How AI is Transforming Additive Manufacturing
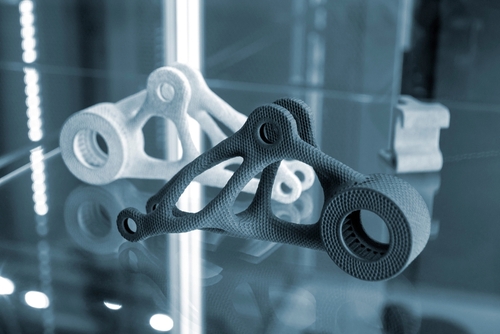
Additive manufacturing (AM), also known as 3D printing, is a manufacturing method that shares similarities with natural additive processes, such as wasps building nests layer by layer.
3D printing is starting to impact various industries, including automotive, aerospace, and biomedical. We will investigate the integration of Artificial Intelligence into the 3D printing process to see how it can further improve these processes.
AI, particularly machine learning, contributes to various stages of the manufacturing process, from design and simulation to real-time control and defect detection. This article examines AI applications in additive manufacturing, focusing on the automotive, aerospace, and biomedical industries. While the potential benefits are substantial, it's important to acknowledge that some may be skeptical about relying entirely on Artificial Intelligence in these critical sectors.
While AI offers powerful capabilities, it is not a magic solution. Successfully applying AI in manufacturing requires a deep understanding of the technology and the manufacturing process it's being applied to.
AI Techniques for Innovation and Quality
One of AI's primary advantages in AM lies in streamlining design and production processes for new innovative products. AI algorithms can optimize part designs specifically for additive manufacturing, reducing complexity and simplifying production. This optimization leads to more efficient material usage, reduced manufacturing costs, and a faster time to market for new products. Deploying the earliest possible AI in the design phase, manufacturers can create parts optimized for performance.
AI can also enhance AM quality assurance. Traditional quality control methods relied on post-production inspection. However, AI enables a more proactive approach. AI-driven quality assurance methods can predict potential issues before production begins, validate designs for 3D printing suitability, and minimize waste and iteration cycles. This proactive strategy significantly reduces trial-and-error processes' time and material costs, leading to more efficient and cost-effective production.
Furthermore, AI supports optimized decision-making throughout the 3D printing workflow. By providing data-driven insights, identifying potential bottlenecks or issues, and suggesting process improvements, AI enables manufacturers to make more informed choices. This enhanced decision-making capability extends from the initial design phase through production planning and execution, contributing to overall operational efficiency.
The integration of AI also leads to accelerated processes across various stages of AM. From design optimization and production planning to post-processing, AI can speed up numerous aspects of the manufacturing process. This acceleration contributes to overall efficiency gains and faster product development cycles, allowing manufacturers to respond more quickly to market demands and stay ahead of the competition.
The culmination of these benefits results in significant improvements to the bottom line. Manufacturers leveraging AI in their AM processes often see measurable cost savings, increased operational efficiency, and enhanced product quality. Manufacturers can create a more robust and efficient manufacturing process by capitalizing on AM's inherent advantages while addressing its challenges through AI.

AI's Role in Additive Manufacturing
AI in 3D printing is changing production by enhancing AM technologies. AI algorithms analyze historical data and real-time information to optimize manufacturing, improving efficiency and quality. This integration allows for ongoing improvement of 3D printing processes, resulting in more reliable and cost-effective production.
One key application of AI in 3D printing is design and topology optimization. AI-driven generative design tools enable the creation of complex geometries that were previously impossible or difficult to achieve with traditional methods. These tools use AI to determine optimal material usage and structural integrity, resulting in lightweight yet strong components.
AI improves design and manufacturing in the automotive sector, producing innovative and efficient components. It supports innovation by expanding design possibilities and ensuring high levels of quality assurance. The biomedical industry benefits from AI through improved manufacturing of customized medical devices and implants.

AI and Machine Learning
Artificial Intelligence has emerged as a game changer in various industries, and in this section, we will explain the fundamentals of AI technology and its potential applications in this field. This chapter aims to provide an overview of AI.
