Challenges in Generative Design for Automotive (EV) Industry

New product design and manufacturing approaches combine algorithms and computational design into new possibilities for the future of automotive industry. With Generative Design for car technology, designers can experiment and find new with the help of algorithms.
The core concept of (GD) is computational creativity. GD leverages computational processes to generate and evaluate design options guided by set objectives and engineering constraints. What does it mean that the technology is computational and iterative?
- GD relies on computational processes to generate and assess potential solutions. Algorithms, in combination with objectives and engineering constraints, explore the design space.
- GD is an iterative process. The output from each iteration informs the next, ensuring that the final design meets the optimal specifications.
The advancement in machine learning and artificial intelligence has impacted the field of generative design. With smarter algorithms and AI assistance, the GD process has become more capable of handling complex design problems. These improvements have also led to increased automation, allowing designers to spend more time on high-level tasks, such as evaluating and refining design options. Moreover, deep neural networks and other machine learning algorithms have opened up new avenues for GD to generate designs beyond human intuition.

Generative Design Challenges for Engineers — EV Example
Generative design is a powerful tool. However, several challenges must be considered when using this technology. Let's review them in the context of electric vehicle (EV) performance and production.
Predicting Electric Vehicle Efficiency
One of the main challenges in using generative design software for EV efficiency is predicting performance targets such as aerodynamics, weight, and powertrain efficiency. Even if generative design software can generate and evaluate multiple design options, it is up to the designers to interpret the results and decide which design is the most efficient. Engineering simulation comes to help here (more on this later).
Electric Vehicle Production
Another challenge is to ensure the design will be manufacturable. Design criteria and manufacturing methods may not always align, but designers can achieve both with the right approach. A paper by EPFL and Neural Concept shows how generative design software can generate realistic designs. This introduces the subject of AI (figure below).
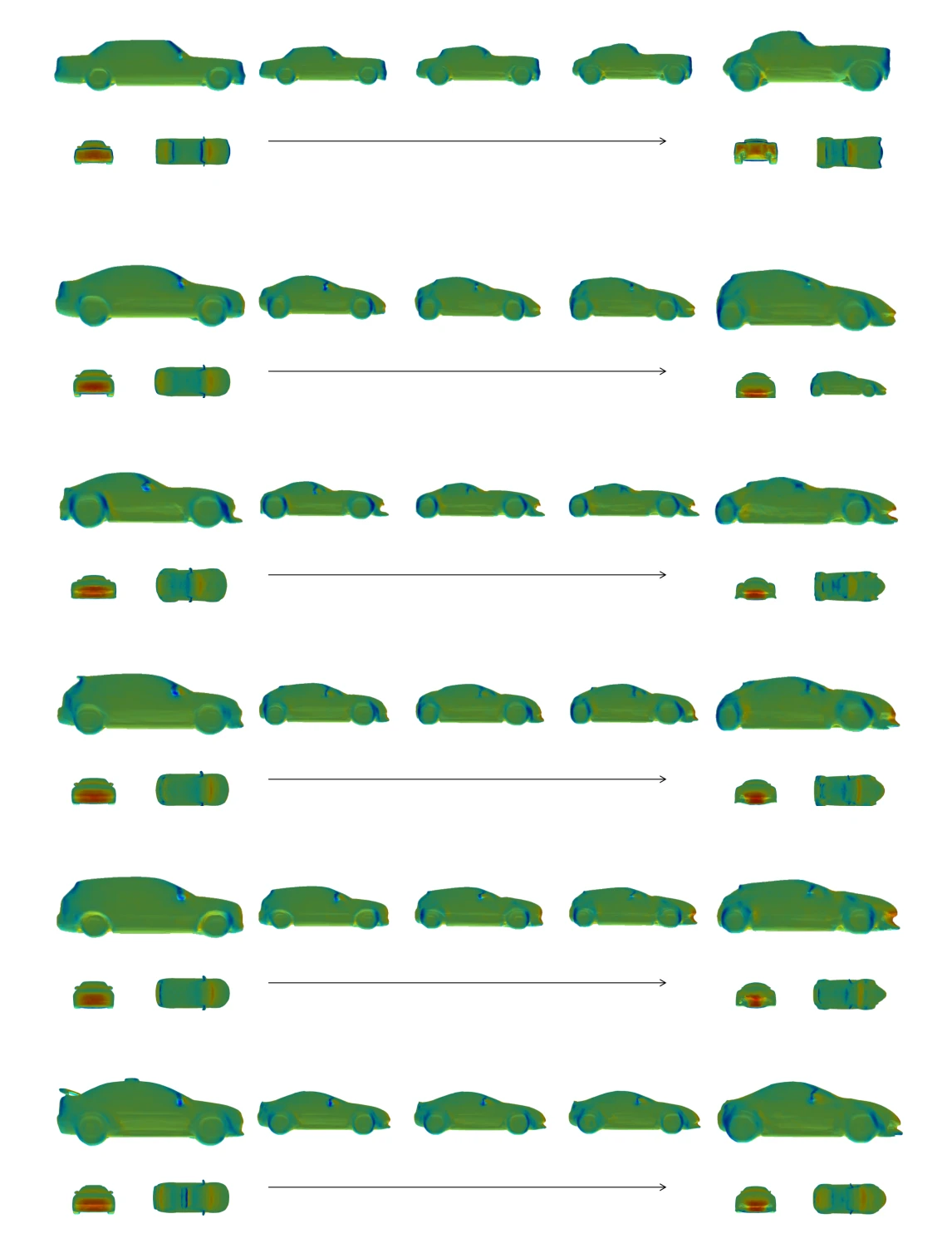
Maintaining High-Performance Standards with AI
