Improving Crash Box Performance by 10% with DLR
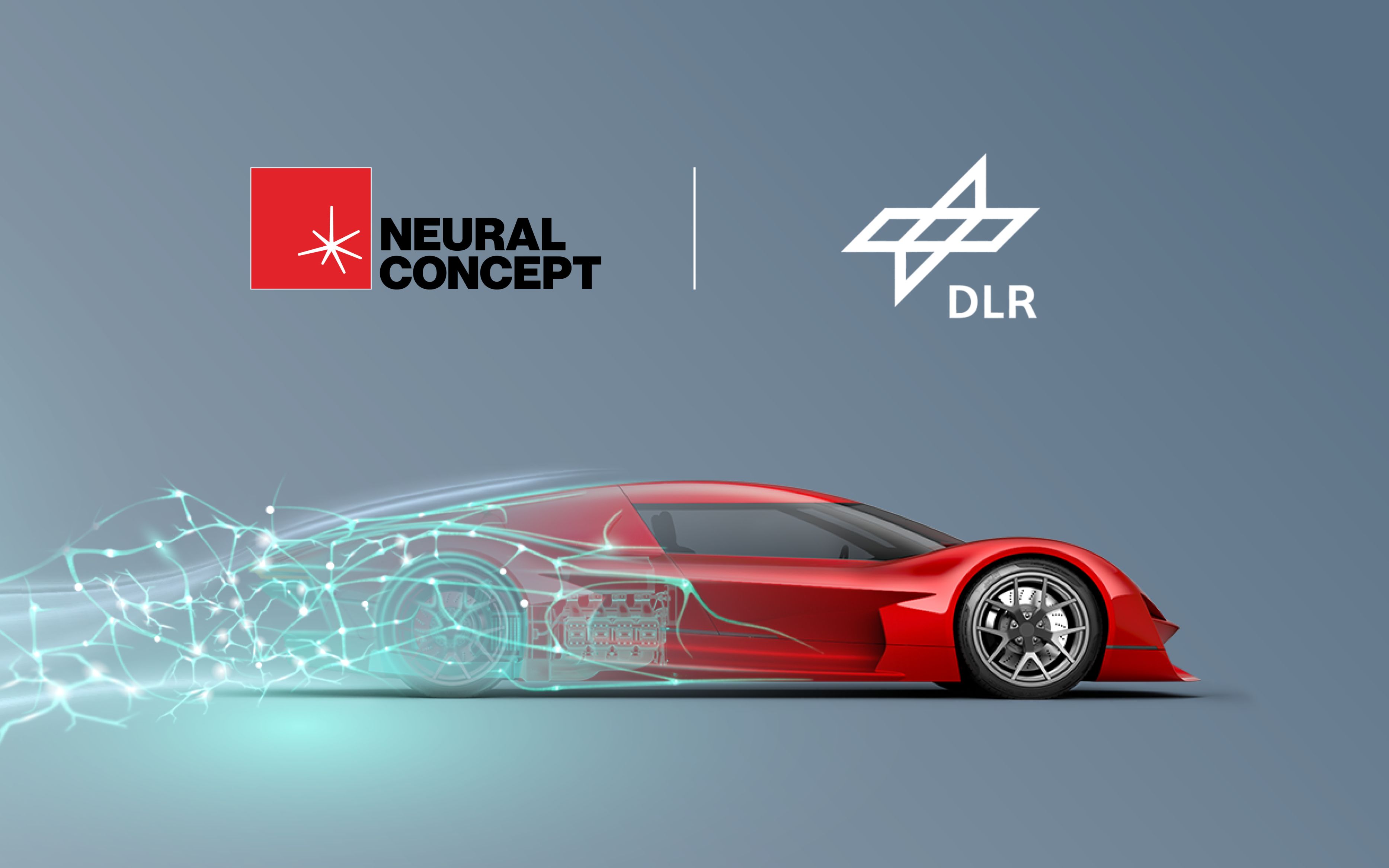

DLR, the German Aerospace Center, partnered with Neural Concept to optimize the design of crash boxes in the context of novel vehicle development.
