AI in Aerospace Industry: Redefining Intelligent Engineering
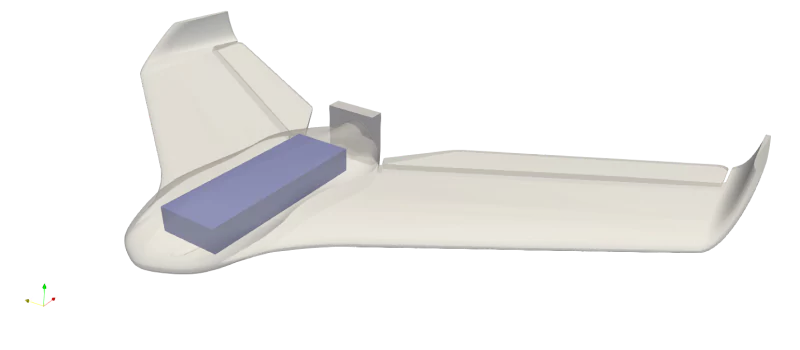
This article, dedicated to AI in aerospace, will deep-dive into technology that helps companies make better decisions, with a special focus on the implications for the product development phase.
Artificial Intelligence (AI) and AI systems have become ubiquitous buzzwords. But it's more than a fashion. Aerospace companies are subject to practical and urgent concerns, ranging from safety to fuel consumption optimization and the safe design of unmanned systems to deliver payloads or passengers.
And headaches are not over. Ever-more-stringent normative trends, such as emissions reduction, are forcing the aerospace industry to think beyond fuel efficiency and embrace a full green transition. This urges the search for a drastic increase in the speed and efficiency of the product development cycle.
When the need for speed is unavoidable and large amounts of data are available, AI and Machine Learning (ML) kick in and are matchless in providing smart solutions that help engineers focus on more intellectual tasks. This is Intelligent Aerospace!
Will AI systems enable design engineers to be more effective? Maybe 10,000 times more effective? As we will see, AI technology helps engineers embrace such "crazy" challenges and practical use cases are already proving it is working.
In the article, we will also address a few concerns by the large public and specialists, such as the availability of data for AI and safety concerns of AI implementation.

What Is Intelligent Aerospace?
An example of the "Intelligent Aerospace" concept is represented by aerospace and defense companies adopting AI systems to speed up their design cycles. The design phase is an upfront stage including concept and detailed design, well before the later stages of manufacturing and final deployment with air traffic control.
What is the purpose of AI technology, and what are the solutions? Why should business leaders in aerospace industries be involved?
Applications of AI in the Aerospace Industry
How is AI reshaping aerospace and defense companies?
AI can help accelerate speed in the concept and detailed design phases. Even if some aerospace programs have long time scales, accelerated speed can help better explore solutions and find new ideas within an allotted timeframe.
We will also show how AI can be implemented in manufacturing and maintenance. AI can help with factory automation, supply chain streamlining, and processing large data sets to help maintenance engineers.
Design Speed
Thanks to optimized designs, AI can help address technical issues such as optimized fuel consumption.
Predictive analytics is a powerful technique for simulating events before they occur, checking the existing situation, and proposing optimized designs (iterative design approach). What has hindered the application of predictive analytics at all levels of product design up to now was its lengthy response time and the costs associated with extensive computational resources, up to thousands of CPU cores.
As we will detail below, the collaboration between Neural Concepts and Airbus with ML has dramatically reduced engineering prediction time from one hour with traditional approaches to 30 ms with machine learning.
There are more use cases under the corresponding section further down, covering various engineering applications ranging from thermal management of onboard electronics to computations of an aircraft flight envelope.
Factory Automation in Aerospace Companies
Aerospace manufacturers are integrating AI into their production processes for commercial aircraft and other applications to optimize supply chains with factory automation solutions or help manage maintenance.
Quality control in production lines. Industries are implementing automation in quality control via AI algorithms that analyze images of aircraft components during manufacturing and can detect defects, cracks, or irregularities. AI ensures that each part meets quality standards. This automated inspection process significantly reduces human error and enhances product quality.
Supply Chain Optimization. AI can optimize supply chain logistics by predicting demand, managing inventory, and identifying cost-effective suppliers. This streamlines production processes and reduces lead times.
Maintenance. As another example, Large Language Models (LLMs) like ChatGPT can assist service engineers. LLMs can process technical manuals and maintenance guides; when engineers encounter an issue, they can query the LLM for troubleshooting steps or repair instructions.
Air Traffic Management
Artificial intelligence algorithms can help air traffic controllers analyze real-time weather patterns, aircraft performance, and other factors. Controllers receive insights to make informed decisions on routing and scheduling.
