How AI Is Used in Predictive Maintenance
%20(1).jpg)
Whether dealing with conveyor belts in a manufacturing or mining plant or a leaking pipe in an oil pipeline, things wear down or break and must be fixed or replaced. Unfortunately, failure is often no fatality; there is a lack of proactive identification. This article will focus on the contribution of Artificial Intelligence to proactive or predictive maintenance to reduce the risk of catastrophic or costly problems before they occur.
From the fundamentals of predictive maintenance to recent machine learning algorithms, we will show how artificial intelligence and machine learning could reshape best practices as a powerful tool, giving actionable insights and empowering organizations to "stay ahead of failure." Ideally, we will be equipped to overcome challenges for a more sustainable future with increased productivity and better product quality.
We will see how machine learning in predictive maintenance can offer benefits such as cost savings, increased reliability, extended equipment life, and customer satisfaction.
But what is predictive maintenance?
Predictive maintenance is a proactive approach to predicting and preventing failure.
What is the link between predictive maintenance and the recent disciplines of data analytics and machine learning?
Reactive vs Preventive vs Predictive Approaches
We will initially review three very different approaches (reactive, preventive, and predictive) before moving to the advanced topic of artificial intelligence applications later on.
The reactive or after-the-fact corrective approach is a sort of "unintelligent feedback" that reacts to breakdowns as they occur. We can imagine them suited to low-cost, non-critical assets that don’t pose safety or operational risks in case of failure.

Preventive vs Predictive Maintenance
Preventive and predictive maintenance are proactive strategies to fix things before they break.
Preventive approaches leverage human knowledge and experience to help engineers schedule regular corrective actions to prevent failure.
Predictive strategies use advanced data techniques and online sensors to forecast potential breakdowns.
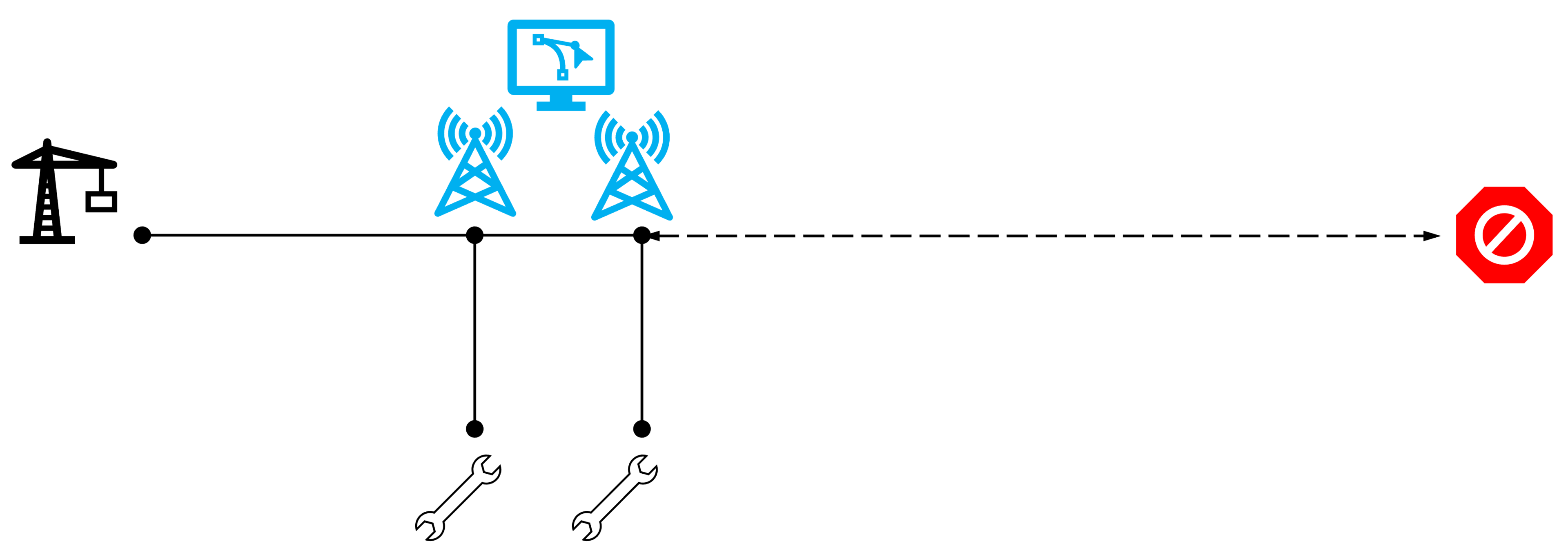
Preventative Maintenance
Prevent(at)ive methods involve regularly scheduled inspections, repairs, and replacements of equipment components to prevent equipment failure. This approach relies on historical data and manufacturer recommendations to determine intervention intervals and tasks. For example, daily preventative maintenance tasks include changing oil filters in vehicles at scheduled mileage or inspecting machinery for signs of wear and tear every month.
