AI in Civil Engineering: Applications & Advancements
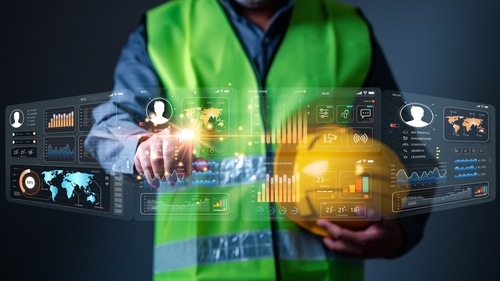
Structural analysis and design, urban comfort predictions, construction management, geotechnical engineering, and smart infrastructure development are just a few civil engineering applications where Deep Learning can offer solutions!
What is Deep Learning?
It is an AI branch based on neural networks that learn from data instead of being programmed with precise instructions. Given the huge amount of Civil Engineering data and data science maturity, Deep Learning is now mature enough to be considered a preliminary design tool for civil engineering structures, especially high-budget mission-critical works such as skyscrapers or bridges.
This article will focus on the AI applications for civil engineering and the promising applications of structural analysis engineering.
In the construction engineering preliminary concept "what-if" phase, AI can make more alternatives available for preliminary exploration, especially when time-consuming aerodynamic and internal fluid dynamic simulations are requested in civil engineering applications.
While AI-based engineering does not replace sophisticated, cutting-edge high-fidelity simulation (CAE) for civil engineering, it helps time-consuming CAE focus on designs preliminary explored by Deep Learning in a design space that could be 1000 to 100.000 times wider than usual.
Thus, the approach of Artificial Intelligence Engineering represents a complement rather than a replacement of consolidated methods.
Summary of Benefits of Simulation and AI in Civil Engineering
In analysis and design, FEM has revolutionized civil engineering; by employing intelligent algorithms, it enabled civil engineers to perform project analysis and use more precise and sophisticated 3D models.
Recent breakthroughs showcase enhanced accuracy, data quality, and efficiency, promising a new era in civil engineering thanks to FEM (and CFD).
AI-driven optimization algorithms feed on the previous high-quality data and help with efficient solutions that enhance structural design efficiency and performance. This application of AI in structural engineering significantly improves design outcomes and streamlines design processes within civil engineering.
General Benefits of AI in Civil Engineering
Before exploring simulation applications (FEM or CFD) of Artificial Intelligence, let us review some more "general purpose" applications in civil engineering.
Efficient Project Planning in the Construction Industry
Artificial Intelligence tools facilitate efficient project planning and scheduling by employing advanced project analysis and optimizing resource allocation and timeline management for civil engineers. Artificial Intelligence-driven project management platforms like Procore and Autodesk BIM 360 utilize historical project data and machine learning algorithms to predict project timelines and identify potential bottlenecks in the construction industry. These platforms analyze past project performance data, including weather conditions, material availability, and workforce productivity, to generate more accurate schedules and mitigate risks.
Moreover, Artificial Intelligence-based scheduling algorithms dynamically adjust project timelines based on real-time data inputs, such as unexpected delays or changes in resource availability. For example, in infrastructure projects, Artificial Intelligence-powered scheduling software continuously optimizes the allocation of resources, such as equipment and labor, to ensure that work proceeds efficiently and critical milestones are timely met.
Additionally, artificial intelligence-enabled project planning tools enhance collaboration among project managers and teams. Platforms like Asana or Trello, to name a few, use AI algorithms and data to streamline workflows and help teams coordinate and stay on track with project goals.
Risk Identification with Artificial Intelligence
AI algorithms excel at identifying risks and predicting maintenance requirements during construction projects. They leverage historical data for informed decision-making, predictive maintenance, and proactive risk mitigation.
For instance, in the construction of a bridge, engineers can use Artificial Intelligence-powered risk identification tools to analyze vast amounts of historical data from similar bridge projects worldwide. The AI system identified potential risks such as corrosion, material fatigue, and seismic vulnerabilities by examining past failures, weather patterns, and construction methodologies. This enabled engineers to implement proactive measures such as enhanced corrosion-resistant coatings, reinforced structural elements, and real-time structural health monitoring computer systems to mitigate these risks effectively.
