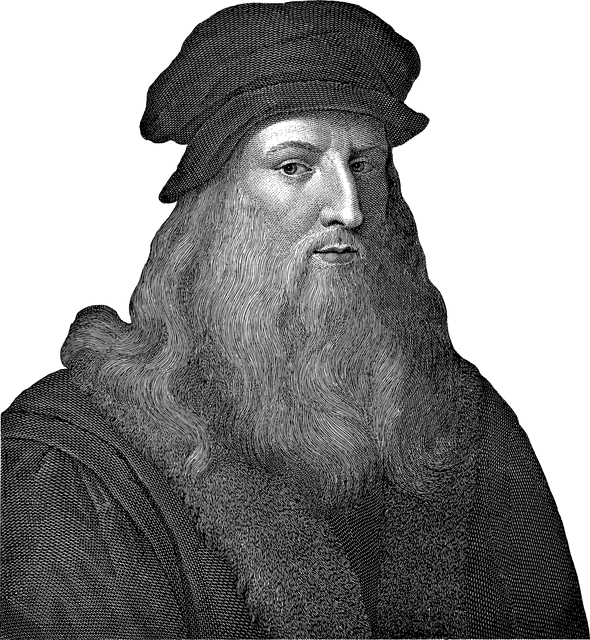
Even if Leonardo was no mathematician, his deep insights laid the foundation for the modern understanding of these phenomena, including the concept of decomposing velocity u into U and u' (read "u prime"), now a cornerstone of turbulence modelling in the Reynolds decomposition.
Turbulence modelling is about complex and chaotic phenomenon in fluids when the flow becomes irregular and unpredictable. It can be observed in many natural and man-made systems, from airflow over a wing to the motion of water in a river or stream.
Leonardo was one of the first people to recognize the existence of turbulence, and he made detailed observations of its behavior. He noted that when water flowed over an obstacle, such as a rock or a tree, it would create eddies and whirlpools that persisted long after the flow had passed. He also observed that the behavior of the fluid was not repeatable, even under identical conditions, suggesting that turbulence was an inherently random and chaotic process.

One of Leonardo's most significant contributions to the study of turbulence was his recognition of the concept of U and u'. In his notebooks, he described the motion of fluid particles as consisting of two components: a uniform, steady flow in one direction (U), and a fluctuating, irregular motion perpendicular to the flow (u'). This concept is now known as Reynolds decomposition, named after the British scientist Osborne Reynolds, who developed it in the 19th century.
The concept of U and u' is fundamental to modern fluid mechanics and forms the basis of many models used in CFD, the Navier-Stokes equations are often decomposed into a steady component (U) and a fluctuating component (u'). This allows researchers to model turbulence by simulating the behaviour of the fluctuating component, which is more challenging to study experimentally. Leonardo's work was groundbreaking, but it was not until many centuries later that his insights were fully appreciated..