Autonomous Flights
The IAS (Intelligent Autopilot System) is an autonomous autopilot system that learns from experienced human pilots using Artificial Neural Networks (ANNs). It can pilot large jets, including airliners, by autonomously executing takeoff, climb, cruise, navigation, descent, approach, and landing tasks. The IAS handles extreme weather conditions during landing, including crosswinds, gusts, wind shear, and turbulence.
Main Issues and Concerns for AI in the Aerospace Field
Let us explore a few potential concerns in the application of AI and ML to the aviation industry.
The first topic is managing data; rather than data poorness, the aerospace industry could suffer from fragmentation into departments that do not communicate.
Another more general topic is making decisions related to safety by relying on AI inputs. Here, humans have an important role before thinking of fully autonomous flight implemented in commercial aviation following its initial successful deployment in the automotive industry.
Data Management
AI and machine learning models are already helping the industry to facilitate an inefficient supply chain and address safety concerns with predictive tools based on big data, implementing design automation.
Pioneering applications show how neural networks could optimize an unmanned aerial vehicle, thus incrementing the vehicle’s autonomy.

The issues to overcome in data management are twofold:
- data are often highly fragmented or poorly stored;
- the aerospace and defense industries have security and safety concerns related to data propagation in the supply chain or outside.
Data extraction? It's not an issue.
AI’s ability to extract data can be implemented in any data-generating technology. Still, here, we will focus on Computer-Aided Design (CAD), i.e., 3D representations of object shapes, and Computer-Aided Engineering (CAE), i.e., 3D representations of object functionality.
All industries have safety issues, but aerospace and defense are particularly sensitive. One solution is Neural Concept's AI tool, where the CAD and CAE data can entirely reside within the company to ensure the safety of proprietary and sensitive data. This is why Neural Concept was awarded substantial investments for the propagation of AI technology in industry-grade implementations.
Safety and Reliability
Reliability engineering, proactive risk management, and human-centric design are essential for building safe and dependable AI systems in aerospace applications. As these systems play a pivotal role in aviation, such as flight control and navigation, any failure could have catastrophic consequences.
Emerging during WW2 to mitigate human and cognitive errors in fighter jet modules, the domain of Human Reliability now converges with AI. Human factors, ranging from inaccuracies in data labeling to biases embedded in algorithmic design, can precipitate system failures. When humans, influenced by an AI system, arrive at decisions, it becomes imperative to holistically assess the entire system, accounting for human and AI components.
Use Cases for Artificial Intelligence in Aerospace and Defense
We will review a few use cases of artificial intelligence and machine learning technologies for the aerospace and defense industry to increase product performance and for more innovative technology development.

Aircraft Aerodynamics with Neural Concept and Airbus
In 2019, Neural Concept demonstrated an application of artificial intelligence to aircraft aerodynamics with the industry leader Airbus.
By using Neural Concept Shape (NCS) as a surrogate for CAE (Computer-Aided Engineering), Airbus reduced the average time its engineers spent deploying predictive analytics to support design choices.
The companies collaborated to accelerate the engineering process and generate new design solutions across various design problems in fluid dynamics, structural engineering, and electromagnetics.
With NCS, Airbus dramatically reduced the time it takes to predict the pressure field on the external body of airplanes. The improvement was from an initial one-hour time with the traditional CAE approach down to 30 ms with machine learning. Thus, machine learning accelerated computational processes over 10,000 times.
What are the practical implications of a 10,000 X speed? Given an allocated time for design within a project, a product design team was enabled to explore 10,000 more design changes. Consequently, Airbus engineers approved the usage of machine learning in aircraft aerodynamics.
This case shows that even without massive hardware infrastructure investment, “Intelligent Aerospace” can leverage AI to bring design tools to engineers directly on their desk PC or notebook, without the need to invest in hardware compute farms for daily usage heavily.
Flight Envelope with Artificial Intelligence
Neural Concept Shape is the first machine learning system to understand 3D shapes (CAD) and learn how they interact with the laws of physics (CAE).
AI can emulate complex CAE simulators, making predictions in approximately 30 milliseconds versus hours or even days with previous approaches. The approach allows aerospace engineers to extract maximum value from their data to explore designs with enhanced operational efficiency.
The prominent AI features are Deep Learning and feature recognition. Take the example of computing a commercial aircraft's flight envelope. While most engineers analyze only a few operating conditions, Neural Concept makes it possible to span the aircraft’s flight envelope in a few seconds over a range of velocities or angles of attack.
The customer experience with data-driven AI and ML differs from traditional approaches because operations become real-time instead of taking hours or days. Thus, the potential to explore more solutions in the aircraft design space is dramatically increased.