The development of next-generation vehicle concepts necessitates innovative approaches to overcome design challenges and accelerate development cycles. In this article, we explore how Neural Concept Shape (NCS) models, based on Geometric Deep Learning, were able to accurately predict the behavior of these components during a crash, a highly non-linear phenomenon. We also showcase how these models can be leveraged to explore a large design space, to converge faster towards innovative design solutions, being here 10% more performant than the more classical designs.
Crash Box Design for Next Generation Car
Transport systems around the world are undergoing significant transformations, necessitating the development of environmentally sustainable and economically feasible solutions for future mobility. The convergence of urbanization, electrification, modularization, and high automation levels demands the systematic development of new vehicle concepts and structures. Among these innovative concepts is the Urban Modular Vehicle (UMV), a part of the Next Generation Car (NGC) family, which focuses on urban mobility, electrification, and the introduction of highly automated systems. Central to the development of the UMV is the need for modular systems that enable the production of various vehicle models while reducing costs. A critical aspect of this modular design is the crash box, which plays a crucial role in ensuring the safety of the vehicle and its occupants during collisions.
Challenges in Crash Box Design
The crash box is an energy-absorbing component typically incorporated into vehicles to protect passengers in the event of a collision. It is primarily responsible for dissipating kinetic energy during an impact, minimizing the transfer of forces to the vehicle's main structure. Developing an optimized crash box design presents several challenges. Firstly, crashworthiness requirements demand a balance between reducing weight and maintaining safety, necessitating innovative solutions. Secondly, the crash behavior is highly nonlinear, making it complex to find an optimized design that maximizes energy absorption while considering manufacturing constraints. As a result, the design process for crash boxes largely relies on engineers' experience and best practices, with limited exploration of the design space.
Deep Learning and Geometric Convolutional Neural Networks
In recent years, deep learning has made significant advancements, opening up new possibilities for solving complex problems. Neural Concept, a pioneering company, has developed unique algorithms based on Geodesic Convolutional Neural Networks (GCNN) for training deep learning models using raw 3D geometrical and simulation data. This revolutionary technology shortcuts traditional simulation chains, providing post-processed results directly from the CAD design.
Improving Crash Box Performance
The objective of this project was to leverage the power of deep learning, specifically GCNN, to enhance the performance of crash boxes and shorten development cycles. By using FEA simulations, a surrogate model based on GCNN can be trained to accurately predict crash box behavior. Unlike traditional methods, this approach does not rely on specific parametric representations of the design space, enabling the reuse of existing simulations and leveraging transfer learning capabilities, as well as allowing greater flexibility in exploring the design space and finding optimal designs Geometric Neural Networks are well-suited for accurately predicting complex local field quantities, such as deformation, temperature, and pressure, making them ideal for crash box optimization.
The Use Case
To illustrate the application of deep learning in crash box design, a frontal head-on crash scenario for the Urban Modular Vehicle is considered. The initial design of the aluminum alloy crash box, along with several design variables, is evaluated.
The goal is to generate an optimal lightweight design within volumetric constraints while maximizing Specific Energy Absorption (SEA).
The surrogate model trained using GCNN will be compared to ground-truth values, demonstrating its accuracy and reliability in predicting crash box performance. Trained from various parametric design of crash boxes, the model is able to predict on unseen, test design, with an high accuracy on both the scalar and field quantities (R2 values > 0.98 and mean displacement error of 2.4 mm).

Then, using Neural Concept's design module, new trigger designs can be created, < 1 sec per created design. Designs are independent from any underlying parameters, so the range of created geometries is much wider than the original design space. Pattern type, location, depth, thickness, width, could then freely vary.
