Aerospace Parts Manufacturing and AI: The Efficiency Guide
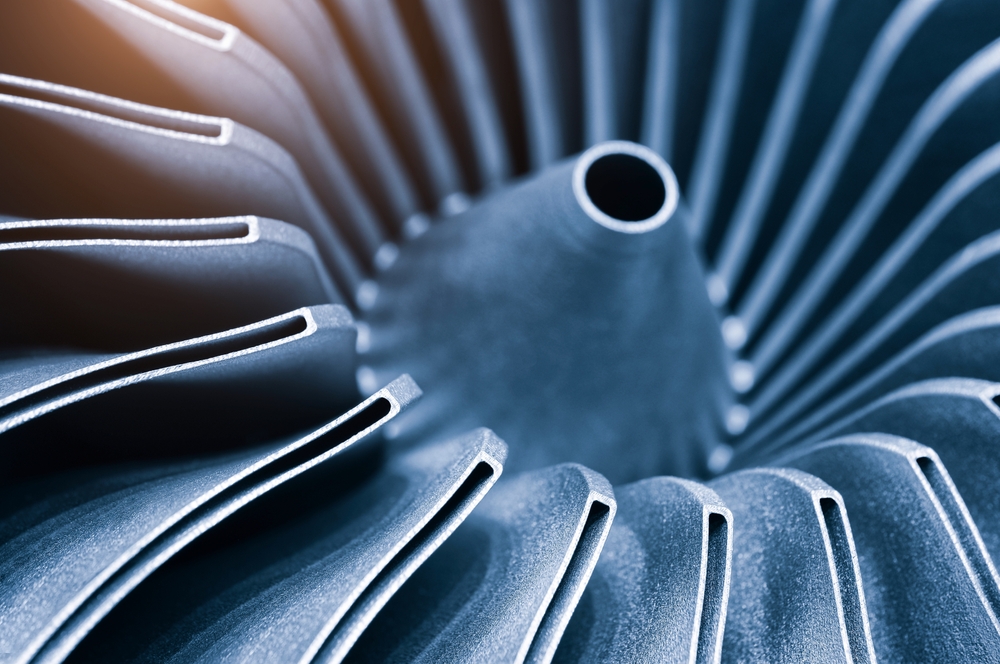
Artificial intelligence (AI) offers engineers groundbreaking tools for designing, manufacturing, and assembling components. This exploration will highlight AI’s impact, from the design of aircraft components to their manufacturing process.
In the design phase, AI integrates with 3D simulation.
AI-driven automation streamlines production lines, improving precision and reducing human error. Aerospace parts manufacturers use machine learning algorithms to enhance quality controls and leverage AI in every production stage.
AI can help Aerospace manufacturing companies with everything from material selection to post-production inspection. AI-powered systems provide real-time monitoring, ensuring that production processes meet the industry's rigorous standards.
Let us start our exploration by defining the aerospace manufacturing process and its difference from other industries.
What is The Aerospace Manufacturing Process?
The aero industry needs to transform raw materials into high-precision, finished components using advanced machinery, skilled labor, and cutting-edge technology.
This table compares production processes across key factors like precision, materials, production volume, and regulations. The aero industry stands out for its extreme need for precision, specialized materials, and strict compliance requirements. The automotive, electronics, and biomedical sectors prioritize scalability, cost-efficiency, and material adaptability.

Manufacturing processes includes various specialized techniques, such as casting for complex, large parts, machining for fine-tuning dimensions, forming for shaping metals, and welding for strong, durable joints. Additive manufacturing (3D printing) is used for lightweight, intricate components, while assembly integrates parts into larger systems. Finally, surface treatment processes like coating or heat treatment enhance material properties, ensuring parts meet the strict safety and performance standards required in the aerospace industry.

Manufacturing integrates multiple systems and technologies, such as automation, robotics, and quality control.
These systems work together to deliver three ìkey benefits. Fast production cycles are carried out by automating repetitive tasks, enabling rapid production of high-volume components with minimal downtime. Uniform product quality is guaranteed through precise robotic control and continuous quality monitoring, ensuring each component meets strict standards. Exact component specifications are obtained with automated measurement systems that verify dimensions and tolerances, ensuring every part is produced aligned to design requirements, reducing the risk of defects or rework.