Artificial Intelligence refers to developing software that performs tasks that typically require human intelligence. These previously impossible tasks for a computer include visual perception, speech recognition, decision-making, and language translation. AI encompasses various methodologies and approaches, with machine learning (ML) being one of the most prominent.
Machine Learning, a subset of AI, focuses on creating software that can learn and improve from experience without being explicitly programmed. One key component of ML is the use of neural networks (NNs), which are inspired by the structure and function of the human brain. NNs consist of interconnected nodes or "neurons" that process and transmit information, allowing the system to recognize patterns and make decisions.
Neural networks are a game changer in AI, providing the foundation for many advanced AI technologies such as Machine Learning and 3D Deep Learning. These networks can be trained on large datasets to recognize patterns and make predictions based on input data. In the context of Additive Manufacturing, NNs can be used to analyze complex processes in manufacturing, predict potential issues, and optimize production parameters.
NNs are powerful because they can handle non-linear relationships, and adapt to new data. When more data becomes available, NNs will continue to learn and improve their performance. This continuous learning makes NNs particularly useful in dynamic manufacturing environments where conditions change frequently.
One key application of AI and NNs in manufacturing is the development of predictive models. These models use historical data and current inputs to forecast future outcomes or behaviors. In AM, predictive models can be game changers by anticipating potential issues before they occur, optimizing production schedules, and improving overall efficiency.
Applying AI through predictive models in Machine Learning or Deep Learning can support various aspects of the manufacturing process. For example, AI can predict machine maintenance needs, forecast material requirements, or estimate production times. By leveraging these predictive capabilities, manufacturers can reduce downtime, minimize waste, and improve product quality.

AI Technology in Manufacturing
The range of AI technology applicable to manufacturing is vast and continually expanding. Some key AI technology include:
Machine Learning: As mentioned earlier, Machine Learning forms the basis for many AI applications allowing applications to learn from data and improve their performance over time.
Computer Vision (CV): This AI technology enables machines to interpret and understand visual information from the world. In AM, CV can be used for quality control, detecting defects in printed parts.
Natural Language Processing (NLP): While less directly applicable to AM, NLP technologies can support communication between humans and machines, potentially simplifying the operation of complex manufacturing equipment.
Reinforcement Learning: This technology involves AI agents learning to make decisions by receiving rewards or penalties. It can be particularly useful in optimizing complex manufacturing processes.
Applying AI in additive manufacturing has proven to be an innovation opening up new opportunities for companies and engineers.

While AI technology, particularly NNs and predictive models, have shown great promise in manufacturing, they don't operate in isolation from other software.
Physics-based models, which use fundamental scientific principles to simulate physical processes, are essential many manufacturing applications. Integrating AI with physics-based models by feeding AI with simulation data represents an exciting frontier in manufacturing technology. Manufacturers can create more robust and accurate approaches by combining AI's data-driven approach with the fundamental understanding provided by physics-based models. This hybrid approach can support more reliable predictions, better process optimization, and improved decision-making.
Optimization is a key focus area where AI technology has shown promise for the innovation of products. Optimization algorithms can be applied to various aspects of the manufacturing process, from design to production to supply chain management. NNs and other AI technology can analyze datasets to identify optimal solutions that might not be apparent to human operators. For example, AI can optimize the design of parts for additive manufacturing, considering factors such as properties of materials, printing orientation, and support structures, using surrogates of 3D simulation software. This can produce lighter, stronger, and more efficient parts.
Materials Used in Additive Manufacturing
Additive manufacturing creates complex structures layer by layer, but what are the materials used? We will list the most popular ones.
- Polymers: Thermoplastics like Polylactic Acid, ABS=Acrylonitrile Butadiene Styrene, or nylon are commonly used due to their ease of printing. Photopolymers are used in stereolithography to offer high precision and smooth finishes. An application example is dental applications.