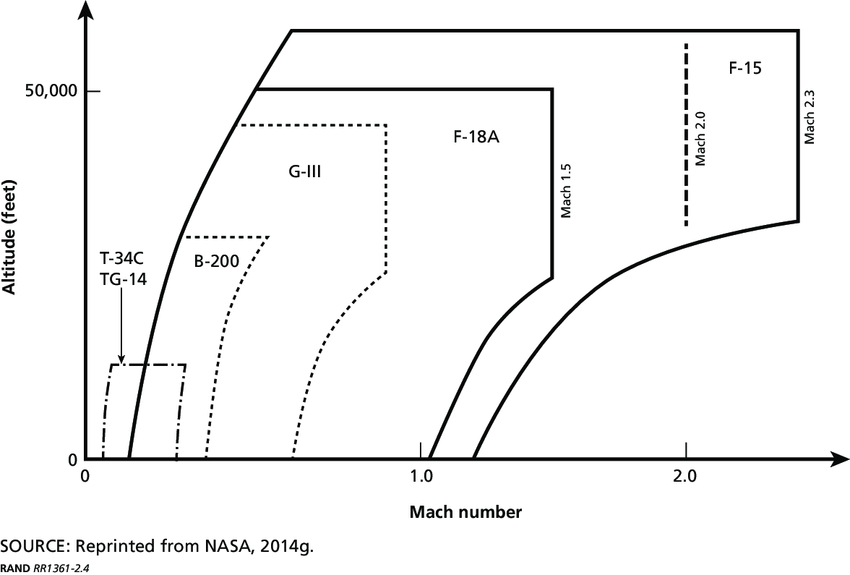
Thermal Effects on Onboard Electronics with Artificial Intelligence
We will summarize how an Artificial Intelligence-based system for thermal transfer simulations of satellite panels helped a space project. The solution can help the satellite industry and any related problems with the thermal management of electronic systems.
A traditional high-fidelity simulation takes about 20 minutes to run, while the successfully met target for AI was to provide a result in tens of milliseconds.
The concept of AI-based real-time simulation can be extended to other engineering aspects affecting satellites and their payloads, such as simulating vibrations and harshness during the launch phase.
Thermal and electronics designers tuned the accuracy and speed of the AI tool in emulating the simulations subject to varying topological changes, such as incrementing the number of chips.
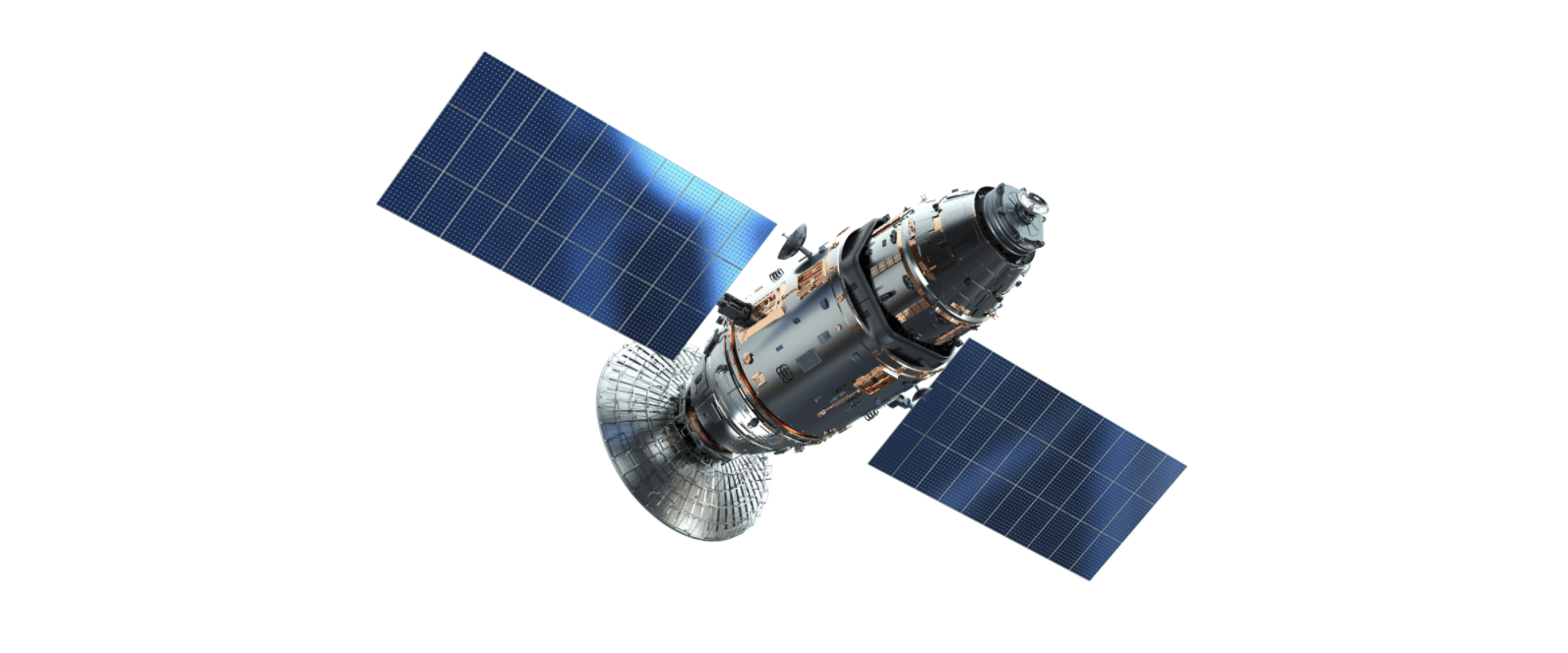
A team of engineers evaluated Neural Concept Shape (NCS) to determine its feasibility as a potential replacement for the simulation workflow. The evaluation was successful.
