AI Industrial Design: Applications and Benefits
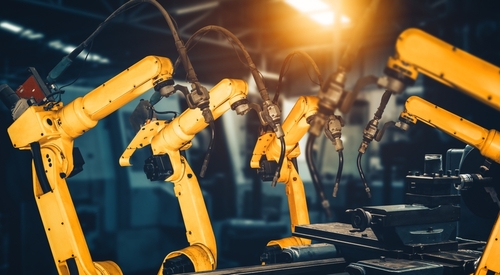
Artificial intelligence and industrial design have crossed roads! This encounter overhauls the way products are conceptualized, developed, and manufactured. AI industrial design is emerging as a real transformation in reshaping both assisting the creative process and surrogating specific technical processes to create new concepts and outstanding products. In this article, we will explore the applications and benefits of Artificial Intelligence in industrial design, examining how it is transforming the industry and opening up new possibilities for innovation, profitability, and sustainability at the same time. We will see how Artificial Intelligence engineering helps design enginers both in design space exploration and optimization.
What is AI in Industrial Design?
AI in industrial design refers to applying artificial intelligence technologies and machine learning algorithms to enhance and optimize the design process. It encompasses many tools and techniques that enable designers to create more efficient, innovative, and user-centric products. AI industrial design leverages the power of data analysis, predictive modeling, and generative algorithms to augment human creativity and expertise.

Importance
Integrating Artificial Intelligence in industrial design is becoming an increasingly essential technology for several reasons. First, it allows industrial designers to tackle complex design challenges more effectively by ingesting datasets and generating innovative solutions. Second, AI tools can significantly reduce the time and effort required in various stages of the design process, from initial concept development to final product refinement. Finally, artificial intelligence capabilities enable designers to create products more responsive to user needs, market trends, and environmental considerations.
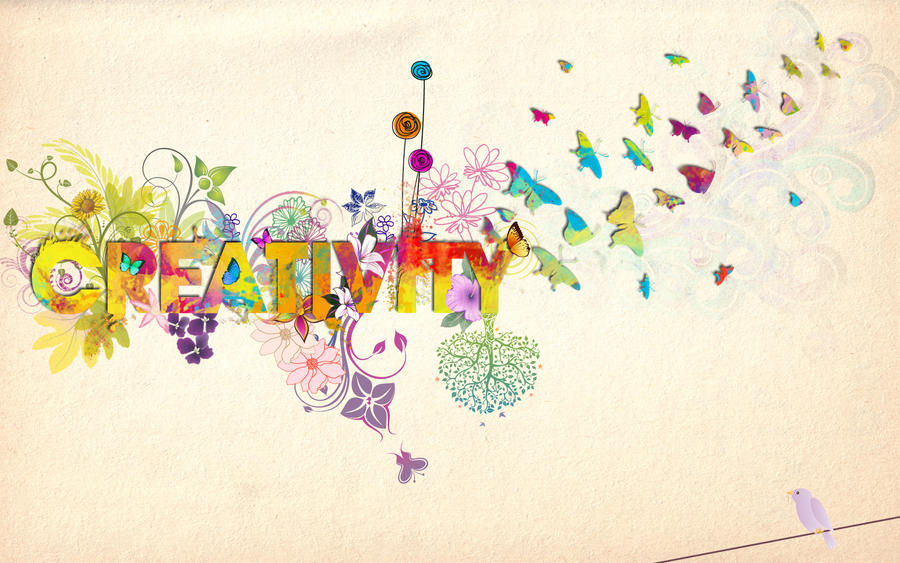
Evolution of Artificial Intelligence in Industrial Design
The use of Artificial Intelligence technology in industrial design has its roots in computer-aided design (CAD) systems, which began to emerge in the 1960s. As computing power increased and algorithms became more sophisticated, the industrial designer started to explore the potential of Artificial Intelligence to assist in various aspects of the design process. Early applications focused primarily on automating repetitive tasks and optimizing existing designs.
Current Trends in Product Design
Artificial intelligence industrial design has evolved into a field encompassing various technologies and approaches.
Current technology trends include:
- Generative design algorithms can create thousands of design options based on specified parameters.
- AI technology that can analyze user behavior and preferences to inform design decisions.
- Machine learning algorithms that can optimize material usage and manufacturing processes.
- Artificial Intelligence image generators that can create realistic product visualizations and concepts.
- Natural language processing systems that can generate human language descriptions of design concepts.
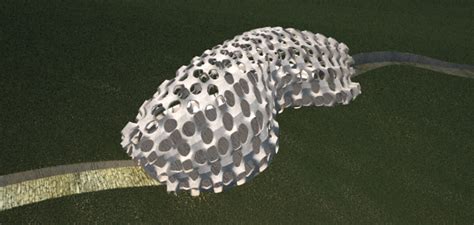
These advancements enable designers to push the boundaries of creativity and innovation while improving efficiency and reducing costs.