- Metals: materials such as stainless steel, titanium, aluminum, and Inconel are employed in powder form for techniques like Selective Laser Melting (SLM) and Electron Beam Melting (EBM), producing high-strength, heat-resistant parts for aerospace and medical implants.
- Ceramics: Advanced ceramics, including alumina, zirconia, and silicon carbide, are printed using binder jetting or stereolithography - they are renowned for their hardness, thermal stability, and for biocompatibility, making them suitable for applications in biomedical devices and aerospace.
- Composites: Combining polymers with fibers (carbon, glass) or metal powders results in materials with enhanced strength and durability. These composites are used in industries requiring lightweight and robust materials, for instance in the automotive industry and for sports equipment.
- Biomaterials: For bioprinting, hydrogels, and bio-inks composed of living cells and biodegradable polymers are used to create tissue scaffolds and organ models, pushing the boundaries of medical research and regenerative medicine.
Improving Production Efficiency and Quality Assurance
Production efficiency is the key measure for successful additive manufacturing. AI helps reduce production time and material waste. AI can identify potential issues and suggest process adjustments by analyzing data from previous manufacturing runs, ensuring smoother production.
AI excels in quality assurance. Traditional quality control methods can be time-consuming and prone to human error. AI-powered CV can inspect components in real time, identifying defects and deviations from desired specifications accurately to ensure that only high-quality products reach the market. This reduces the need for rework and improves overall productivity.
Industry Applications: Artificial Intelligence in the Automotive Sector
AI in automotive industry is gaining traction because of a long tradition in additive manufacturing coming from the age where it was known under different names.
Since the 1990s, "rapid prototyping" (even deployed at a small case) and the production of complex components have changed automotive design and production. Nowadays, AI-driven generative design allows engineers to create optimized structures that reduce weight without compromising strength. This is particularly important in electric vehicle development, where weight reduction is crucial for improving battery efficiency and range.
AI also improves manufacturing efficiency by identifying potential issues and optimizing production parameters. This reduces production time and lower costs, making additive manufacturing a viable option for automotive mass production.
Several automotive manufacturers have successfully implemented AI in their processes.
BMW has used AI-driven design optimization to produce lightweight metal components, reducing overall vehicle weight. Ford has used AI to improve the quality and precision of 3D-printed parts, ensuring they meet safety standards.
Artificial Intelligence in the Aerospace Industry
The aerospace industry has strict requirements for material performance and structural integrity.
The application of AI in aerospace enables the production of complex components and materials that meet these high standards. Using Artificial Intelligence-driven generative design, aerospace engineers can create lightweight yet strong parts that improve fuel efficiency and reduce emissions.
Artificial Intelligence can also assist in quality assurance and defect detection. Aerospace is an industry where even small flaws can have serious consequences, second probably only to Nuclear Energy!
AI-powered inspection ensures that every component meets the highest quality and reliability standards.
Several aerospace companies have adopted AI in their manufacturing processes. GE Aviation has used AI-driven topology optimization to design and produce complex jet engine components, resulting in weight savings and improved fuel efficiency. Airbus has employed AI to enhance the quality and reliability of 3D-printed parts, ensuring they meet the rigorous standards required for aerospace applications.
AI in the Biomedical Industry
The biomedical industry is experiencing changes with the integration of AI in additive manufacturing. AI-driven design and optimization enable the production of customized medical devices and implants tailored to individual patients. This customization improves the effectiveness of medical treatments and enhances patient outcomes. AI also helps ensure the quality and safety of medical devices. AI-powered inspection detects defects and helps to determine if every device meets regulatory standards. This reduces the risk of product recalls and improves the overall safety of medical devices.
Several biomedical companies have successfully integrated AI into their AM processes. AI-driven generative design is used to create optimized implants that enhance patient recovery. Companies are using AI to develop 3D-printed tissues and organs, advancing regenerative medicine.
How to Optimize Additive Manufacturing Processes
Let's now get more technical on AM. This final chapter explores key aspects of 3D printing optimization, focusing on critical elements from the microscopic melt pool to the overall construction process.