What is Aerospace Manufacturing
As shown before, the industry requires extreme precision, high-performance materials (e.g., titanium, composites), innovative materials, and strict safety regulations.
Specific processes include advanced machining, additive manufacturing, and non-destructive testing.
Aerospace parts (components) must withstand high stress, temperature extremes, and rigorous quality standards. AS9100, a standard based on ISO9001, adds specific features for the aerospace parts manufacturing market, such as safety, reliability, and regulatory compliance.

Aerospace Parts Manufacturing
Aerospace parts manufacturing is a sector that specializes in producing aerospace components and systems. Critical components ensure safety, performance, and longevity.
The market has grown as the demand for advanced aerospace parts continues to rise. Aerospace components manufacturers and companies are at the forefront of innovation, using advanced technologies and materials to create reliable, high-performance aerospace parts that meet strict regulatory standards.
This includes aircraft and spacecraft safety, performance, and efficiency components.
Key elements include heat exchangers for temperature regulation, manifolds that distribute fluids to different systems, and turbo pumps that pressurize fuel for engine performance.

Fuel nozzles control fuel flow for optimal combustion, while conformal cooling channels are integrated into parts to improve heat dissipation in high-performance systems like turbines.
In addition, structural components such as fuselage frames, wings, and empennage parts maintain the aircraft’s structural integrity.
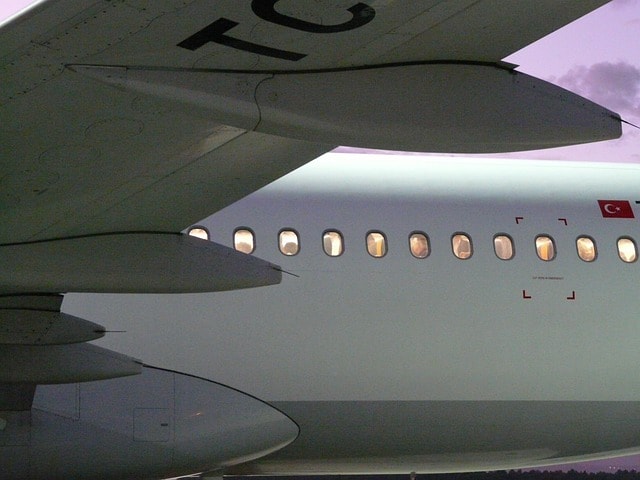
Landing gear systems, which incorporate hydraulic actuators and shock absorbers, are vital for enduring extreme forces during takeoff and landing. Control surfaces, including elevators, rudders, and ailerons, are crucial for maneuverability and stability.
Avionics components, such as sensors, navigation systems, and communication equipment, guarantee the safe operation of aircraft.
Furthermore, engine parts like turbine blades, combustion chambers, and compressor stages are constructed from high-performance materials that can withstand extreme temperatures and pressures.
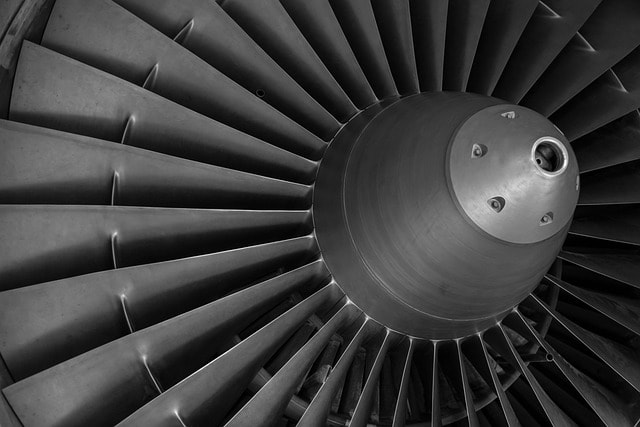
Collectively, these components create intricate systems. Each requires precise design, testing, and integration to meet the rigorous safety and performance standards required in aviation and space exploration.
Short History
In the early 20th century, aircraft were made of wood, fabric, and basic engines. As technology progressed, metal replaced wood, with riveted aluminum as the standard. World War II sped up mass production and assembly-line techniques, increasing speed, lowering costs, and standardizing bombers and fighters for large-scale operations. Post-war, jet engines required titanium and high-temperature alloys, while precision machining and automation improved processes. The 1960s Space Race introduced ultra-lightweight materials, cleanroom assembly, and strict quality control, shaping current standards.