Applications of AI in Product Design
Integrating AI in industrial design has led to numerous practical applications transforming how products are conceived, developed, and manufactured. Let's explore some key areas where AI is making a significant impact.
Design Space Exploration and Optimization
Design Space Exploration is an upfront engineering segment that systematically investigates and evaluates various design alternatives to find optimal solutions for the product, i.e. new product designs. It encompasses exploring different design parameters, materials, and configuration combinations to achieve desired performance criteria while satisfying constraints. Combining many variables creates many possible navigation choices - this is the exploration part.
Mathematically, a design space is a multidimensional set of all possible design solutions for a given problem. Each dimension represents a design variable or parameter that can be adjusted in a space that can be inconceivably vast for complex products. It has nothing intuitively similar to our familiar 3D space, challenging in terms of computational resources and time any thorough exploration based on traditional methods.
Key aspects of Design Space Exploration include:
- Parameter Definition, i.e., identifying the relevant design variables and their possible ranges.
- Constraint Mapping, i.e., determining the limitations and requirements that viable designs must meet.
- Performance Evaluation, i.e., assessing how well each design alternative meets the specified objectives.
- Trade-off Analysis, i.e., understanding the relationships between different design parameters and their impact on overall performance.
Optimization in this context refers to finding the best possible design or set of designs within the explored space.
This often involves balancing multiple, sometimes conflicting, objectives such as performance, cost, manufacturability, and sustainability.
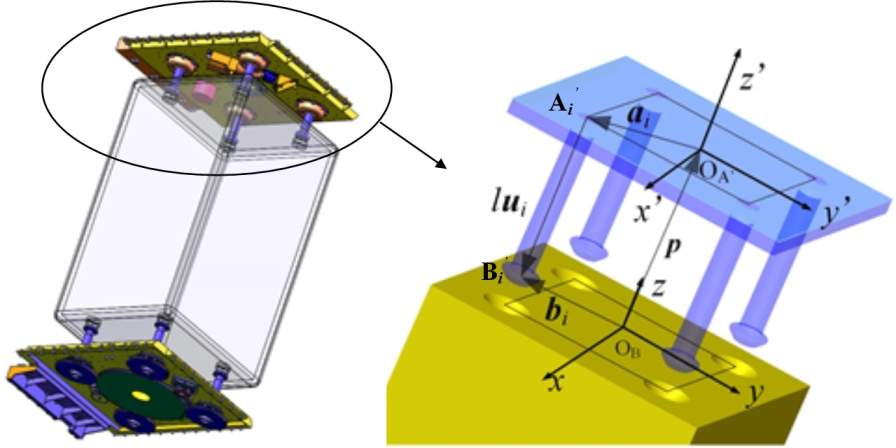
Traditional approaches to DSE and optimization often rely on techniques like:
- Design of Experiments (DoE)
- Response Surface Methodology (RSM)
- Gradient-based optimization methods
- Genetic algorithms and evolutionary strategies
However, these methods can be time-consuming and may not always capture the full complexity of the design space, especially for highly nonlinear problems or those with many variables.
How AI Speeds Up Design Space Exploration and Optimization
Artificial Intelligence, particularly machine learning and 3D deep learning techniques, is already significantly accelerating the Design Space Exploration and Optimization process.

Here's how AI can enhance this crucial aspect of industrial design:
- Efficient Sampling: AI algorithms can intelligently sample the design space, focusing on areas likely to yield promising results. A targeted exploration reduces the design iterations required to find optimal solutions.
- Surrogate Modeling: AI can create accurate surrogate models or meta-models that approximate the behavior of complex systems. These models can be evaluated much faster than running full simulations, allowing for rapid design space exploration.
- Multi-objective Optimization: Advanced AI algorithms handle multi-objective optimization problems, quickly identifying Pareto-optimal solutions that balance competing design goals.
- Pattern Recognition: AI can identify patterns and relationships in the design space that may not be apparent to human designers, leading to innovative solutions and unexpected insights.
- Adaptive Search Strategies: Machine learning algorithms can adapt their search strategies based on previous results, continuously improving their exploration efficiency as they gather more data.
- High-dimensional Space Navigation: AI can effectively navigate high-dimensional design spaces that would be impractical or impossible for humans to explore manually.
- Integration of Historical Data: AI systems can leverage historical design data and expert knowledge to inform and accelerate the exploration of new design spaces.
- Real-time Optimization: With AI, designers can perform real-time optimization, adjusting design parameters on the fly based on immediate feedback and changing requirements.
- Constraint Handling: AI algorithms can efficiently handle complex constraints, ensuring that explored designs remain within feasible regions of the design space.