The pool is a small volume of material melted by the energy source (typically a laser or electron beam). The behavior of this pool influences the final product's quality. Efforts to model and control melt pool dynamics have improved material properties and reduced defects in manufactured parts. Researchers have developed sophisticated frameworks for real-time monitoring and control of pool characteristics. These systems can adjust process parameters on the fly, ensuring consistent quality along each printed line and throughout the entire build.
Build and Post-Processing Operations
Beyond the pool, optimizing the overall build process is crucial for efficient manufacturing. This involves careful planning of build orientation, support structures, and layer thickness. Optimizing the scanning strategy - the path the energy source follows to melt the material - can impact build time and part quality.
Advanced software tools now offer comprehensive frameworks for process planning, allowing operators to effectively balance factors such as build time, material usage, and part quality. These tools often incorporate machine learning algorithms to refine and continually optimize build parameters based on historical data. While much focus is placed on printing, post-processing operations are equally critical in creating the AM workflow. These may include support removal, heat treatment, surface finishing, and quality inspection. Efforts to streamline these operations have led to more efficient production lines and reduced manufacturing time. For instance, robotic systems can now handle many post-processing tasks, from support removal to surface polishing, with minimal human intervention. This process not only increases efficiency but also ensures consistency across parts. This shows the key role of automation in optimizing post-processing.
Meeting Increasing Demand Through Process Integration
As the demand for 3D printing parts grows, particularly in industries like aerospace and medical devices, there's an increasing need to integrate AM processes into broader manufacturing workflows. This integration allows for more efficient production planning and resource allocation.
Manufacturing Execution Systems (MES) tailored for 3D printing are being developed to manage and optimize 3D printing operations within larger production environments. These systems can effectively coordinate multiple 3D printing machines, manage material inventory, and schedule post-processing operations, ensuring smooth production flow. Optimizing AM processes is an ongoing effort ranging from the microscopic scale of pool dynamics to the macroscopic level of production line integration. Thus, manufacturers can more effectively meet the growing demand for high-strength, complex, customized parts.
How to Ensure Structural Integrity of AM Parts through Simulation
The convergence of Computer-Aided Design (CAD), 3D Simulation, and Additive Manufacturing creates a digital thread that connects design, simulation, and manufacturing. In this section, we'll explore how these technologies work together to ensure the structural integrity of AM-produced parts, with a particular focus on delicate lattice structures.
CAD: The Foundation of Digital Product Development
Computer-aided design (CAD) is the cornerstone of modern product development. It provides a digital representation of a product, capturing its geometry, dimensions, and other critical attributes. In the context of additive manufacturing, CAD is used not just to define the final product but also the manufacturing process itself.
Advanced CAD systems now incorporate AM-specific features, such as:
1. Support structure generation
2. Lattice structure design
3. Topology optimization
4. Build orientation optimization
These features enable designers to create parts optimized for 3D printing processes, taking full advantage of AM's design freedom. For delicate lattice structures, CAD tools provide the means to design complex geometries that would be impossible to produce using traditional manufacturing methods.
Product Lifecycle Management (PLM) systems act as the central nervous system of the digital product development process. They manage and integrate data from various product lifecycle stages, including design (CAD), engineering analysis, manufacturing planning, and in-service performance.
PLM systems are deployed in:
1. Managing complex specific data specific to 3D printing, such as material properties and process parameters
2. Tracking design iterations and version control
3. Facilitating collaboration between design, engineering, and manufacturing teams
4. Ensuring regulatory compliance and traceability
For delicate lattice structures, PLM systems can help manage the complex data associated with these designs, including detailed geometric information and simulation results.
Digital Twins: Bridging the Physical and the Digital
Digital twins represent a notable advancement in integrating digital and physical systems. A digital twin is a virtual representation of a physical product or process that can be used for various purposes throughout the product lifecycle.
Digital twins can be particularly powerful within these contexts:
Design Optimization
Digital twins can simulate and optimize part designs for AM, considering factors such as material properties, build orientation, and support structures. For lattice structures, this can involve optimizing the lattice design for specific loading conditions while maintaining manufacturability.