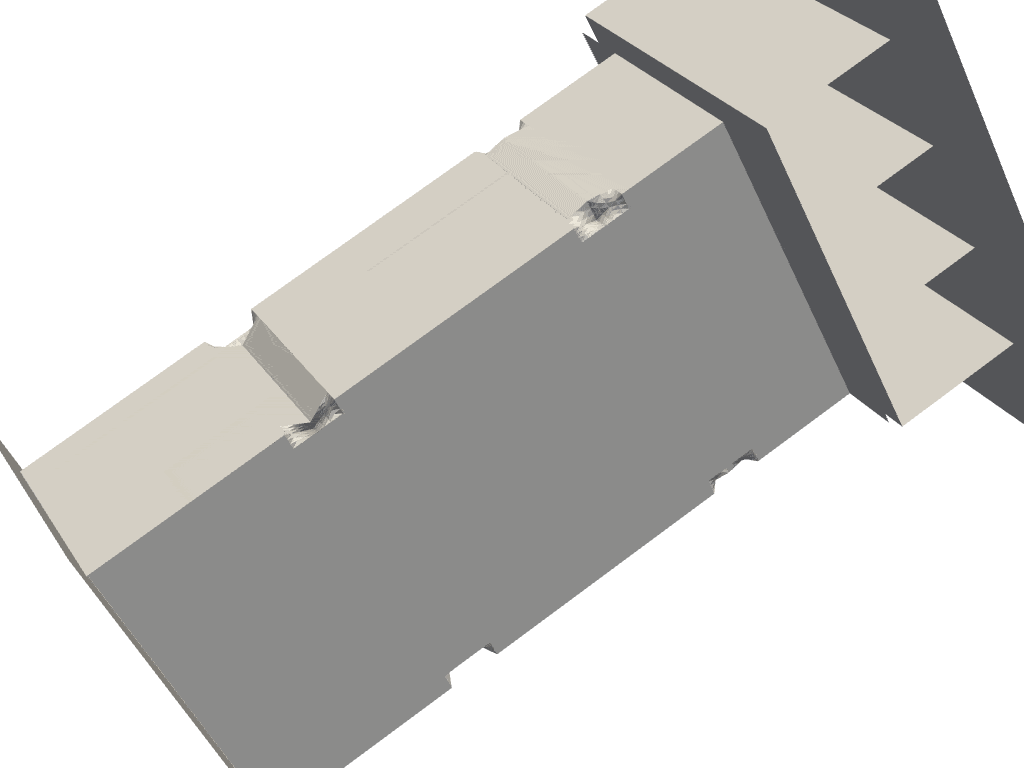
Finally, the design module coupled with the predictive model for optimization, with the goal of :
- Maximizing the absorbed energy
- Minimizing the max intrusion
- Keeping a Crushing Force Efficiency > 0.7

A full optimization loop was run, and, while exploring the resulting Pareto Front, the model was able to converge towards designs showing a 10% improvement in both target quantities, compared to baseline designs. These results were confirmed by the simulations afterwards.
Results & Conclusion
The development of next-generation vehicle concepts necessitates innovative approaches to overcome design challenges and accelerate development cycles. Deep learning techniques, such as Geometric Convolutional Neural Networks, offer a transformative solution for optimizing crash box designs. By leveraging raw 3D geometrical and simulation data, engineers can train surrogate models that accurately predict crash behavior, maximizing safety while reducing weight. This paradigm shift in crash box design represents a significant step towards achieving the mobility of the future, characterized by environmentally sound and economically feasible solutions. As deep learning continues to evolve, it holds the potential to revolutionize not only crash box design but also various aspects of automotive engineering and crashworthiness optimization, making transportation safer and more sustainable.
Reference
Deep-Learning for Enhanced Engineering: Evaluation of crash performance for novel vehicle concepts paper, presented at NAFEMS World Congress, May 2023. P. Lualdi, S. Sokolaki, Dr.-Ing. Ralf Sturm (Institute of Vehicle Concepts (FK), German Aerospace Center (DLR), Germany); A. Pozzetti, T. von Tschammer, K. Kritikos (Neural Concept, Lausanne, Switzerland).