- Uncertainty Quantification: AI methods can incorporate uncertainty analysis into the exploration process, providing more robust and reliable optimization results.

Generative Design
Within AI for industrial design, generative design represents the deployment of AI algorithms to explore various design possibilities, based on constraints and objectives imposed by designers. Generative design enables the designer to create products with optimized performance, reduced material usage, and innovative forms that may not have been conceivable through traditional design methods.
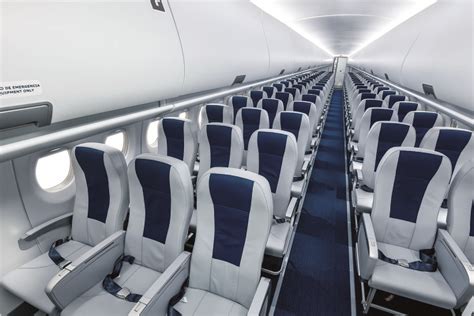
For example, in an Autodesk Research Group project, additive manufacturing with metal casting creates an airplane seat frame that is 54% lighter while maintaining structural integrity, according to Autodesk. This not only reduced material costs but also improved fuel efficiency.
Virtual Prototyping and Simulation
AI-enhanced virtual prototyping and simulation tools allow industrial designers to test and refine their concepts in a digital environment before moving to physical prototypes.
Let us list four key benefits of simulation:
- Reduce time and cost compared with physical prototyping
- iterate and test multiple design variations
- improved accuracy in predicting product performance
- easier data sharing and collaboration among design team members (e.g., simulation with CAD).
Companies like Siemens or Dassault Systèmes offer advanced physics-driven, high-fidelity simulation software that enables designers to evaluate the performance of their products under various conditions, from structural integrity to fluid dynamics, using best-in-class simulation tools.
Design Optimization
AI algorithms are crucial in optimizing designs for better performance, cost efficiency, and manufacturability. By analyzing extensive datasets and considering multiple variables simultaneously, AI can suggest design modifications that human designers might overlook.
Some key techniques in AI-driven design optimization include:
- Topology optimization: Refining the structure of a product to achieve maximum strength with minimum material usage.
- Parametric optimization: Fine-tuning design parameters to achieve optimal performance.
- Multi-objective optimization: Balancing multiple, often conflicting, design goals.

Customization and Personalization
The growing demand for personalized products has led to the development of AI tools that can quickly generate customized designs based on individual user preferences and requirements. This trend is particularly evident in the fashion, consumer electronics, and automotive design industries.
AI's role in customization includes:
- Analyzing user data to predict preferences and suggest personalized designs
- Generating multiple design variations based on user inputs
- Optimizing manufacturing processes for small-batch or one-off production
For example, Nike uses AI-powered design tools to create customized shoes based on individual customer preferences and biomechanical data, a so-called "private garden" of data exclusive to Nike.
Predictive Analytics
AI-driven predictive analytics transforms how industrial designers anticipate market trends and consumer preferences. By analyzing big datasets from various sources, including social media, sales figures, and user feedback, AI algorithms can provide valuable insights to inform the design process.
In general, implementation examples include:
- Trend forecasting: Predicting upcoming design trends and consumer preferences
- User behavior modeling: Understanding how consumers interact with products to inform future designs
- Product lifecycle analysis: Anticipating how products will perform in the market over time
- Advanced predictive analytics platforms that industrial designers can leverage to make data-driven decisions throughout the design process. A specific, design process-oriented approach is that from Neural Concept, targeting CAD (3D drawing) and simulation data that are available in huge quantities, usually stored in company vaults, and accessible via PLM (product lifecycle management) environments.
Benefits of AI in Industrial Design
Integrating AI in industrial design offers numerous benefits that are transforming the industry. Let's explore some of the key advantages:
Increased Efficiency
AI tools can significantly reduce the time required for various design tasks, from concept generation to design refinement. By automating repetitive processes and quickly generating multiple design options, AI is enabling designers to focus on more creative and strategic aspects of their work.
AI algorithms can optimize resource allocation by predicting material requirements, estimating production costs, and identifying potential bottlenecks in the design process, leading to more efficient use of time, materials, and human expertise.

Innovation and Creativity
AI creates new possibilities for creativity by generating design concepts that human designers may not have considered. This can result in groundbreaking innovations and unique product features that distinguish companies from their competitors.
AI tools promote collaboration between human designers and machine intelligence, creating a synergy between the two. While AI can handle complex calculations and data analysis, human designers bring intuition, emotional intelligence, and a deep understanding of user needs to the creative process.
AI can help cut production costs by optimizing designs for manufacturability and efficiency, which includes minimizing material waste, streamlining assembly processes, and identifying cost-effective manufacturing methods.