In automotive design, designers need to balance the trade-offs between performance, cost, and environmental impact when designing a vehicle; not to mention safety and durability. Finally, we need to consider the design in the real world rather than in ideal conditions. An example is a lightweight seat bracket prototype, where designers optimized the design of the bracket itself. However, they also need to consider safety and durability factors by testing the prototype in real-world conditions with different driving scenarios, such as rough roads, sharp turns, and sudden braking. Data are collected during laboratory or track testing; when enough data is available, AI with Deep Learning can help data-driven decisions with predictive models.
AI is often first deployed in a proof of concept project where the AI partner closely associates with the company experts and implements the design goals expressed by the production engineers. This ultimately produces a predictive tool for designers easily incorporable into engineers' daily activities.
Benefits of Generative Design for Car Manufacturing
In auto manufacturing, GD can achieve several benefits: mass reduction, functional optimization of components and reduction of carbon emissions. Let us examine this in more detail.
Example: Mass Reduction
Definition: efficient energy consumption equates to a greater range per charge. This highlights the importance of optimizing energy consumption for EVs. The less energy is consumed, the greater the range of travel per charge. This is particularly important for EVs, where the range per charge is one of the most significant factors for consumers.
When evaluating electric vehicles for more efficient energy consumption, automotive manufacturers use a range of design and engineering strategies; such as lightweight materials, aerodynamic design, and energy-efficient powertrains- thus potentially leading to the ultimate mobility.
Each kilogram saved results in a decrease in energy consumption or longer autonomy. As a quantifiable example, a 10% reduction in weight can result in a 5% improvement in range.
Use Case - Mubea: Lightweighting Parts while Ensuring Crashworthiness
Generative design capabilities include optimising the structural design of an electric vehicle. This can reduce the overall weight of the vehicle and reduce the materials used, ultimately reducing costs while also making the vehicle more fuel-efficient and environmentally friendly. Use cases can demonstrate how a combination of CAD and AI's predictive capabilities can help design lighter and crashworthy electric vehicles thanks to better components.

Since a key component in hybrid and electric cars is the battery, optimizing the battery encasing is a key challenge to ensure that the product respects crashworthiness' limits while not compromising the target of overall weight reduction.