Digital twins, AI-driven design, and automation have enhanced operational productivity and resource optimization. Companies like SpaceX and Boeing leverage rapid prototyping to reduce design cycles and costs while utilizing reusable components to minimize material waste and maximize part lifecycles. This results in faster time to market, lower production costs, and improved resource allocation, directly impacting aerospace operations’ overall effectiveness.
Current Status of Aerospace Parts Manufacturing Market
The aerospace parts manufacturing market is expected to rebound after recovering from the supply chain and production disruptions caused by the COVID-19 pandemic.
In 2023, the global aerospace and defense industry generated approximately $829 billion in revenue, marking an 11% increase from the previous year. Rising air passenger numbers and the demand for new aircraft and components primarily drive this growth.
Key drivers in the aviation industry are:
Rising Air Passenger Traffic
The aviation sector has seen a rebound in passenger traffic, increasing airline revenues and demand for new aircraft to accommodate the growing number of travelers.
Fleet Expansion and Modernization
Airlines are investing in new aircraft to replace aging fleets and to meet environmental regulations. This trend is expected to continue, with the worldwide commercial aviation fleet projected to expand by 33% to over 36,000 aircraft by 2033, reflecting a compound annual growth rate of 2.9%.
Technological Advancements
Innovations in aircraft design and manufacturing processes have led commercial airlines to more fuel-efficient and environmentally friendly aircraft, attracting both airlines and passengers.
Military Aircraft Market Share
The military aircraft market has experienced notable shifts in the post-COVID-19 era. In 2024, it is projected to be valued at approximately USD 96.24 billion, with a CAGR of 4% from 2022 to 2031.
Industry Challenges in Aerospace Component Manufacturing and How AI Solves Them
Aerospace manufacturing demands micron-level accuracy, optimization of production workflows, and rigorous cost management. Components must meet strict tolerances to ensure safety, performance, and durability, requiring advanced techniques like precision machining and laser cutting. At the same time, manufacturers must minimize material waste, reduce labor costs, and improve throughput without compromising quality, which drives the use of AI and automation in aerospace industry, and advanced materials to achieve cost-effective, high-performance solutions.
- Material Waste. Aerospace materials like titanium and composites are costly, and traditional machining creates waste. AI predictive modeling optimizes material use by enhancing cutting paths and reducing scrap. AI-driven 3D printing minimizes waste by layering materials precisely where needed.
- Supply Chain Optimization. Global supply chains face disruptions and inventory challenges. AI forecasts demand, analyzes delays, and suggests suppliers in real-time. Machine learning optimizes inventory, reducing excess and preventing shortages. Digital twins simulate scenarios to improve logistics.
- Production costs stem from labor, material prices, and inefficiencies. AI automation reduces labor expenses, predictive maintenance minimizes downtime, and quality control algorithms identify defects early, cutting rework.
AI in the Design Stage for Aerospace and Defense Industries
Generative design algorithms integrate with AI to optimize aerospace component design in lean manufacturing. They use computational power to explore extensive design spaces, producing innovative, manufacturable components.
“Design space exploration” begins with defining parameters and constraints for an aerospace component. AI generates and evaluates numerous design iterations, allowing engineers to explore potential solutions beyond their typical limitations.
Generative Design: Algorithmic Insights for the Aerospace Sector
AI enhances generative design via Machine Learning, which is crucial for precision engineering in aerospace components. The process starts by inputting data into ML algorithms, including design history, materials, and performance criteria. The algorithm uncovers patterns and correlations between design variables and performance outcomes.
Algorithmic Exploration of Aerospace Parts’ Design Space
The AI algorithm explores numerous design iterations based on the specified criteria. Each generated design of aerospace parts undergoes a rigorous evaluation against performance metrics and mechanical properties such as structural integrity and aerodynamic performance. Through an iterative refinement process, the AI algorithm converges on optimal solutions.
AI in Aerospace Manufacturing Processes
AI and Additive Manufacturing (AM) are linked through optimized designs generated by AI algorithms. This combination is promising and can lead to exciting manufacturing advancements. AI-driven generative design replaces traditional surfaces with intricate, nonintuitive geometries that enhance, for instance, performance in external aerodynamics.
The combination of AI and Additive Manufacturing enables the production of highly customized, lightweight, and structurally efficient materials and components for the aerospace industry, such as intricate heat exchangers with optimized thermo-fluid performance and reduced weight.