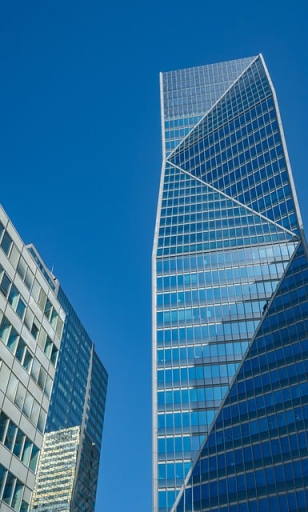
Moreover, AI algorithms can predict maintenance requirements for the building's elevators and HVAC systems when constructing a skyscraper. The Artificial Intelligence system forecasts when components require servicing or replacement by analyzing data from similar high-rise buildings and considering usage patterns, equipment age, and environmental conditions. This proactive maintenance approach minimized downtime and operational disruptions, optimized the building's long-term performance, and reduced lifecycle costs.
Additionally, Artificial Intelligence-driven risk identification tools are increasingly used in large-scale infrastructure projects such as railway expansions and highway constructions.

In a high-speed train project, AI algorithms analyze geological data, construction progress, and environmental factors to identify potential safety hazards, such as ground settlement, tunnel collapses, and ecological impacts. This allows project managers to take preemptive measures, such as adjusting construction methods or reinforcing critical areas, to mitigate these risks and ensure the project's successful completion within budget and schedule.
Geotechnical Engineering
AI enhances geotechnical engineering by improving the accuracy of soil analysis and enabling better decisions through precise data interpretation and prediction.
Artificial Intelligence-powered monitoring systems coupled with IoT (Internet of Things) can continuously assess ground conditions, detecting subtle shifts that may indicate impending instability.
Furthermore, Artificial Intelligence aids the construction sector in landslide detection and prevention by analyzing geospatial data to recognize patterns and contribute to safer infrastructure development.
Smart City Development with Artificial Intelligence
Artificial Intelligence's integration into smart city development optimizes resource usage and enhances urban services. It also fosters sustainable growth, making smart cities more livable, resilient, and efficient.
Artificial Intelligence plays a crucial role in smart city development by orchestrating smart systems and integrating IoT technologies to optimize resource usage and enhance urban services for sustainable growth. For instance, AI-driven energy management systems analyze real-time data from smart meters and sensors to optimize electricity distribution, reducing waste and lowering costs for consumers and utility providers. In Barcelona, Spain, the CityOS platform (for more information, see: https://ajuntament.barcelona.cat/imi/en/projects/city-os) utilizes Artificial Intelligence to monitor energy consumption, water usage, and waste management, enabling city officials to make data-driven decisions for sustainability.
Moreover, AI algorithms analyze traffic patterns, predict congestion, and optimize transportation systems to alleviate traffic congestion and reduce emissions. For example, Singapore's Smart Nation initiative employs AI-powered traffic management systems that dynamically adjust traffic signals based on real-time traffic flow data, easing congestion and improving travel times. Additionally, AI-driven predictive maintenance systems monitor the condition of infrastructure assets such as bridges and roads, enabling proactive maintenance to prevent costly failures and ensure public safety.

Furthermore, Artificial Intelligence supports urban planning initiatives by analyzing demographic data, environmental factors, and economic indicators to inform land use, zoning, and infrastructure development decisions. In Songdo, South Korea, urban planners leverage Artificial Intelligence models to simulate the impact of proposed developments on traffic flow, air quality, and energy consumption, ensuring that new projects align with the city's sustainability goals and enhance residents' quality of life.
Advancements in AI within civil engineering
Advancements in AI within civil engineering include predictive modeling, automated quality control, machine learning algorithms, and natural language processing for communication with human workers.
These innovations foster innovation and efficiency in project execution and construction processes. Predictive modeling techniques enable accurate prediction of project outcomes, material behavior, and structural performance, driving informed decision-making in civil engineering projects.
Additionally, AI-based automated quality control improves construction process monitoring data analysis and inspection, ensuring adherence to standards and safety protocols and delivering higher-quality infrastructure.
AI-powered communication tools, like virtual assistants and chatbots, enhance stakeholder collaboration through NLP, streamlining communication and efficient project management and coordination.
Predictive Modelling for Civil Engineering - FEA Approach and Use Cases
As a classic example of the significant impact of predictive models based on Finite Element Analysis (FEA), the stress distribution in a bridge under different traffic loads can be simulated to identify potential failure points and optimize structural reinforcement.
FEA in Short
FEA software is a tool that predicts stress distribution, deformation, and failure points in structures such as buildings, bridges, and dams. Engineers use FEA to assess structural integrity under various loading conditions, optimizing design for enhanced performance and safety.