Automotive Component Optimization
Generative design optimizes components in vehicles, such as suspension systems, engine parts, and HVAC systems; leading to improved performance, durability, and reduced maintenance costs. Heat exchangers, essential for HVAC regulation in cars, must be optimized for efficiency, especially in EVs where the range is limited by energy consumption. After introducing Deep Learning, we will show a use case on a generatively designed heat exchanger.
Generative Design and E-Mobility
Generative Design (GD) can significantly impact EV manufacturing by improving efficiency, reducing waste, and streamlining production processes. Here are some examples:
- Streamlined production process: generative design can optimize the manufacturing process by identifying and eliminating unnecessary steps in the production line. Traditional methods to assemble the battery packs for EVs involve manual time-consuming, error-prone steps such as placing individual battery cells into a pack, followed by manual welding. Using generative design, you could reduce the number of components and streamline the manual assembly. The optimized design could also eliminate manual welding, significantly saving time and cost and reducing risk.
- Reduced material waste: generative design can help reduce material waste during production. An example would be a manufacturer of EV components that uses large sheets of material that need to be cut to size, with the excess being discarded. With generative design, the manufacturer would optimise the component's design, reducing the amount of material required and reducing waste during production.
- Improved quality control: generative design can also improve quality control by using algorithms and simulations to detect potential manufacturing problems before they occur, leading to a higher-quality end product.
- Optimized tooling and fixtures: generative design can be used to optimize the design of tooling and fixtures used in the manufacturing process.
- Better supply chain management: generative design can optimize the supply chain by identifying potential bottlenecks and improving inventory management, resulting in a more efficient and cost-effective production process.
Enhance the Automotive Design Process for EVs
Generative design streamlines the design process for electric vehicles. By allowing designers to generate and evaluate options and create prototypes rapidly, it can speed up the design and develop faster to bring EVs to market faster.
For example, a team of designers working on an EV can use GD to explore several options in the design space with CAD, CAE and AI data-driven simulation and select the most efficient one. The generative design tool can also help them identify potential issues and make changes quickly, leading to a faster design process and shorter time-to-market.
Combining Generative Design Technology & Additive Manufacturing
Combining generative design technology with additive manufacturing/3D printing can lead to many benefits, from improved performance and innovation to faster prototyping and reduced waste.
3D printing supports complex geometries and internal structures that would be difficult or impossible to manufacture with traditional approach. GD can be used to generate and evaluate these complex designs, leading to new possibilities for innovation and improved performance.
Furthermore, additive manufacturing makes creating customized parts on demand possible, rather than keeping a stock of pre-made parts. GD can generate and evaluate designs for customized parts. This leads to improved personalization and a better fit for individual customers' needs; i.e. mass customization, one of the new paradigms introduced in Industry 4.0.
GD can generate and evaluate multiple design options quickly, and additive manufacturing can produce physical prototypes for testing. This can lead to a faster design and development process, helping to get products to market more quickly.
Generative Design Examples: Czinger 21C and 3D Printing
Czinger Cars, a high-performance hybrid vehicle company, demonstrates the potential of combining generative design and additive manufacturing in the auto industry. GD technology enables efficient design optimization and internal structures that wouldn't be possible through traditional methods. Additive manufacturing allows a highly customized and sustainable design, with the ability to produce only the needed parts on demand.
This unique additive manufacturing approach distinguishes Czinger from Tesla's conventional designs and traditional production methods. The combination of GD and 3D printing/additive has led to a highly efficient and unique car that breaks the boundaries of traditional automotive production. The auto industry will likely see more companies adopt this approach for performance, efficiency, and sustainability.

Software for Generative Vehicle Design
Generative design softwares typically have design space exploration and optimization modules. The space exploration module generates many design options, while the optimization module evaluates them based on the specified limits and objectives. Product designers typically use these software packages in the auto industry to optimize the design and performance of their products.
Generative Design Software and AI
Generative design softwares use various optimization and design exploration techniques, some of which are based on artificial intelligence (AI) algorithms. AI helps to generate and optimize the design based on weight, strength, cost, and efficiency.
Some software uses evolutionary algorithms, a subset of AI, to generate and evaluate design options. Others use topology optimization, a mathematical optimization technique loosely considered a form of AI.