Aerospace Parts Manufacturing: Robotic Assembly Lines and Cobots
Aerospace companies utilize AI-driven robotic arms for drilling and riveting, decreasing production time. Quality is essential in manufacturing. A collaborative robot (cobot) with ML capabilities learns from a dataset of high-quality components by analyzing key features. While collaborating with human workers, the cobot monitors production parameters like dimensions and surface finish. ML algorithms detect deviations from standards and make real-time corrections, adjusting parameters or alerting operators as necessary.
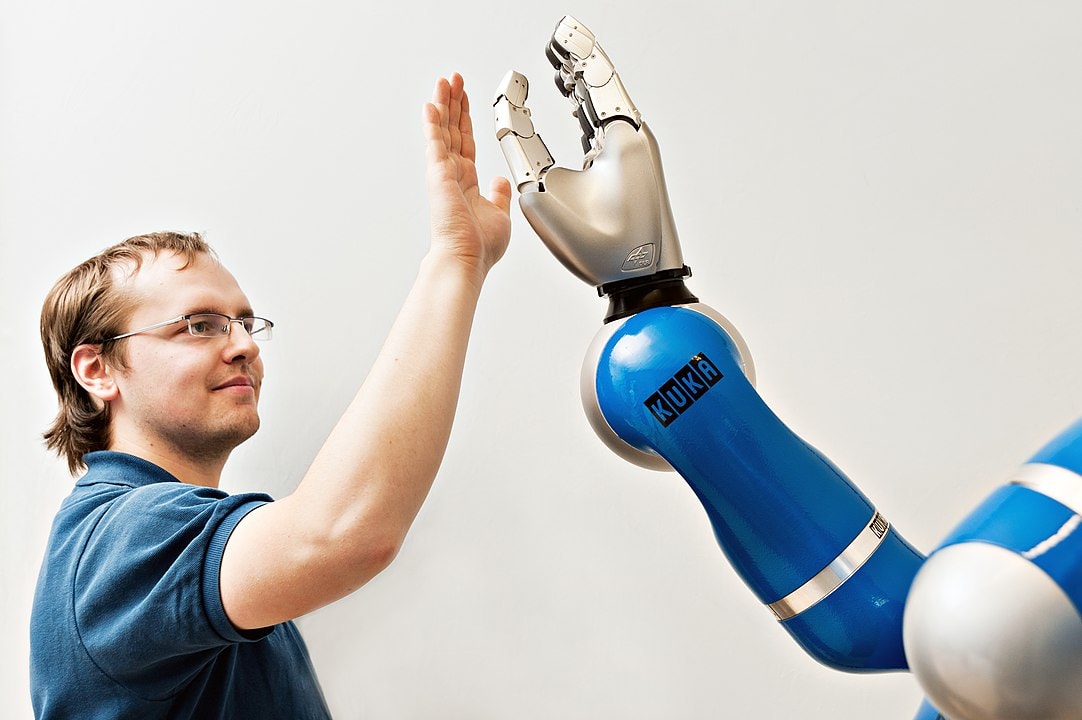
Over time, the ML model on the cobot keeps learning from new data. This allows it to adjust to subtle changes in processes, materials, or product specifications, contributing to a continuous improvement cycle.
Materials for Aerospace Parts Manufacturing
Stainless steel is used in the aerospace industry due to its high strength and good corrosion resistance.
Titanium is a lightweight and strong material with excellent corrosion and temperature resistance, making it a go-to for aerospace components.

Aluminum has a high strength-to-weight ratio, which makes it suitable for housing and brackets that must support high loading in aerospace applications.
Inconel is a nickel-chromium superalloy ideal for rocket components requiring high-temperature resistance. Examples of rocket engine components include turbine blades, combustion chambers, nozzle components, and exhaust nozzles.