While engineers and architects were used in the past to carry out dynamic and static structural analysis projects via manual calculations for simple structures, nowadays, for more complex problems, they can leverage either "classic" FEA software (based on physics) or new AI-enabled tools (based on data generated by FEA or experimental records).
Construction Material Behavior
FEA also assists in predicting the behavior of construction materials, such as concrete, steel, and composites, under different loading scenarios.
Engineers can optimize material selection and design decisions for durability and performance by simulating construction material properties and responses. FEA is utilized to simulate the behavior of reinforced concrete beams under varying loads, predicting crack propagation and deformation patterns and informing construction material selection and reinforcement strategies.
Geotechnical and Seismic Analysis
FEA also supports geotechnical analysis by simulating soil-structure interaction, slope stability, and foundation performance. Engineers use FEA to predict soil behavior under different loading conditions, guiding foundation design, maintenance management, and risk mitigation strategies.
Finite Element Analysis facilitates seismic analysis and risk assessment for structures in earthquake-prone regions. Engineers use FEA to simulate seismic loading scenarios, predict structural response, and assess the vulnerability of buildings, construction sites, and infrastructure to earthquakes.

Predictive Modelling for Civil Engineering - AI Approach and Use Cases
Computational Fluid Dynamics (CFD) utilizes numerical methods to solve fluid flow and heat transfer equations in civil engineering to provide valuable insights into airflow around buildings, water flow in hydraulic structures, and pollutant dispersion in urban environments.
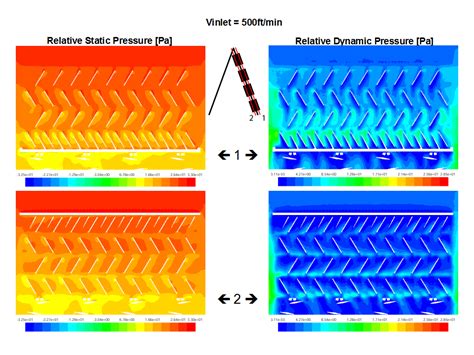
Application - HVAC
CFD-based predictive modeling assists in the design of ventilation systems and HVAC (Heating, Ventilation, and Air Conditioning) systems for buildings. Engineers can simulate airflow patterns, temperature distribution, and indoor air quality to optimize system design for comfort and energy efficiency. CFD is used to analyze airflow patterns in a building's atrium to optimize natural ventilation strategies and reduce the need for mechanical cooling.
Application - Hydraulic Structures and Flood Modelling
CFD facilitates water flow analysis in hydraulic structures such as dams, weirs, and channels. Engineers can simulate water flow patterns, sediment transport, and scouring effects to optimize the design and operation of hydraulic and infrastructure projects. CFD is employed to simulate water flow around a bridge pier to assess scouring potential and design suitable protective measures to mitigate erosion risks.
Application Examples of CFD in Hydraulic Structures and Flood Modelling
CFD-based predictive modeling aids in flood modeling and the risk management and assessment for flood-prone areas to avoid risk for construction projects or to monitor existing structures. Engineers can simulate rainfall-runoff processes, river flow dynamics, and floodplain inundation to assess flood risk and develop mitigation strategies. CFD simulates river flow and floodplain inundation during extreme rainfall to identify flood-prone areas and plan flood protection measures such as levees and floodwalls. Data-driven deep Learning can help high-fidelity CFD with preliminary real-time analysis.
The nonparametric nature of NCS (Neural Concept Shape) based on artificial neural networks makes it ideal to read highly unstructured data, for instance, from Digital Elevation Maps (DEM).
It has the potential for a significant impact in the future!

Conclusion - Future Artificial Intelligence Apps in the Construction Sector
In summary, we have seen how Artificial Intelligence offers advanced solutions in civil engineering, from structural analysis to smart infrastructure development. Deep Learning is becoming a preliminary design tool for civil engineers, expanding design exploration and optimizing structural efficiency during construction. AI-driven risk identification enhances project safety, and AI-based project planning tools streamline scheduling and resource allocation, ensuring project success in construction management.
Given the real-time response based on input data and not requiring sophisticated FEM or CFD skills, it is possible to envisage future Artificial Intelligence apps made available not only to civil engineers but also to architects and regulatory authorities to answer preliminary questions during the concept phase of urban planning, either in the office or in construction sites.