Generative Design Software and Deep Learning
Generative design softwares have been widely used in the automotive industry to optimize vehicles' design based on certain constraints and objectives. Deep learning is a new development offering even more benefits for designers.
Deep learning is a subset of machine learning that uses neural networks, an algorithm inspired by the human brain, to perform data-driven prediction tasks such as image recognition. When applied to GD, deep learning can optimize vehicles' design in ways traditional optimization methods cannot.
What is needed for deep learning to become an active part of your design process? Three things: data, data and again, data!
We will see an example of the data-driven approach in the following.
Generative Design Software and Deep Learning: Heat Exchanger Use Case
The design of heat exchangers is a complex task, and there is a wide range of acceptable solutions depending on the application's specific requirements. The number of parameters that describe the geometry of a heat exchanger can quickly become large, particularly as the design becomes more intricate. Numerical simulation of heat exchanger performance is computationally intensive. As a result, designers are often limited in the number of parameters they can iterate on to improve system performance.

To mitigate this challenge, various optimization techniques have been developed to reduce the number of design iterations and minimize the computational cost of the simulation. For example, multi-objective optimization algorithms allow for the simultaneous optimization of multiple performance objectives, such as thermal efficiency, pressure drop, and cost. Additionally, surrogate models based on data-driven predictive analytics can be used to approximate the performance of the heat exchanger system, reducing the need for expensive numerical simulations.
The data-driven approach was based on extracting CAD and simulation (CAE) data from the company PLM (storing CAD and CAE data) to develop a surrogate of CAE predictions in seconds instead of hours. This approach could become a standard and save thousands of man-hours per month in an automotive design department.

Generative Design and Weight Reduction
One of the major areas of focus in any industry is weight reduction. As we become more conscious of the impact of transportation on the environment, the need for lighter, more energy-efficient vehicles with lighter-weight parts becomes increasingly important. Many automotive manufacturers are exploring new technologies to reduce the total weight of their vehicles.
Organic shapes and parts consolidation are design capabilities that reduce the vehicle's mass. Those shapes can be used to create lightweight parts that are aerodynamic and reduce drag, for example. On the other hand, part consolidation is a technique that involves combining multiple smaller parts into one larger part, which can result in a reduction in weight without sacrificing structural integrity.
Using lightweight parts and reducing mass through solutions and technologies is critical to achieving the design goals. With generative design, designers can explore multiple design options and evaluate the potential impact of different design choices on the vehicle's total weight. This allows the design team to create a lightweight vehicle that meets high-performance standards. By reducing the vehicle's overall weight, automotive designers can create a more energy-efficient that is better for the environment and more fuel-efficient for the consumer.
Conclusion and Ways Forward
We have seen how new generative design technologies will greatly impact future product design. The keywords are explore and optimize: by utilizing iterative algorithmic processes, designers can explore many design possibilities and optimize their designs based on engineering constraints and objectives with unprecedented speed and efficiency.
This has led to the creation of generatively designed components and entire generatively designed vehicles, such as a supercar.
There is still some resistance to the generative design approach, even if it offers numerous advantages, because of concerns about manufacturability, safety, and durability and the need to evaluate the in real-world conditions. However, this is no limitation for AI!
The beauty of the AI approach lies in the following:
- Engineering experiences (from the laboratory, on-track testing, CAE simulation, CAD) are stored as data.
- Including the above real-world conditions, data can be considered the building blocks of data-driven predictive analytics, providing valuable insights that can be utilized further to enhance the human experience in design and manufacturing.
- With the aid of predictive AI tools, designers in the future will focus on making informed decisions rather than being burdened with raw, time-consuming computational tasks.