In-graph Training Loop
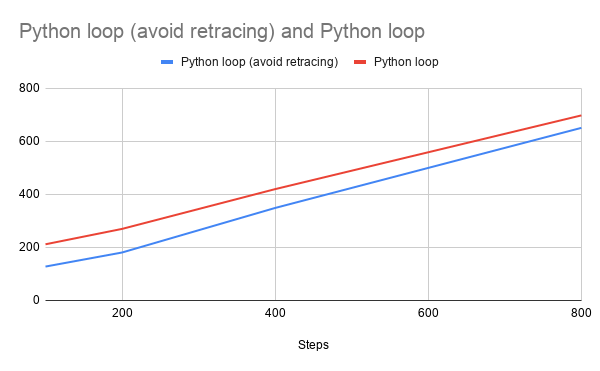
In a previous experiment, we explored the behaviour and interaction between keras models, tf.functions, saved models and tf.dataset. A summary of this experiment is available here as a notebook.
As a follow up to this previous test, we compared the performance of in-graph training loop ( https://www.tensorflow.org/guide/function#advanced_example_an_in-graph_training_loop ) with loop in python and model with annotated __call__ function.
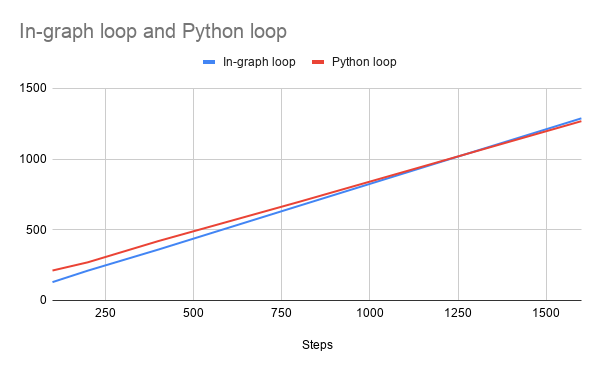
We performed training with 100, 200, 400, 800 and 1600 steps with the naval dataset with the following config –
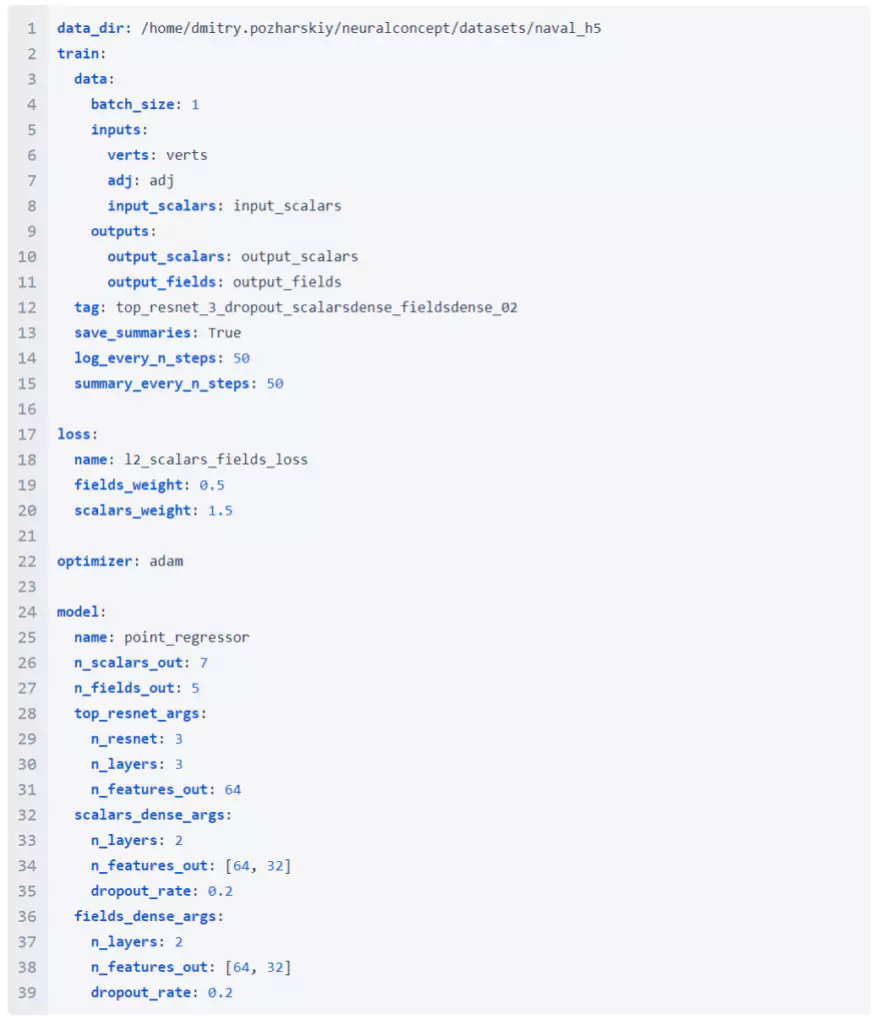
It is seen that the python loop has some upfront initial cost, but over the period of time it runs faster than in-graph training loop.
The initial cost of the python loop can be attributed to the fact that multiple traces are performed for each different shapes, but once all the different shapes are encountered, there are no further delays and it runs faster than the in-graph loop.
The finding also corroborates with the issue https://github.com/tensorflow/tensorflow/issues/35165
In order to avoid retracing for different shapes, we should provide input_signature to the model tf.function. The input_signature is known only after the partial shape information is available, so instead of statically annotating with tf.function, we need to create a tf.function with the right input signature on the fly.
Comparisons of training in python loop, avoiding the retracing –
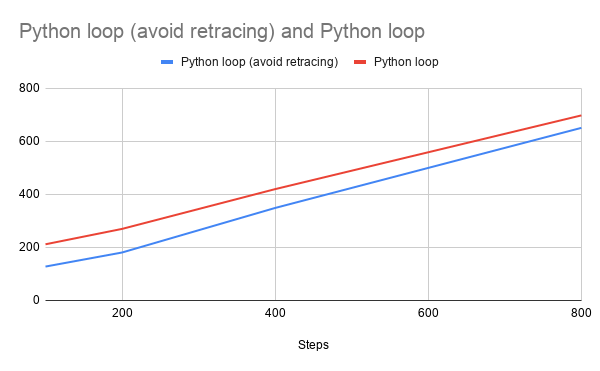
Follow Up
As a follow up to Tf.function retracing vs specifying an input signature , training on naval dataset is run for 5000 steps, with various combinations of annotating tf functions. Following are the results

Refer to attached notebook for details:
TF-function-timings Download
We can conclude that retracing is expensive operation, but once the function has retraced all possible shapes, the compiled function is faster.
Making train_step as the tf.function is much faster than making compute_gradient as the tf function.