How to feed an AI-powered predictive tool?
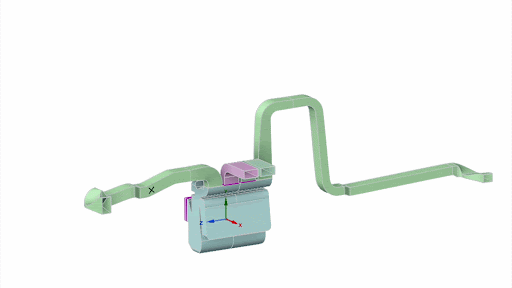
With Engineering Intelligence, designers get access to instant high-fidelity predictive tools. The question is, from where comes the data used to train the tool?
The neural network is fed by both CAD geometries and CAE simulations in order to create predictions for product designers. Since each team provides respectively these raw data without the need to transform them, the tool becomes more than the sum of its parts.
Neural Concept Shape is able to combine any CAD data with any CAE data. CAD designs can be STL/IGES or native formats, while CAE data can come from simulations with arbitrary levels of sophistication; such as multiphase, transient etc. Therefore, the predictive tool introduces realistic CAD/CAE representations which is a step forward from traditional methods. Indeed, reduced-order modelling traditionally requires to parametrize CAD geometries, while CAD-embedded simulations usually requires downgrading in the CAE fidelity and introduces uncertainties.
This Production App exemplifies the powerful convergence from realistic CAD and CAE data. The example captures a workflow for an automotive HVAC’s fluids and thermal performance. In this design scenario, the inputs to the AI-tool provided by product designers are 1) raw CAD geometries and 2) operating parameters (e.g. inlet air mass flow rate and temperature). The expected outputs are air pressure, velocity and temperature, available in 3 seconds after importing a CAD.
The predictive tool is much more than “easy-to-use software for designers”. Its usage is immediate in a design environment, because it encapsulates a workflow commissioned by the final users and tailored to their personalized needs.

































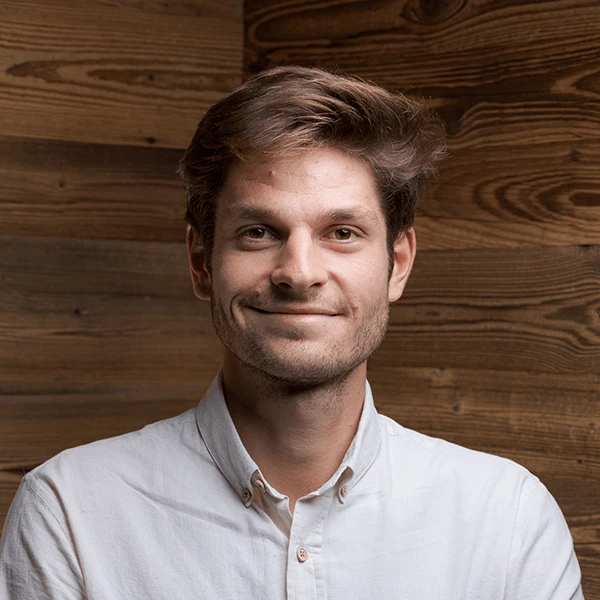

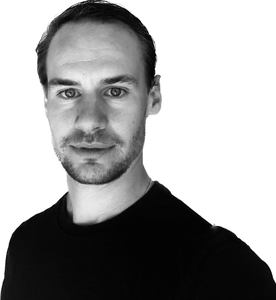
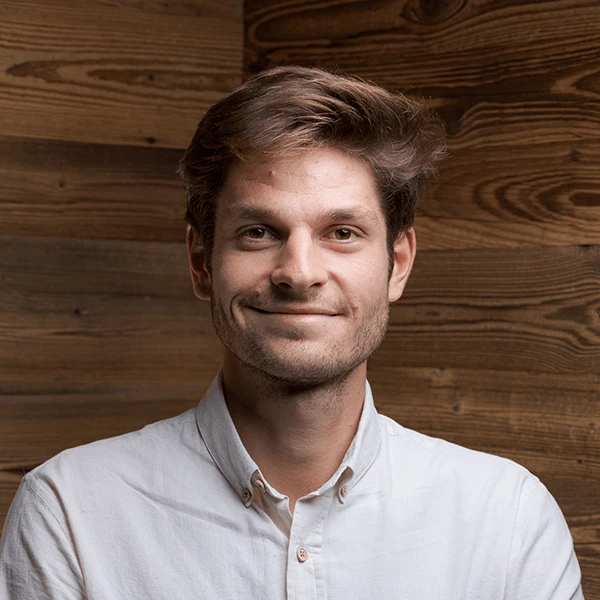










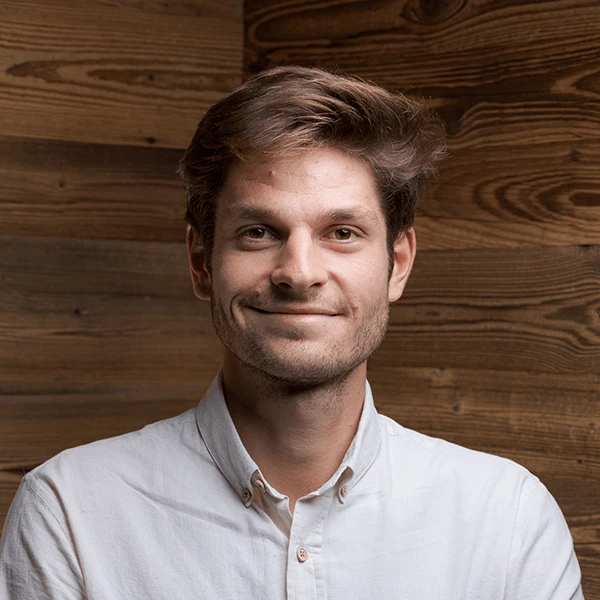














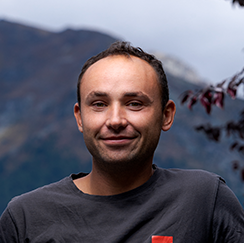