Preventative maintenance aims to identify and address issues early, minimizing the risk of unexpected breakdowns and optimizing asset reliability and lifespan. But it may not always be the most efficient approach to preventing machine failure: over-maintenance can lead to unnecessary downtime and expenses, and under-maintenance can result in missed opportunities to prevent failure.
Predictive Maintenance
Predictive maintenance relies on real-time data and advanced analytics to predict when equipment will likely fail. Predictive maintenance algorithms can identify patterns and anomalies indicative of impending failure by continuously monitoring machine performance indicators such as temperature, vibration, and fluid levels.
A technique used in predictive maintenance is condition-based monitoring, where sensors collect data on equipment health and performance. Machine learning analyzes this data to detect early warning signs of potential issues for maintenance teams.
The advantage of predictive maintenance is its ability to maximize productivity: organizations can avoid costly unplanned equipment downtime and reduce unnecessary maintenance activities. Predictive maintenance can extend asset lifespan by preventing premature failures and optimizing maintenance schedules.
Choosing the Right Strategy - Preventive or Predictive?
Preventive and predictive maintenance are two proactive strategies organizations can use to further equipment effectiveness. The choice between preventive and predictive maintenance depends on factors like the asset's criticality and the availability of data and resources. Combining both strategies can allow organizations to leverage the benefits of proactive and predictive maintenance services while minimizing the drawbacks of each approach.
AI in Predictive Maintenance and the Role of Data Scientists
Predictive maintenance requires a predictive model to support it, and machine learning is an AI branch that offers various data analysis approaches to prediction.
But how can machines learn? Let us review some basic concepts.
Supervised learning involves training a model on "labeled" data. Here, the artificial intelligence neural network algorithms learn to map input features to corresponding output labels. This approach is commonly used to predict failure probabilities based on historical data.
An unsupervised machine learning model deals with unlabeled data to identify hidden patterns or clusters within the dataset. Unsupervised learning algorithms can uncover anomalies or detect deviations from normal equipment behavior in predictive maintenance, signaling potential failures.
Reinforcement learning involves training an agent to interact with an environment and learn optimal actions through trial and error. It can be used to optimize maintenance schedules or resource allocation strategies to minimize unplanned downtime and maximize reliability.

The role of data scientists is to strive for accurate predictions based on datasets collected during operations and integrate any new data into a working predictive model rather than working on practical maintenance issues. The first state for data scientists is collecting information.
Maintenance Data Collection and Elaboration
Data collection in predictive maintenance comes from various sources, such as sensors, monitoring systems, and historical records. Records include equipment health metrics, operating conditions, and environmental factors.
Once datasets are collected, they undergo thorough analysis using statistical techniques, machine learning algorithms, and data visualization tools. Analysis helps identify patterns and trends and spot anomalies indicative of potential equipment failures or maintenance needs. Predictive models are delivered as easily deployable tools, giving meaningful insights to anticipate failures in manufacturing operations and other operations, such as logistics, without requiring data science skills.