Additionally, the engineers tested NCS's ability to handle different topologies within a single dataset. They found that NCS successfully assigned the corresponding heat flux to each component and propagated it within the panel using the neural network.

The Technology Behind - Enabling Factors for Engineers
Is AI helping the aerospace and defense industry accelerate R&D cycles, enhance product performances, and solve complex engineering challenges?
NCS is a positive example as a high-end implementation of deep learning. It approach is based on computer vision principles. The NCS algorithms can emulate high-fidelity CAE simulation software, yielding predictions in approximately 30 ms versus minutes to hours (or even days) for CAE, with comparable professional quality.

Professionals in aerospace engineering can deploy NCS on their desks (without the need for compute farms or other expensive infrastructure for daily usage), to manually or automatically explore an unlimited number of designs without calling back the resource- and time-consuming CAE software.
Compared to other artificial intelligence technologies, NCS can be seen as a smart technology that provides faster reproduction (or surrogate) of what R&D simulation experts are doing. This happens thanks to AI-enabled simulators, bringing technology to casual users while reducing human error on the user side. The suite of AI tools can be used both at the aircraft components and full vehicle levels. AI and machine learning algorithms are used without requiring engineers to become data scientists. The key factor is to get hold of historical data from simulation engineers and the associated product design data from the design engineers.
The common objective of aerospace and defense companies and Neural Concept is the deployment of new design methodologies based on ML systems. The outcome is making the ML power accessible into the hands of design engineers for real-time simulation and interactive design optimization. This will help the aerospace industry accelerate and improve the engineering of next-generation aircraft and other AI in aerospace and defense applications.
The Changing Role of Aerospace Engineers
When it comes to integrating AI, aerospace engineers could face challenges but also some benefits. Still, with a clear role organization, this new technology helps human beings deliver solutions faster by leveraging smart technology such as NCS.
In the past, specialist technologies such as "cloud computing" or "gas dynamics simulations" became key factors for design solutions in the aerospace market, helping in topics such as fuel efficiency and predictive maintenance of aircraft systems, even if their exotic names could be unknown to aircraft passengers.
Similarly, AI algorithms are managed by data scientists who analyze data from various sources: recorded data can be originated by experimental testing or 3D simulation (engineering simulation, not to be confused with virtual reality). The aerospace engineer's role is augmented because AI technology can help her/him to focus on high-level topics such as fuel efficiency or predictive maintenance rather than the minutiae of IT implementation and AI algorithms.
Thus, aerospace engineers can take more care of management and commercial aspects like the supply chain and customer satisfaction while using AI tools tuned by specialists for quick daily usage. Rather than being cut, labor costs and job places are diverted to higher-quality intellectual tasks. This is also "intelligent aerospace"!
Conclusion
Whether you need to optimize fuel consumption or ensure the safety of onboard electronics in a satellite, real-time data-driven technology can help the aerospace sector. Training AI to produce actionable tools to support engineers in preventative maintenance and predictive analytics can be daunting. As the use cases have shown, Neural Concept Shape (NCS), starting from computer vision concepts, has been tested by industry leaders such as Airbus as a valuable solution to make once expensive and laborious tools available on any aerospace engineer's desk.
The potential for the application of this smart technology to deliver solutions in engineering prediction is practically limitless, thanks to the wealth of data offered by the aerospace and defense industry!