Process Simulation
With the digital twin of the AM process, manufacturers can simulate and optimize the printing process before any physical production occurs. This is critical for delicate lattice structures since it can help predict and mitigate issues like warping or incomplete fusion of the lattice elements.
In-Service Performance Prediction
Digital twins can continue to provide value after production, simulating the part's behavior under various operating conditions. This can involve predicting deformation and failure under complex loading scenarios for lattice structures.
Feedback Loop
Data from physical parts can be fed into the digital twin, continuously improving its accuracy and predictive capabilities. This is particularly valuable for lattice structures, where real-world performance data can be used to refine design and simulation models.

Structural simulation, often performed using Finite Element Analysis (FEA), is a critical tool in predicting the performance of parts under various loading conditions. For AM-produced parts, especially those with delicate lattice structures, structural simulation takes on added importance due to the unique characteristics of AM processes:
Anisotropic Material Properties: Many 3D printing processes produce parts with direction-dependent properties. For lattice structures, this means that the orientation of individual lattice elements can seriously affect their strength and stiffness. Advanced simulation tools can account for these anisotropic properties, ensuring accurate part performance prediction.
Residual Stresses: The layer-by-layer building process in 3D printing can introduce residual stresses, which can affect part performance and must be accounted for in simulations. For delicate lattice structures, residual stresses can lead to warping or even failure of individual lattice elements. Simulation can help predict and mitigate these effects.
Topology Optimization: Structural simulation can be coupled with topology optimization algorithms to create optimized parts for performance and manufacturability. Optimizing the lattice density and orientation for lattice structures helps to meet specific strength and weight requirements.
Multi-scale Simulation: Lattice structures often require multi-scale simulation approaches, where the behavior of individual lattice elements is modeled at a fine scale, and this information is then used to predict the overall behavior of the part at a larger scale.
Fatigue Analysis: Fatigue analysis is vital for parts undergoing cyclic loading. Due to stress concentrations at lattice junctions, lattice structures can be susceptible to fatigue failure. Advanced simulation tools can predict fatigue life and identify potential failure points.
- Buckling Analysis: Delicate lattice structures may be prone to buckling under compressive loads. Simulation can help predict buckling behavior and optimize the lattice design to prevent premature failure.
- Thermal-Structural Coupling: The 3D printing process involves rapid heating and cooling cycles, which can induce thermal stresses. Coupled thermal-structural simulations can predict these effects and their impact on the final part geometry and performance.
Additionally, ensuring the structural integrity of delicate lattice structures requires advanced simulation techniques:
Homogenization Methods allow the complex geometry of lattice structures to be represented by equivalent continuum properties, reducing computational complexity while maintaining accuracy.
Many lattice structures exhibit non-linear behavior under large deformations, and non-linear FEA techniques are crucial for accurately predicting this behavior.
Given the variability inherent in 3D printing processes, probabilistic simulation techniques can help quantify the reliability of lattice structures and identify the most critical parameters affecting their performance.
The Future of AI in Additive Manufacturing
The future of AI in additive manufacturing holds promising possibilities. Advances in AI algorithms and the development of new materials and technologies will additionally enhance additive manufacturing capabilities. This will create new opportunities for innovation and enable the production and manufacture of even more complex and customized components.
Improvement and collaboration between industry stakeholders, researchers, and policymakers are essential for realizing AI's full potential in additive manufacturing. By working together, we can address challenges and unlock the opportunities presented by AI technologies.
Conclusion - 3D Printing and AI
AI in additive manufacturing significantly affects the automotive, aerospace, and biomedical industries. AI enables new levels of innovation and productivity by improving production line efficiency, optimizing design, and ensuring quality assurance. While some skepticism remains, addressing concerns related to reliability, ethics, and regulation will be crucial for gaining trust and realizing the full potential of AI in 3D printing. As we continue to explore and develop these technologies, the future of manufacturing looks promising.