Thus, maintenance professionals in the manufacturing industry can anticipate and proactively address issues using embedded or canned software solutions through advanced analytics and predictive modeling before they escalate into costly breakdowns. Data elaboration enables continuous machine performance monitoring, facilitating condition-based interventions and optimizing maintenance schedules.
Benefits of AI-Powered Predictive Maintenance
AI-powered predictive maintenance offers numerous advantages that revolutionize practices across industries.
First, it significantly lowers maintenance costs by enabling proactive measures to address potential equipment failures before they occur. By analyzing data from sensors and monitoring systems, AI algorithms can detect early warning signs of malfunctions. This allows timely interventions to prevent equipment failures and reduces the need for costly emergency repairs.
Furthermore, AI-powered predictive maintenance contributes to extending the product's lifecycle. By addressing issues promptly and preventing unnecessary strain on equipment, predictive maintenance reduces the frequency of replacements, machine downtime, and capital expenditures, maximizing organizations' return on investment.
AI-driven predictive maintenance improves operational efficiency by optimizing maintenance schedules and resource allocation. Analyzing real-time data and predictive analytics allows AI algorithms to prioritize tasks and allocate resources more effectively. Maintenance teams achieve more with fewer resources: labor costs are reduced, and service technicians' productivity increases!
Example - Energy Grids and the Role of Predictive Maintenance AI
Energy grids are networks that transmit electricity from energy plants to consumers. They comprise interconnected components, including generators, transformers, and transmission lines. AI-based optimization algorithms can analyze data from smart meters, weather forecasts, and energy consumption patterns to accurately predict electricity demand and supply fluctuations.
Preventing Unplanned Outages
AI-based risk assessment tools can analyze historical data to identify high-risk areas susceptible to failures. By prioritizing maintenance and upgrades for critical assets, energy companies can reduce the likelihood of unplanned outages. AI-based prediction systems analyze data collected from sensors to detect early warning signs of potential failures, such as equipment overheating or voltage fluctuations, and alert maintenance teams.

Example of AI in Predictive Maintenance - Logistics and Fleet Management
Overseeing and coordinating a fleet of vehicles, such as trucks or ships, ensures efficient operations and maximizes productivity. AI technologies provide real-time insights into vehicle performance, driver behavior, and operational process logistics.
AI uses onboard sensors, GPS devices, and telematics data to monitor vehicle health and location and analyze driver behavior. Machine learning algorithms identify patterns and trends from data, enabling fleet managers to make data-driven decisions to optimize their operations.
For example, artificial intelligence algorithms can predict when vehicles are due for maintenance based on existing data on mileage, engine diagnostics, and historical records.
By scheduling proactive repairs based on predictive models, maintenance professionals can minimize unplanned downtime, with vehicles in optimal condition and less maintenance costs.
Predicting Component Failures
Predictive maintenance of components is an area where AI is transforming operations. In commercial trucking fleets, AI-powered predictive maintenance systems can analyze the data, for instance, from brake systems, to detect early warning signs of potential failures, such as abnormal temperatures, vibrations, or fluid leaks.
Role of AI in Linear Programming and Logistics
Linear programming is a method for optimizing performance with given constraints to find the optimum resource utilization. Artificial intelligence algorithms can help drivers avoid traffic congestion, road closures, and other delays by optimizing routes in real-time.
As a practical example, logistics companies collect real-time data from traffic cameras, GPS trackers on their delivery vehicles, weather reports, and historical traffic patterns. The AI algorithm processes the collected data to predict traffic issues and calculates the most efficient routes using techniques such as linear programming.
Thus, artificial intelligence ensures that deliveries are made on time and reduces the risk of late deliveries or missed deadlines.
Conclusions - Dark and Bright Sides?
By leveraging sensors and data, AI in predictive maintenance can empower organizations to manage their assets proactively.
The dark side of continuous monitoring is that it can potentially promote a "surveillance regime" extended to objects used by citizens and companies.
The bright side is that the continued evolution and refinement of AI technologies will pave the way for even greater advancements in the future!
Customer satisfaction will ever increase with a better product and service experience, a dramatic reduction in unplanned downtime, and other improvements in our daily lives.