AI-driven generative design algorithms and optimization techniques can create products that use less material while maintaining or improving performance, ultimately reducing costs and contributing to sustainability efforts.
AI provides industrial designers valuable insights from large datasets, enabling them to make more informed decisions throughout the design process. This data-driven approach can lead to better product performance, improved user satisfaction, and increased market success.
AI tools can offer real-time feedback on design choices, allowing designers to quickly iterate and refine their concepts. The iterative process helps identify potential issues early in the design phase, reducing the likelihood of costly revisions later in the product development cycle.
Challenges and Considerations
Sure, AI offers numerous benefits, but it also presents challenges and considerations that must be addressed. Using AI in design raises questions about intellectual property rights, especially when AI generates new design concepts. Clear guidelines and legal frameworks are needed to address ownership and attribution of AI-generated designs.
AI technologies still cannot "understand" complex design contexts and subjective aesthetic preferences. In this sense, Neural Concept's perception-oriented approach is a breakthrough, using CAD 3D data instead of images to support technical decision-making.
However, taking a step back and looking at the AI landscape in general suggests that overreliance on AI-generated solutions may lead to design homogenization or miss important cultural and emotional factors.
Integrating AI in industrial design requires designers to develop new skills and adapt to new working methods. Continuous education and upskilling are needed to ensure that designers can effectively leverage AI tools. Maintaining the right balance between AI assistance and human creativity is crucial to creating innovative and meaningful designs. AI algorithms are only as good as the data they're trained on. Ensuring high-quality, diverse, and unbiased data sets prevents AI from perpetuating biases or making flawed design recommendations. Incorporating AI tools into existing design workflows and software ecosystems can be complex and require significant investment in technology and training.
As AI becomes increasingly embedded in the design process, there's a need for greater transparency in how AI algorithms make decisions. This transparency is crucial for building trust among designers, stakeholders, and end-users.
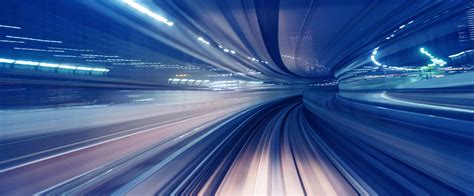
Future of AI in Industrial Design
The future of AI in industrial design looks promising, with several emerging trends and potential long-term impacts:
Emerging Trends - Material Selection
AI is expected to be crucial in developing new materials with specific properties tailored for particular applications.
AI algorithms will increasingly focus on optimizing designs for sustainability, considering factors like recyclability, energy efficiency, and lifecycle impact.
Future AI systems may be able to understand and incorporate emotional and psychological factors into design decisions, leading to products that better resonate with users on an emotional level.
We may see the development of AI systems that can autonomously handle entire design projects, with human designers taking on more supervisory and strategic roles.
Long-Term Impact
The long-term impact of AI on industrial design is likely to be profound:
- Democratization of Design: AI tools may make advanced design capabilities more accessible to a broader range of people, potentially disrupting traditional design education and career paths.
- Hyper-Personalization: As AI becomes more sophisticated in understanding individual preferences, we may shift towards mass customization and hyper-personalized products.
- Interdisciplinary Collaboration: AI may facilitate closer collaboration between industrial design and other fields, such as materials science, psychology, and data analytics.
- Redefined Role of Designers: Industrial designers may evolve to focus more on strategic thinking, problem framing, and ethical considerations as AI takes over more routine design tasks.
- Accelerated Innovation: AI-driven design processes could significantly speed up product development cycles, leading to faster innovation and more responsive product evolution.

Conclusion
AI industrial design represents a change in the way we approach product creation and innovation. By harnessing the power of artificial intelligence, industrial designers can develop exceptional products that are more efficient, sustainable, and user-centric than ever before. Combining human creativity and AI capabilities creates new approach to business and design challenges and expands the boundaries of what is possible - "the only limit is imagination".
AI will continue to play an increasingly important role in industrial design. The integration of artificial intelligence in engineering design for example, will revolutionize how designers optimize processes, predict performance outcomes, and enhance product functionality. However, AI is a tool to enhance human creativity, not replace it. The most successful applications of AI in industrial design will strike a balance between technological innovation and human insight.
Industrial designers who embrace AI technologies and build the skills to use these tools effectively will be well-equipped to lead the industry into a new era of innovation. AI will undoubtedly bring new challenges and ethical considerations. Still, thoughtful implementation and a focus on human-centered design principles will speed up the evolution of industrial design, creating a future where products are more functional, efficient, sustainable, and responsive to human needs.
AI industrial design is not just a "passing trend" but a fundamental shift in our approach to product development. Designers will create products once thought impossible, solve complex problems more effectively, and shape a better future.