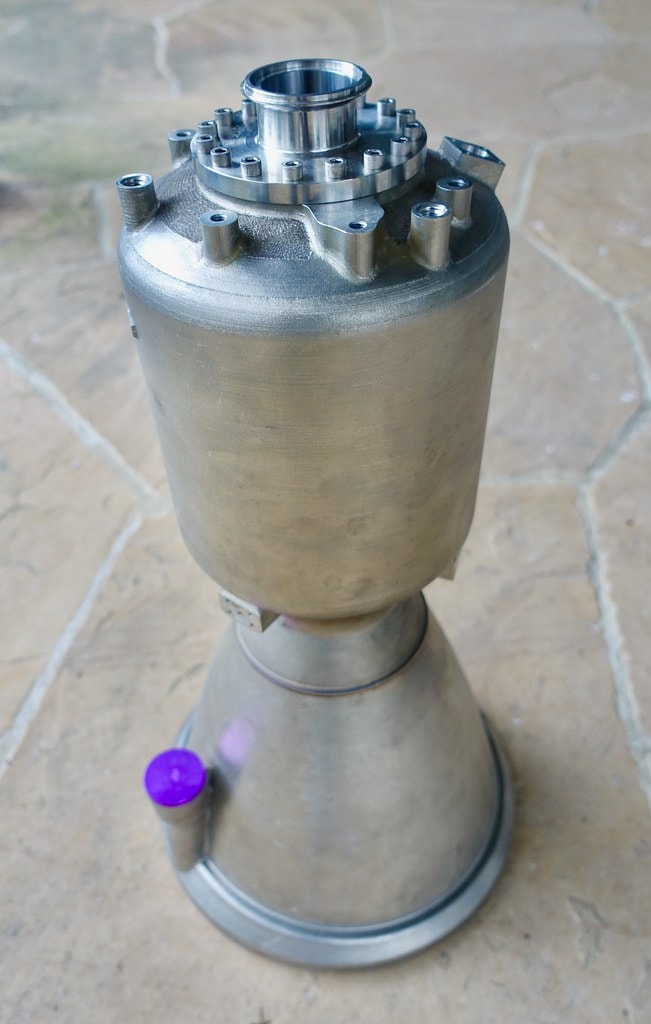
Composite materials, such as carbon-fiber composites, reduce aircraft weight and enhance fuel efficiency because they offer a higher strength-to-weight ratio than traditional metals. Manufacturers can create lighter, strong, and durable structures. Composites resist corrosion, reducing maintenance. They withstand high stress and fatigue, improving aircraft longevity and performance. Reduced weight and durability lower fuel consumption and operational costs.
Nanocomposites integrate nanoparticles with traditional composite materials to achieve superior properties in aerospace applications.
Smart materials are deployed in sophisticated sensing and monitoring systems in aerospace. Smart aerospace materials are used in structures that can detect changes in stress, strain, temperature, or vibration, providing real-time data about the health of an aircraft or spacecraft. This capability helps in maintaining safety and performance over the lifecycle of these complex systems.
How AI is Transforming Aerospace Parts Manufacturing: Additive and Traditional Methods
3D printing in aerospace is optimizing aerospace product development for better manufacturability, improving material usage, and reducing production time. AI-driven design helps engineers identify efficient structures among various impractical designs. It predicts optimal printing parameters for better layer deposition and integrity, validating designs by simulating real-world performance and reducing costly prototypes.
AI-powered systems monitor real-time sensor data to detect defects during production, such as warping or structural inconsistencies in 3D-printed or traditionally manufactured aerospace systems or components. Adaptive control systems leverage this information to adjust printing or machining parameters automatically, ensuring high-quality outputs and reducing rework or material waste.
AI in Traditional Aerospace Component Manufacturing
AI improves aerospace component manufacturing by increasing accuracy, reducing cycle times, and enhancing throughput. Machine learning analyzes historical data to predict maintenance needs, prevent bottlenecks, and streamline operations while ensuring quality and reducing costs. AI adjusts CNC machining and forging by evaluating operational data. It predicts tool wear, optimizes speeds, and manages material flow, reducing errors and extending tool life, leading to better production cycles and quality control.
Key Benefits of AI with Case Studies
- Enhanced Quality & Precision. AI-driven defect detection uses machine vision and deep learning algorithms to analyze high-resolution images of components during production. These systems compare real-time data with pre-defined standards, identifying microscopic cracks or inconsistencies in materials. Machine learning models continuously improve by recognizing patterns from past defects, enabling early detection of potential failures. This approach ensures components meet standards, reducing rework and scrap while maintaining consistency across production batches.
- Improved Production Efficiency. AI optimizes production schedules and workflows. For instance, in collaboration with Accenture, Airbus explored AI-powered manufacturing solutions to transform operations. These solutions focused on automating the detection of assembly progress through computer vision, predicting delays, and dynamically adjusting workflows.
- Cost Reduction. AI-driven material optimization in aerospace analyzes material properties, part geometry, and production methods to select cost-effective materials. AI identifies the best components by evaluating, for instance, strength, weight, and cost. For example, Boeing uses AI to optimize sourcing, reducing excess inventory and waste. The AI-powered procurement platform, Tail Spend, automates sourcing for low-value, high-volume purchases. It analyzes spending patterns and suggests suppliers, streamlining procurement and reducing excess inventory.
- Faster Production Speed. AI-powered monitoring systems collect data from machines and equipment, analyzing it with machine learning to track production, detect anomalies, and predict issues. For instance, GE Aviation uses AI to monitor vibrations and temperature. If a potential fault is detected, it alerts operators to perform maintenance, preventing costly downtime. AI ensures smoother operations, accelerates production, and reduces time needed to launch new aircraft models.
GE Case Study in Manufacturing
General Electric (GE) Aerospace has integrated artificial intelligence (AI) into its manufacturing. In partnership with Microsoft, GE launched “Wingmate,” an AI tool that summarizes manuals and drafts documents. GE’s CIO stated that AI integration boosts productivity, safety, sustainability, and supply chain management.
QuesTek Case Study
QuesTek Innovations LLC, a materials engineering company, uses AI to transform aerospace component manufacturing. With Integrated Computational Materials Engineering (ICME), QuesTek designs innovative metal alloys for aerospace applications. This AI-driven approach predicts material performance, halving development time and reducing costs by over 70% compared to traditional methods.
Rolls-Royce Case Study for AI Inspection
Rolls-Royce has implemented AI-driven systems to enhance the manufacturing and inspection of turbine blades. For instance, it developed the "Intelligent Borescope," an AI-powered inspection tool that automates the detection of issues within engines. This technology reduces inspection times and minimizes human error by capturing high-quality, standardized data for analysis.
Future Trends in AI and Aerospace Component Manufacturing
Incorporating AI in the design of composite materials sparks innovations in aerospace manufacturing. AI algorithms evaluate data to refine composite formulations, enhancing strength-to-weight ratios and durability. This strategy yields lighter, more fuel-efficient aircraft and cost-effective components. When paired with Industry 4.0 principles, AI enables real-time oversight of composite fabrication processes, guaranteeing accurate material properties at every stage. This shift towards AI-driven material innovation aligns with the prevalent trend in digitalization, where materials science and advanced manufacturing processes are more connected for improved performance and sustainability.
Self-learning Manufacturing Systems
Self-learning systems mark a new era in autonomous production, aligning with Industry 4.0 and advancing toward Industry 5.0. They utilize AI and machine learning to optimize workflows, adapt to anomalies, and adjust to changing requirements. These AI-driven systems refine processes and predict failures autonomously. As Industry 5.0 focuses on human-AI collaboration, these systems foster a personalized production environment, enhancing flexibility and precision while reducing human error and costs.
Conclusion
AI transformed the aviation industry by helping unprecedented precise measurements minimizing waste and speeding up production. It also helps engineers to design better components with generative techniques, improving performance and assembly simplicity. Automation reduces errors and increases production speed, while real-time monitoring and predictive maintenance maintain high industry standards, lowering costs and quickening production. AI application will be essential for competitiveness across aerospace's commercial, defense, and space sectors.
FAQ
What are the challenges associated with implementing AI in aerospace manufacturing?
Integrating AI poses challenges, including high initial costs, the need for skilled personnel, compatibility with legacy systems, data quality issues, and cybersecurity risks. Maintaining AI accuracy in a regulated industry like aerospace can be complex.
Are there ethical concerns related to the use of AI?
Ethical concerns in aerospace AI include data privacy, biased decision-making, and accountability for AI actions. Addressing these issues to sustain public trust requires transparency, fairness, and regulatory compliance.
Can AI integration displace jobs?
AI automates tasks but will not fully replace jobs. Instead, it evolves roles toward higher-skill tasks like AI management and data analysis, encouraging workforce transition instead of elimination.
Are there any hand-made parts on the plane?
Some precision or custom components still require handcrafting. Cockpit instruments, intricate finishes, and specific composites often require craftsmanship to ensure top quality and customization.